Search-Efficient Computerized Adaptive Testing
PROCEEDINGS OF THE 32ND ACM INTERNATIONAL CONFERENCE ON INFORMATION AND KNOWLEDGE MANAGEMENT, CIKM 2023(2023)
摘要
Computerized Adaptive Testing (CAT) arises as a promising personalized test mode in online education, targeting at revealing students' latent knowledge state by selecting test items adaptively. The item selection strategy is the core component of CAT, which searches for the best suitable test item based on students' current estimated ability at each test step. However, existing selection strategies behave in a brute-force manner, which results in the time complexity being linear to the number of items (N) in the item pool, i.e., O (N). Thus, in reality, the search latency becomes the bottleneck for CAT with a large-scale item pool. To this end, we propose a Search-Efficient Computerized Adaptive Testing framework (SECAT), which aims at enhancing CAT with an efficient selection strategy. Specifically, SECAT contains two main phases: item pool indexing and item search. In the item pool indexing phase, we apply a student-aware spatial partition method on the item pool to divide the test items into many sub-spaces, considering the adaptability of test items. In the item search phase, we optimize the traditional single-round search strategy with the asymptotic theory and propose a multi-round search strategy that can further improve the time efficiency. Compared with existing strategies, the time complexity of SECAT decreases from O(N) to O (logN). Across two real-world datasets, SECAT achieves over 200x speed up with negligible accuracy degradation.
更多查看译文
关键词
Computerized Adaptive Testing,Educational Resource Search,Educational Measurement,Cognitive Diagnosis
AI 理解论文
溯源树
样例
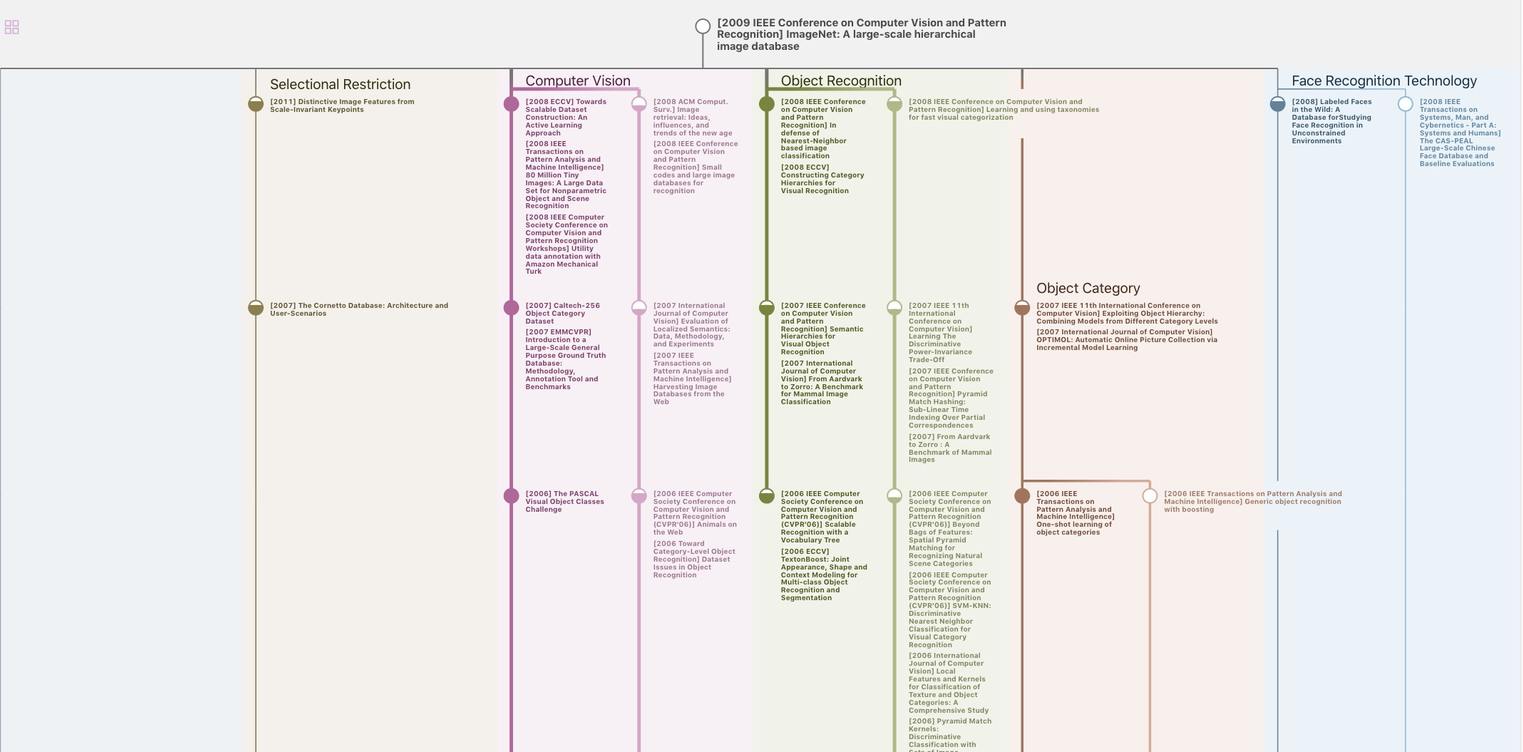
生成溯源树,研究论文发展脉络
Chat Paper
正在生成论文摘要