MultiPLe: Multilingual Prompt Learning for Relieving Semantic Confusions in Few-shot Event Detection
PROCEEDINGS OF THE 32ND ACM INTERNATIONAL CONFERENCE ON INFORMATION AND KNOWLEDGE MANAGEMENT, CIKM 2023(2023)
Abstract
Event detection (ED) is a challenging task in the field of information extraction. Due to the monolingual text and rampant confusing triggers, traditional ED models suffer from semantic confusions in terms of polysemy and synonym, leading to severe detection mistakes. Such semantic confusions can be further exacerbated in a practical situation where scarce labeled data cannot provide sufficient semantic clues. To mitigate such bottleneck, we propose a multilingual prompt learning (MultiPLe) framework for few-shot event detection (FSED), including three components, i.e., a multilingual prompt, a hierarchical prototype and a quadruplet contrastive learning module. In detail, to ease the polysemy confusion, the multilingual prompt module develops the in-context semantics of triggers via the multilingual disambiguation and prior knowledge in pretrained language models. Then, the hierarchical prototype module is adopted to diminish the synonym confusion by connecting the captured inmost semantics of fuzzy triggers with labels at a fine granularity. Finally, we employ the quadruplet contrastive learning module to tackle the insufficient label representation and potential noise. Experiments on two public datasets show that MultiPLe outperforms the state-of-the-art baselines in weighted F1-score, presenting a maximum improvement of 13.63% for FSED.
MoreTranslated text
Key words
Few-shot event detection,prompt learning,semantic confusions
AI Read Science
Must-Reading Tree
Example
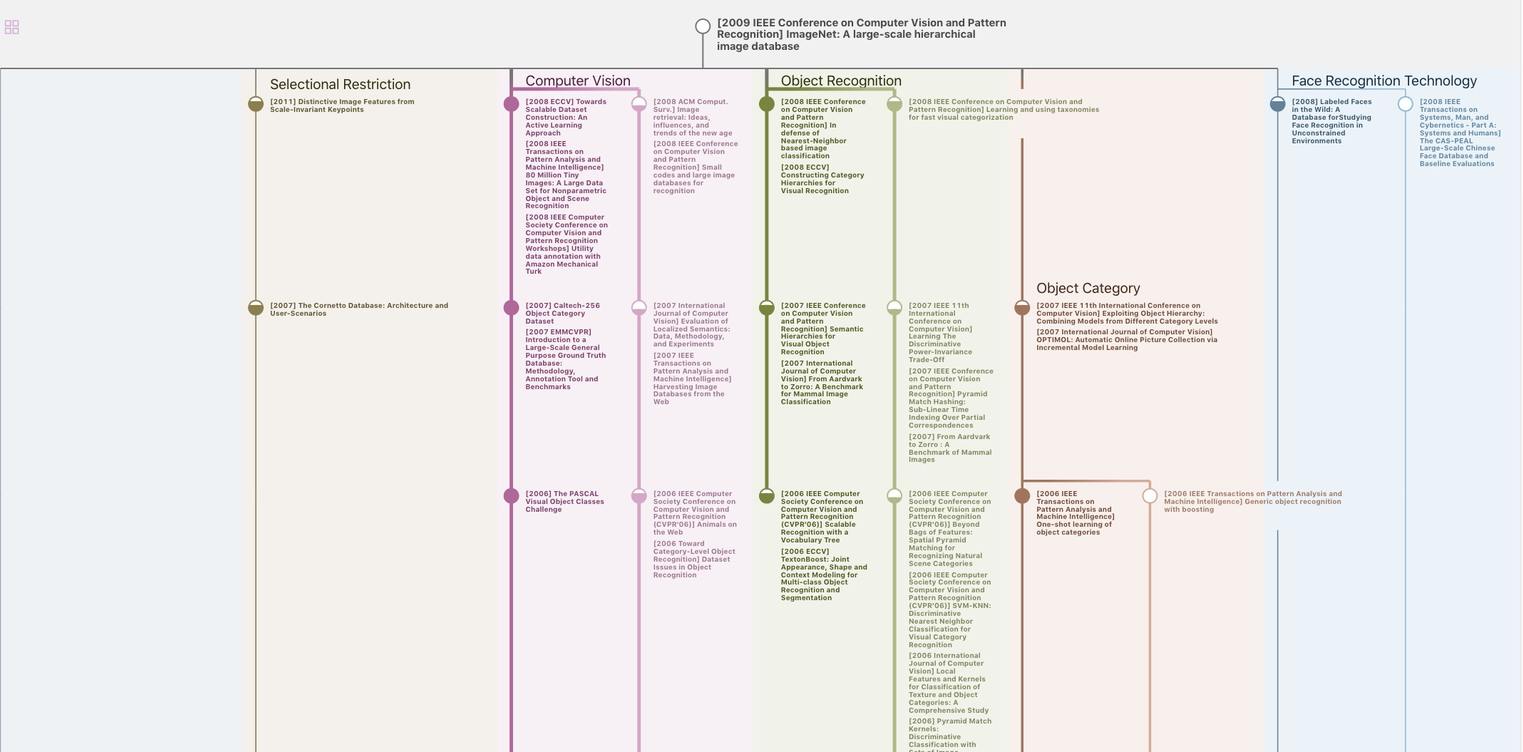
Generate MRT to find the research sequence of this paper
Chat Paper
Summary is being generated by the instructions you defined