Contrastive Counterfactual Learning for Causality-aware Interpretable Recommender Systems
PROCEEDINGS OF THE 32ND ACM INTERNATIONAL CONFERENCE ON INFORMATION AND KNOWLEDGE MANAGEMENT, CIKM 2023(2023)
摘要
The field of generating recommendations within the framework of causal inference has seen a recent surge. This approach enhances insights into the influence of recommendations on user behavior and helps in identifying the underlying factors. Existing research has often leveraged propensity scores to mitigate bias, albeit at the risk of introducing additional variance. Others have explored the use of unbiased data from randomized controlled trials, although this comes with assumptions that may prove challenging in practice. In this paper, we first present the causality-aware interpretation of recommendations and reveal how the underlying exposure mechanism can bias the maximum likelihood estimation (MLE) of observational feedback. Recognizing that confounders may be elusive, we propose a contrastive self-supervised learning to minimize exposure bias, employing inverse propensity scores and expanding the positive sample set. Building on this foundation, we present a novel contrastive counterfactual learning method (CCL) that incorporates three unique positive sampling strategies grounded in estimated exposure probability or random counterfactual samples. Through extensive experiments on two real-world datasets, we demonstrate that our CCL outperforms the state-of-the-art methods.
更多查看译文
关键词
Recommender systems,Causal inference,Contrastive counterfactual learning,Propensity scores
AI 理解论文
溯源树
样例
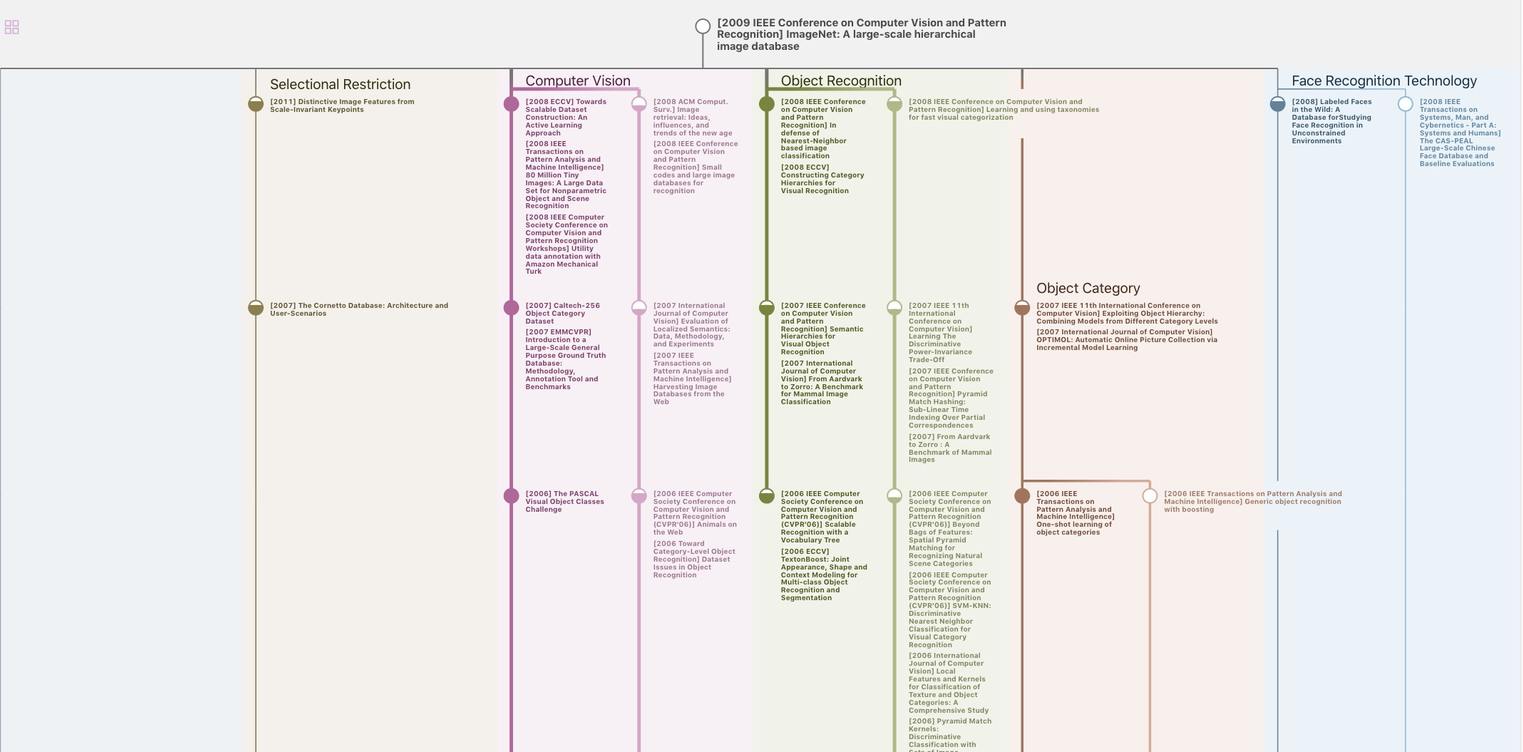
生成溯源树,研究论文发展脉络
Chat Paper
正在生成论文摘要