Liberate Pseudo Labels from Over-Dependence: Label Information Migration on Sparsely Labeled Graphs
PROCEEDINGS OF THE 32ND ACM INTERNATIONAL CONFERENCE ON INFORMATION AND KNOWLEDGE MANAGEMENT, CIKM 2023(2023)
摘要
Graph Convolutional Networks (GCNs) have made outstanding achievements in many tasks on graphs in recent years, but their success relies on sufficient training data. In practice, sparsely labeled graphs widely exist in the real world so self-training methods have become popular approaches by adding pseudo labeled nodes to enhance the performance of GCNs. However, we observe that most selected high-confidence pseudo labeled nodes by the existing methods would surround the true labeled nodes. It is what we called pseudo label over-dependence, which could lead to the non-uniform pseudo label distribution. Furthermore, a thorough experiment shows that the classification accuracy changes significantly under different label densities and the label-sparse regions show great potential improvement in the model performance. Based on the above findings, we theoretically analyze the constraint factors in the label-sparse regions and further propose reducing the feature distribution difference between the label-dense regions and label-sparse regions can effectively decrease the classification error. Thus, in this paper, we propose a novel Graph Label Information Migration framework (GLIM) to liberate pseudo labels from over-dependence. Specifically, we first propose a training dynamics module (TDM) that uses abundant training process information to find more reliable node labels and improve the model robustness against label noise. Then we propose a label migration module (LMM) that migrates label information from label-dense regions to label-sparse regions by a spectral-based graph matching algorithm. These migrated labels are like the glimmers in the darkness, providing the supervision signals for the unlabeled nodes in label-sparse regions. Finally, we conduct extensive experiments to demonstrate the effectiveness of the proposed GLIM.
更多查看译文
关键词
Graph Neural Networks,Self-Training,Pseudo Label
AI 理解论文
溯源树
样例
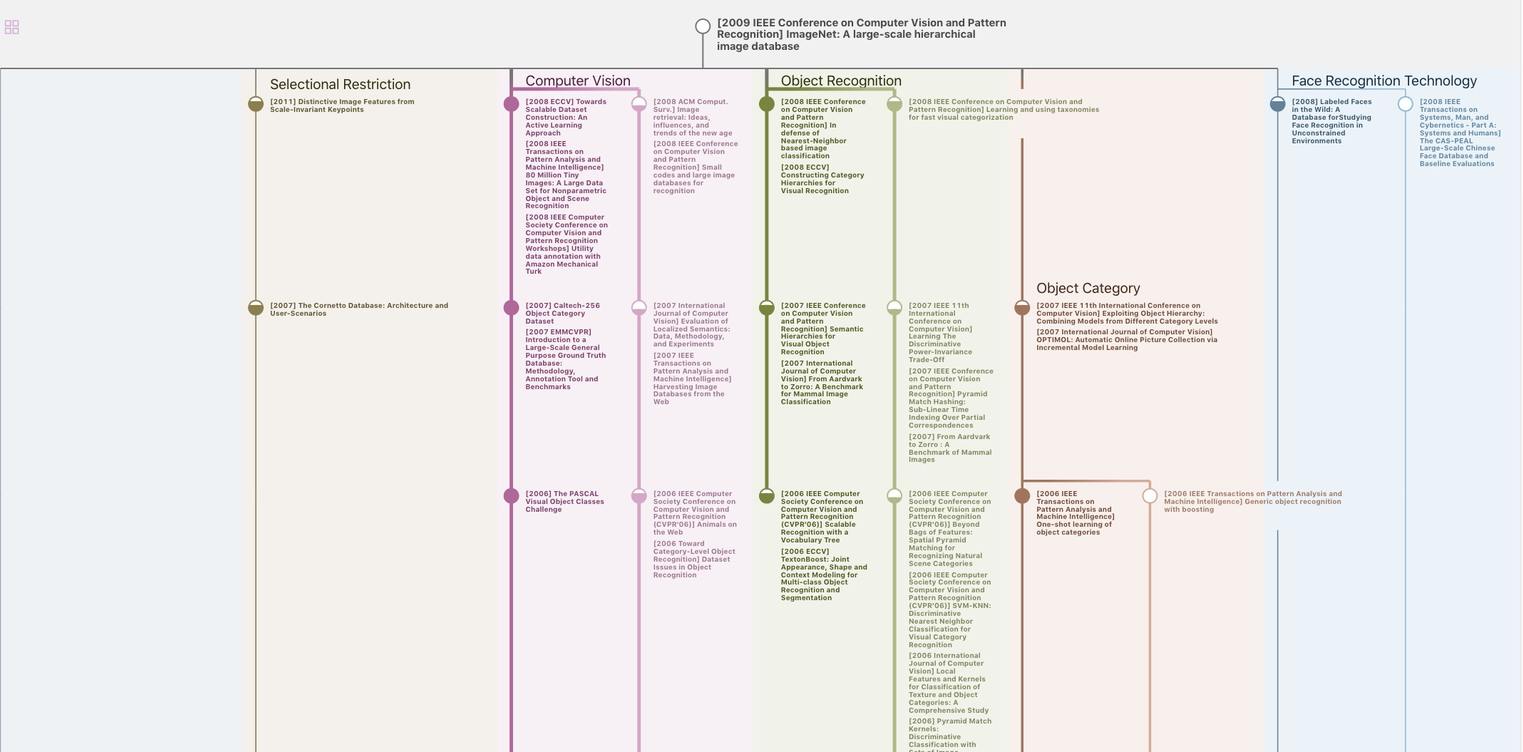
生成溯源树,研究论文发展脉络
Chat Paper
正在生成论文摘要