Celebrity-aware Graph Contrastive Learning Framework for Social Recommendation
PROCEEDINGS OF THE 32ND ACM INTERNATIONAL CONFERENCE ON INFORMATION AND KNOWLEDGE MANAGEMENT, CIKM 2023(2023)
摘要
Social networks exhibit a distinct "celebrity effect" whereby influential individuals have a more significant impact on others compared to ordinary individuals, unlike other network structures such as citation networks and knowledge graphs. Despite its common occurrence in social networks, the celebrity effect is frequently overlooked by existing social recommendation methods when modeling social relationships, thereby hindering the full exploitation of social networks to mine similarities between users. In this paper, we fill this gap and propose a Celebrity-aware Graph Contrastive Learning Framework for Social Recommendation (CGCL), which explicitly models the celebrity effect in the social domain. Technically, we measure the different influences of celebrity and ordinary nodes by mining social network structure features, such as closeness centrality. To model the celebrity effect in social networks, we design a novel user-user impact-aware aggregation method, which incorporates the celebrity-aware influence information into the message propagation process. Additionally, we design a graph neural network-based framework which incorporates social semantics into the user-item interaction modeling with contrastive learning-enhanced data augmentation. The experimental results on three real-world datasets show the effectiveness of the proposed framework. We conduct ablation experiments to prove that the key components of our model benefit the recommendation performance improvement.
更多查看译文
关键词
Social Recommendation,Celebrity Effect,Graph Neural Network
AI 理解论文
溯源树
样例
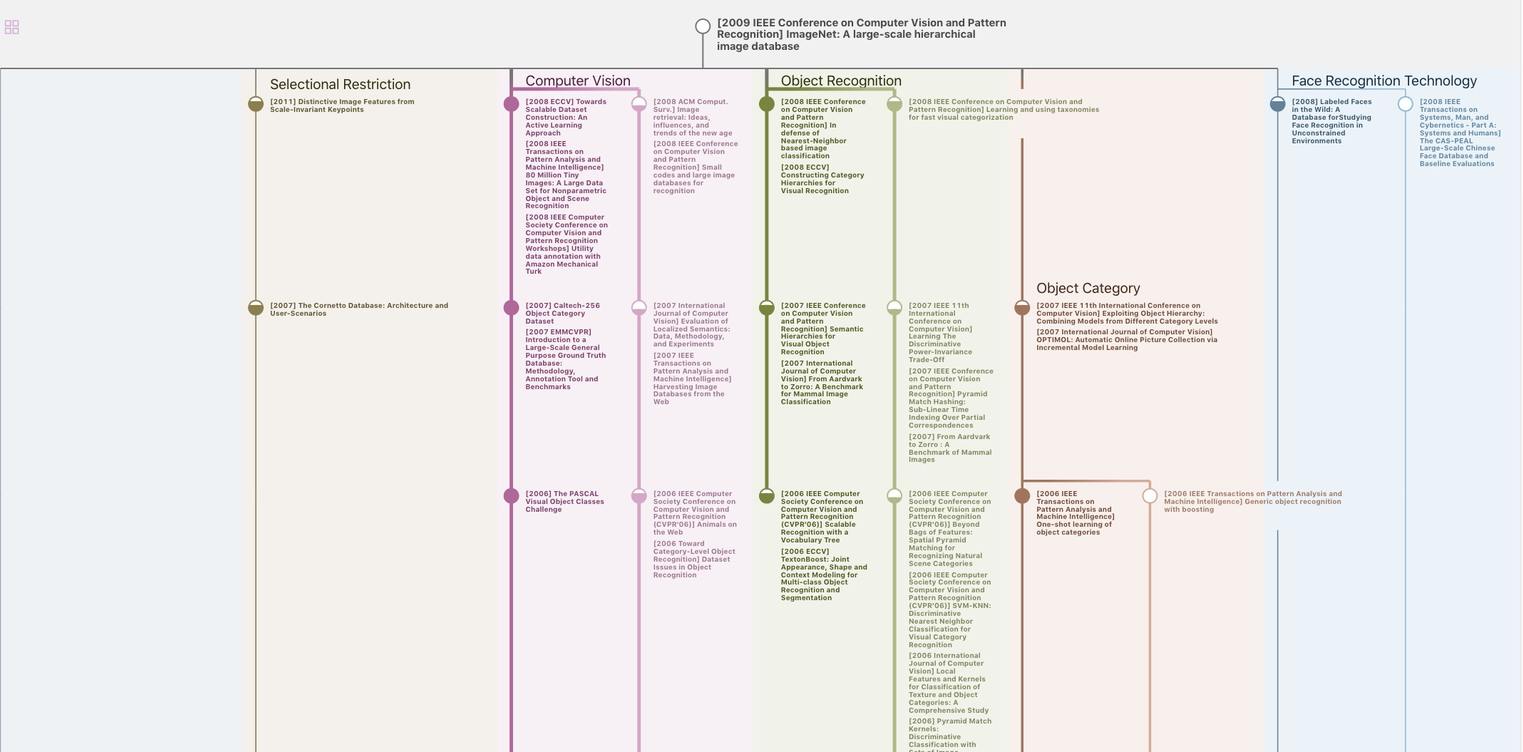
生成溯源树,研究论文发展脉络
Chat Paper
正在生成论文摘要