Partial Evaluation of Automatic Differentiation for Differential-Algebraic Equations Solvers
PROCEEDINGS OF THE 22ND ACM SIGPLAN INTERNATIONAL CONFERENCE ON GENERATIVE PROGRAMMING: CONCEPTS AND EXPERIENCES, GPCE 2023(2023)
Abstract
Differential-Algebraic Equations (DAEs) are the foundation of high-level equation-based languages for modeling physical dynamical systems. Simulating models in such languages requires a transformation known as index reduction that involves differentiating individual equations before numerical integration. Commercial and open-source implementations typically perform index reduction by symbolic differentiation (SD) and produce a Jacobian callback function with forward-mode automatic differentiation (AD). The former results in efficient runtime code, and the latter is asymptotically efficient in both runtime and code size. However, AD introduces runtime overhead caused by a non-standard representation of real numbers, and SD is not always applicable in models with general recursion. This work proposes a new approach that uses partial evaluation of AD in the context of numerical DAE solving to combine the strengths of the two differentiation methods while mitigating their weaknesses. Moreover, our approach selectively specializes partial derivatives of the Jacobian by exploiting structural knowledge while respecting a user-defined bound on the code size. Our evaluation shows that the new method both enables expressive modeling from AD and retains the efficiency of SD for many practical applications.
MoreTranslated text
Key words
Automatic Differentiation,Differential-Algebraic Equations,Partial Evaluation,Jacobian Generation,Compiler
AI Read Science
Must-Reading Tree
Example
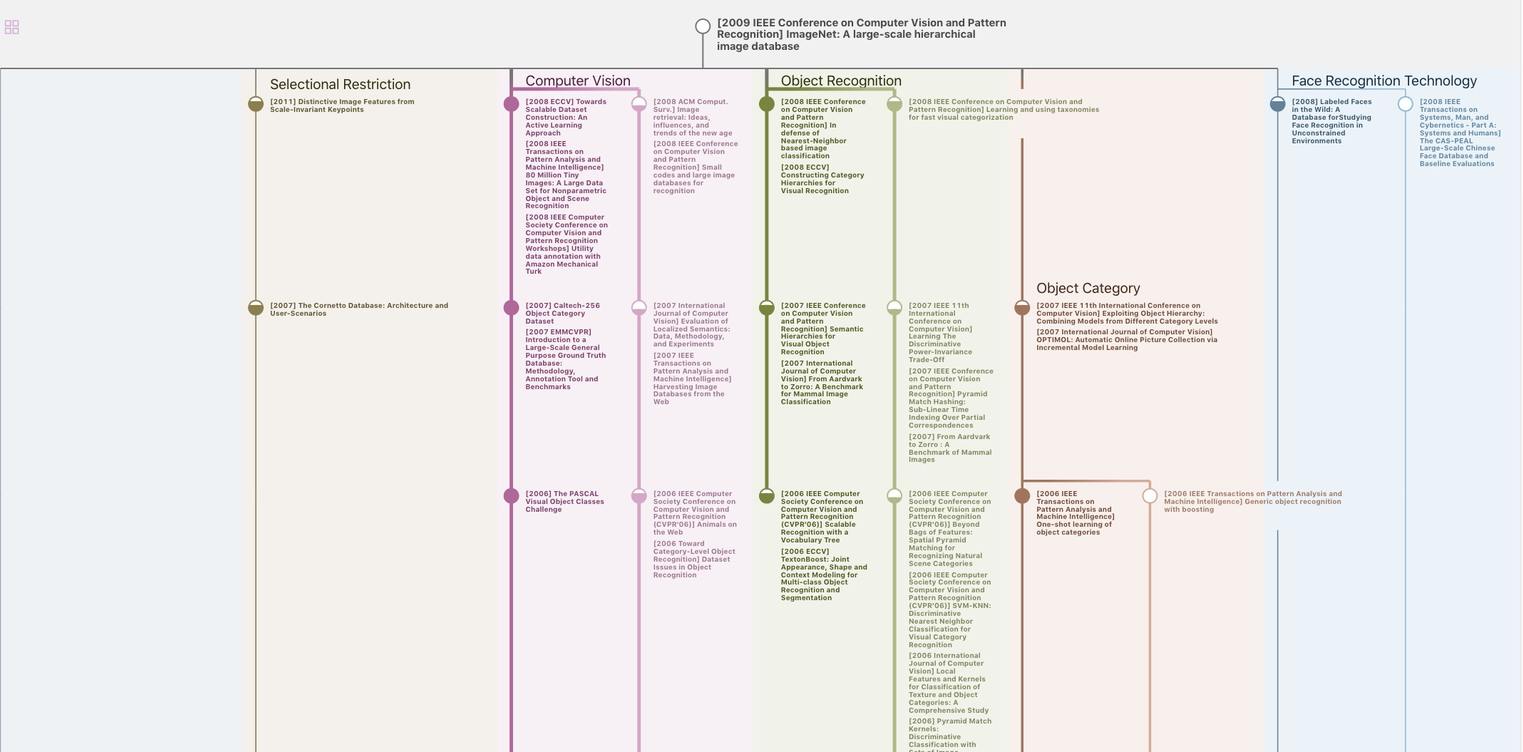
Generate MRT to find the research sequence of this paper
Chat Paper
Summary is being generated by the instructions you defined