Time-series Shapelets with Learnable Lengths
PROCEEDINGS OF THE 32ND ACM INTERNATIONAL CONFERENCE ON INFORMATION AND KNOWLEDGE MANAGEMENT, CIKM 2023(2023)
摘要
Shapelets are subsequences that are effective for classifying time-series instances. Learning shapelets by a continuous optimization has recently been studied to improve computational efficiency and classification performance. However, existing methods have employed predefined and fixed shapelet lengths during the continuous optimization, despite the fact that shapelets and their lengths are inherently interdependent and thus should be jointly optimized. To efficiently explore shapelets of high quality in terms of interpretability and inter-class separability, this study makes the shapelet lengths continuous and learnable. The proposed formulation jointly optimizes not only a binary classifier and shapelets but also shapelet lengths. The derived SGD optimization can be theoretically interpreted as improving the quality of shapelets in terms of shapelet closeness to the time series for target / off-target classes. We demonstrate improvements in area under the curve, total training time, and shapelet interpretability on UCR binary datasets.
更多查看译文
关键词
time series,shapelets,classification,interpretability
AI 理解论文
溯源树
样例
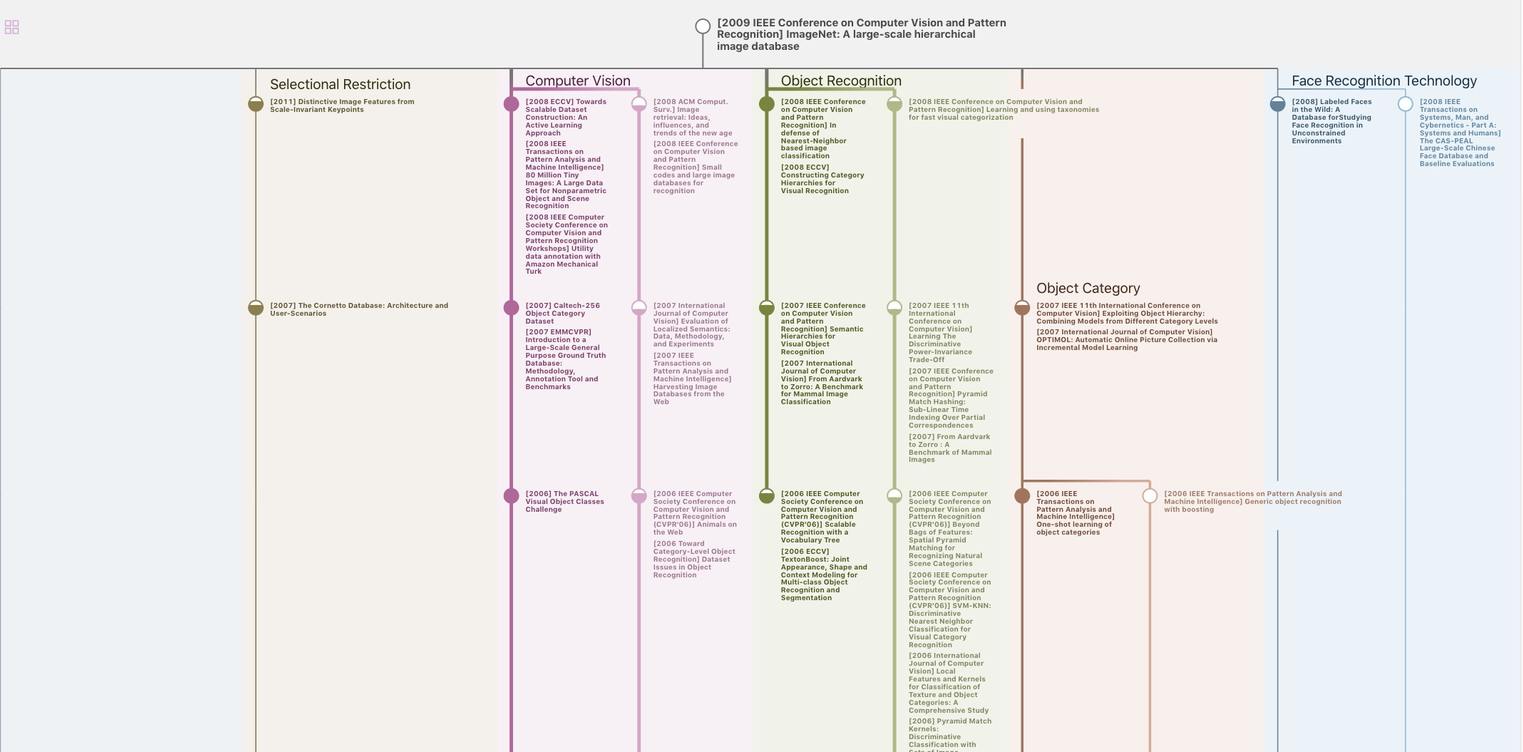
生成溯源树,研究论文发展脉络
Chat Paper
正在生成论文摘要