PriSHAP: Prior-guided Shapley Value Explanations for Correlated Features
PROCEEDINGS OF THE 32ND ACM INTERNATIONAL CONFERENCE ON INFORMATION AND KNOWLEDGE MANAGEMENT, CIKM 2023(2023)
摘要
Among numerous explainable AI (XAI) methods proposed in recent years, model explanations based on Shapley values are widely accepted for their solid theoretical support from game theory. However, most existing methods approximate Shapley values based on a feature independence assumption considering the complexity of calculating exact Shapley values. This assumption could bring some counterfactual problems when interpreted features are highly correlated and result in explanations contrary to human intuition. In this paper, we propose PriSHAP to explicitly model the dependency relationship between correlated features and provide reasonable explanations for tabular data. Feature dependencies are analyzed and taken as prior information to guide the process of estimating Shapley values. Additionally, PriSHAP is free to be applied in popular Shapley value-based explainers to address counterfactual problems while providing more faithful explanations. A pipeline is given to apply PriSHAP in existing explainers with simple adjustments. Extensive experiments on both public datasets and artificial datasets are provided to demonstrate the effectiveness of our method.
更多查看译文
关键词
Interpretability,XAI,Shapley value,feature dependency,SHAP
AI 理解论文
溯源树
样例
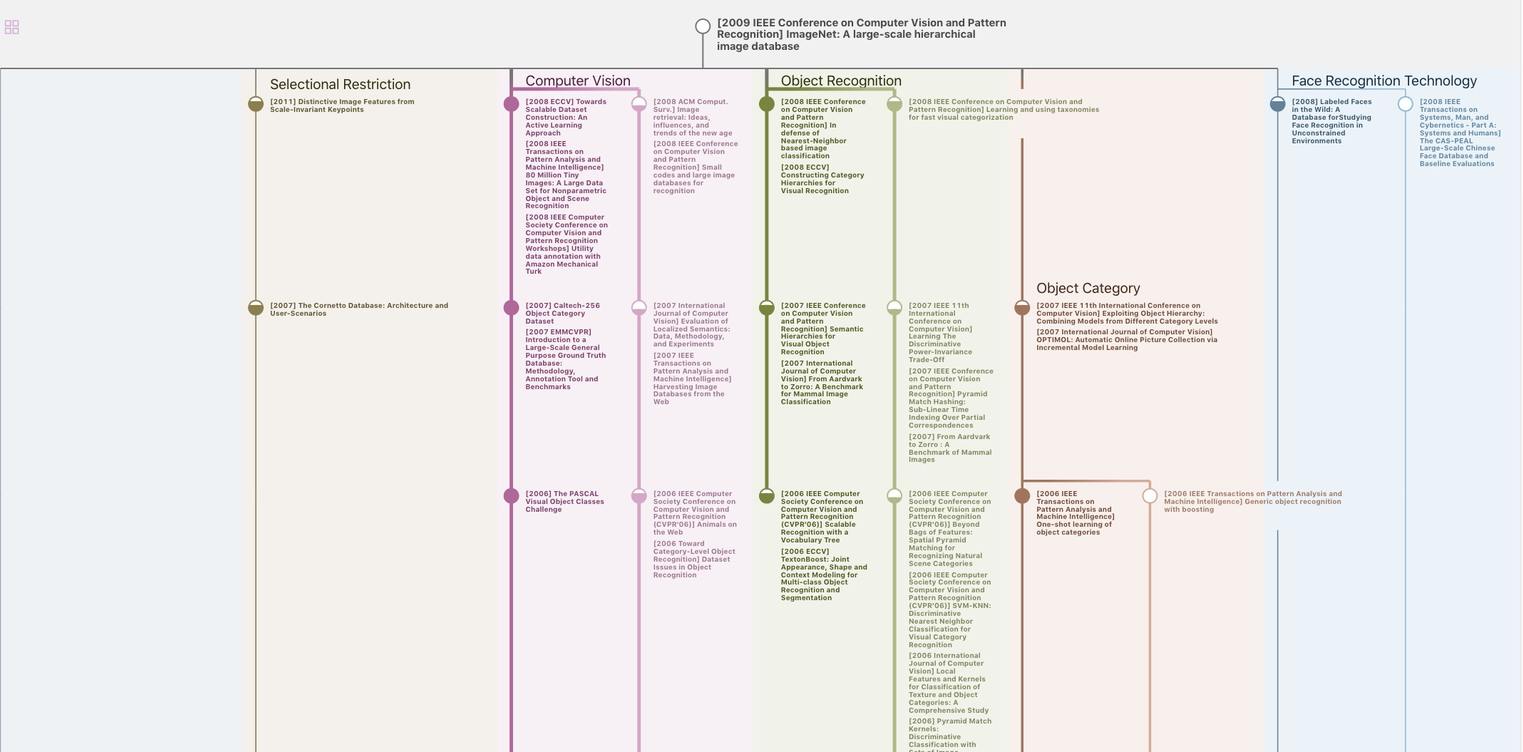
生成溯源树,研究论文发展脉络
Chat Paper
正在生成论文摘要