Optimizing for Member Value in an Edge Building Marketplace
PROCEEDINGS OF THE 32ND ACM INTERNATIONAL CONFERENCE ON INFORMATION AND KNOWLEDGE MANAGEMENT, CIKM 2023(2023)
Abstract
Social networks are prosperous marketplaces where creators and consumers congregate to share and consume various content. In general, products that rank content for distribution (such as news-feeds, stories, and notifications) and are related to edge recommendations (such as connect to members, follow celebrities or groups or hashtags) optimize the experience of active users. Typically, such users generate ample interaction data amenable to accurate model training and prediction. In contrast, we prioritize enhancing the experience of inactive members (IMs) who do not have a rich connection network. We formulate strategies for recommending superior edges to help members grow their connection network. Adapting the recommendations provides enormous value to the IMs and can significantly influence their future behaviour and engagement with the ecosystem. To that end, we propose a general and scalable multi-objective optimization (MOO) framework to provide more value to IMs as invitation recipients on LinkedIn, a professional network with over 900.. members. To deal with the enormous scale, we formulate the problem as a massive constrained linear optimization involving billions of variables and millions of constraints and efficiently solve it using accelerated gradient descent, making this the largest deployment of LP-based recommender systems worldwide. Furthermore, the proposed MOO paradigm can solve the general problem of matching different types of entities in an m-sided marketplace. Finally, we discuss the challenges and benefits of implementing and ramping our method in production at scale at LinkedIn and report our findings about the core business metrics related to users' engagement and network health.
MoreTranslated text
Key words
second-pass-ranker,multi-objective optimization,people recommendation,linear programming
AI Read Science
Must-Reading Tree
Example
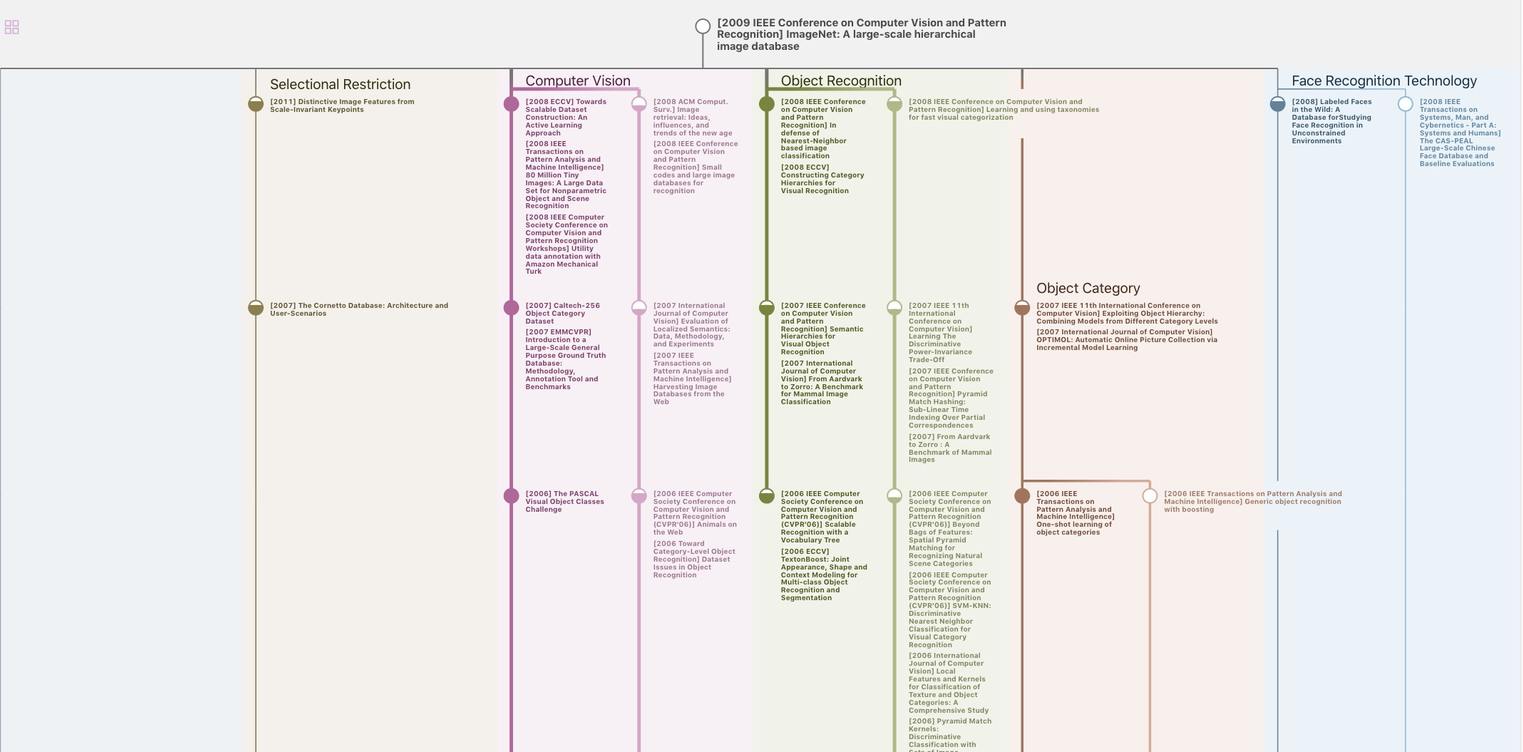
Generate MRT to find the research sequence of this paper
Chat Paper
Summary is being generated by the instructions you defined