Mutual Information-Driven Multi-View Clustering
PROCEEDINGS OF THE 32ND ACM INTERNATIONAL CONFERENCE ON INFORMATION AND KNOWLEDGE MANAGEMENT, CIKM 2023(2023)
摘要
In deep multi-view clustering, three intractable problems are posed ahead of researchers, namely, the complementarity exploration problem, the information preservation problem, and the cluster structure discovery problem. In this paper, we consider the deep multi-view clustering from the perspective of mutual information (MI), and attempt to address the three important concerns with a Mutual Information-Driven Multi-View Clustering (MIMC) method, which extracts the common and view-specific information hidden in multi-view data and constructs a clustering-oriented comprehensive representation. Specifically, three constraints based on MI are devised in response to three issues. Correspondingly, we minimize the MI between the common representation and view-specific representations to exploit the inter-view complementary information. Further, we maximize the MI between the refined data representations and original data representations to preserve the principal information. Moreover, to learn a clustering-friendly comprehensive representation, the MI between the comprehensive embedding space and cluster structure is maximized. Finally, we conduct extensive experiments on six benchmark datasets, and the experimental results indicate that the proposed MIMC outperforms other clustering methods.
更多查看译文
关键词
Multi-view clustering,mutual information,contrastive learning
AI 理解论文
溯源树
样例
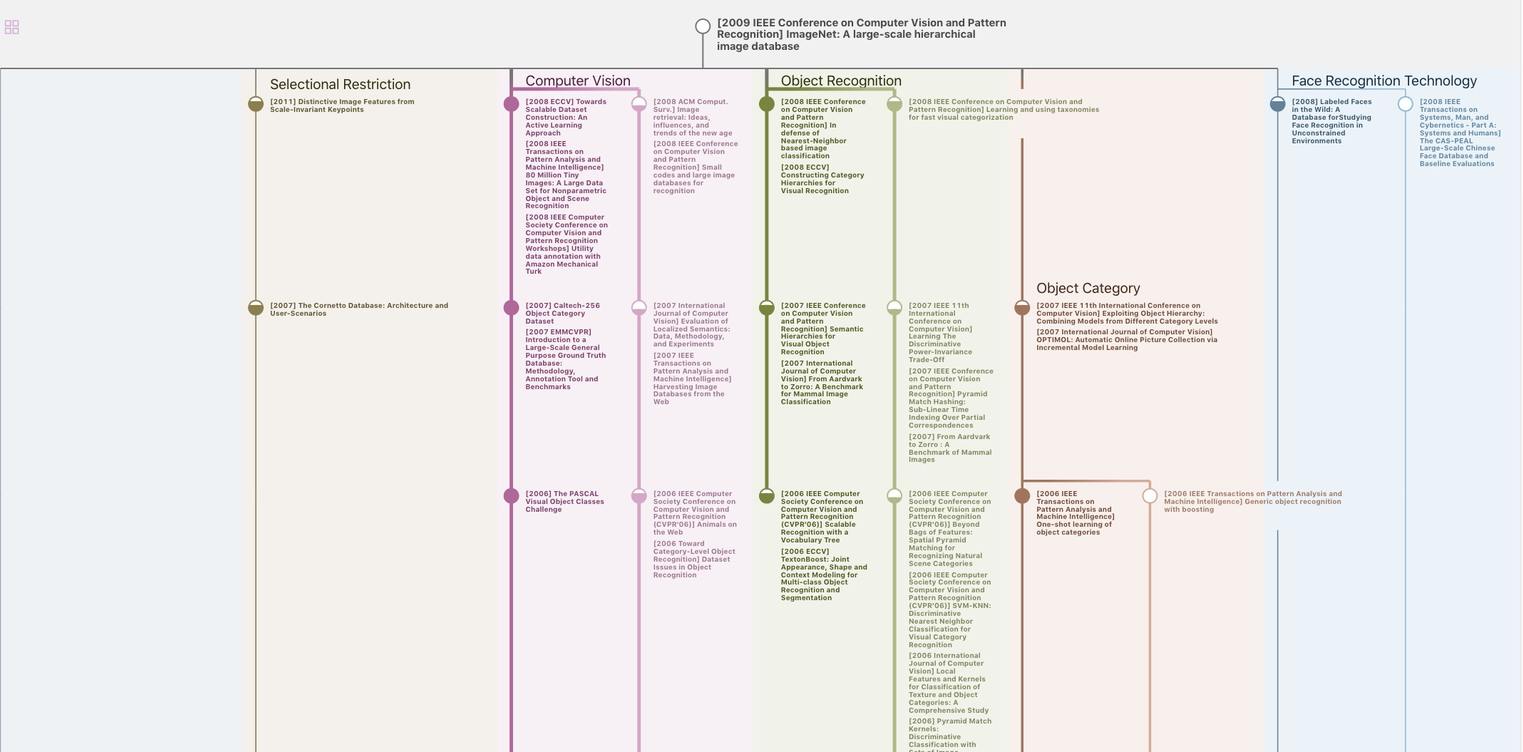
生成溯源树,研究论文发展脉络
Chat Paper
正在生成论文摘要