A Mix-up Strategy to Enhance Adversarial Training with Imbalanced Data
PROCEEDINGS OF THE 32ND ACM INTERNATIONAL CONFERENCE ON INFORMATION AND KNOWLEDGE MANAGEMENT, CIKM 2023(2023)
摘要
Adversarial training has been proven to be one of the most effective techniques to defend against adversarial examples. The majority of existing adversarial training methods assume that every class in the training data is equally distributed. However, in reality, some classes often have a large number of training data while others only have a very limited amount. Recent studies have shown that the performance of adversarial training will degrade drastically if the training data is imbalanced. In this paper, we propose a simple yet effective framework to enhance the robustness of DNN models under imbalanced scenarios. Our framework, Imb-Mix, first augments the training dataset by generating multiple adversarial examples for samples in the minority classes. This is done by first adding random noise to the original adversarial examples created by one specific adversarial attack method. It then constructs Mixup-mimic mixed examples upon the augmented dataset used by adversarial training. In addition, we theoretically prove the regularization effect of our Mixup-mimic mixed examples generation technique in Imb-Mix. Extensive experiments on various imbalanced datasets verify the effectiveness of the proposed framework.
更多查看译文
关键词
model robustness,data augmentation,adversarial training
AI 理解论文
溯源树
样例
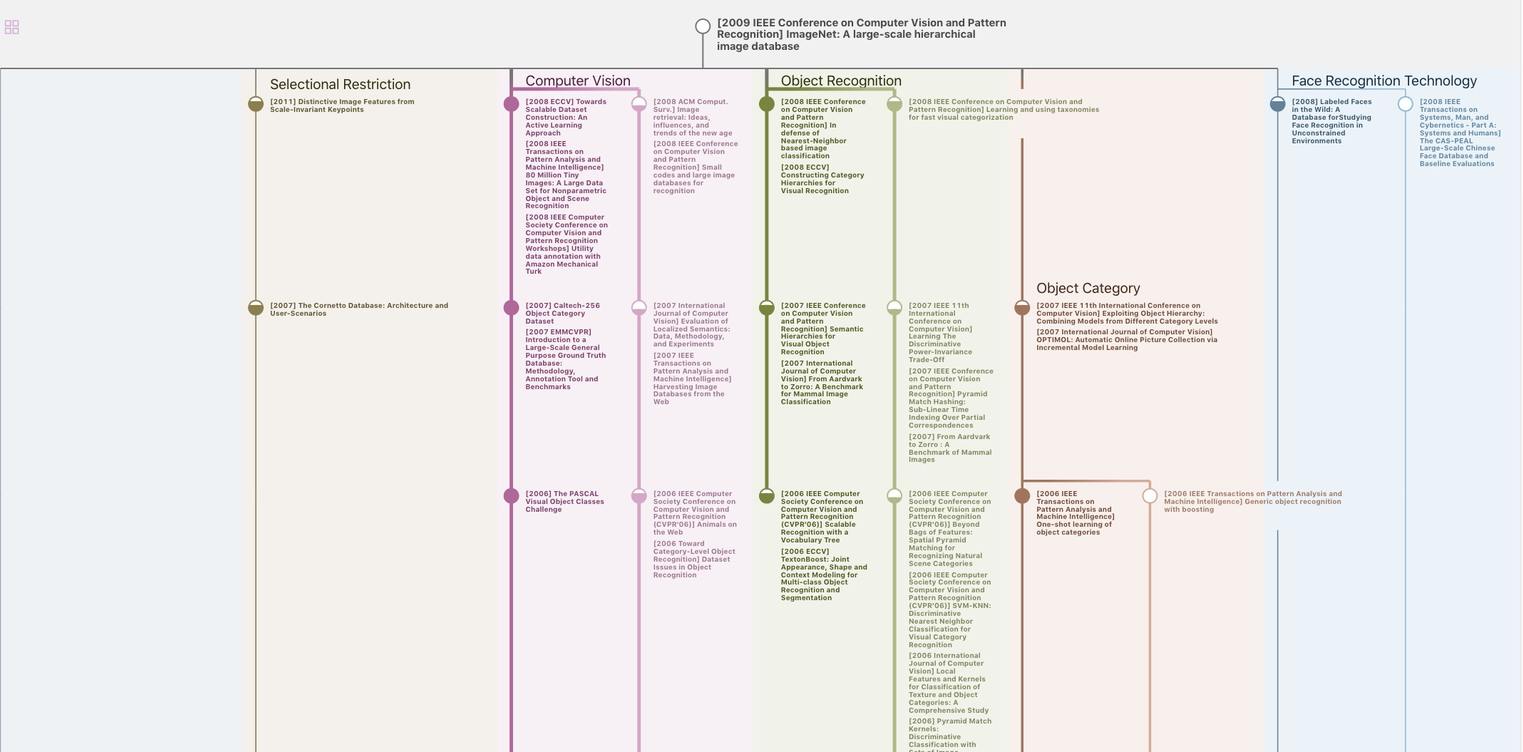
生成溯源树,研究论文发展脉络
Chat Paper
正在生成论文摘要