Tree Search in DAG Space with Model-based Reinforcement Learning for Causal Discovery
CoRR(2023)
Abstract
Identifying causal structure is central to many fields ranging from strategic
decision-making to biology and economics. In this work, we propose CD-UCT, a
model-based reinforcement learning method for causal discovery based on tree
search that builds directed acyclic graphs incrementally. We also formalize and
prove the correctness of an efficient algorithm for excluding edges that would
introduce cycles, which enables deeper discrete search and sampling in DAG
space. The proposed method can be applied broadly to causal Bayesian networks
with both discrete and continuous random variables. We conduct a comprehensive
evaluation on synthetic and real-world datasets, showing that CD-UCT
substantially outperforms the state-of-the-art model-free reinforcement
learning technique and greedy search, constituting a promising advancement for
combinatorial methods.
MoreTranslated text
Key words
reinforcement learning,dag space,causal,discovery,tree,model-based
AI Read Science
Must-Reading Tree
Example
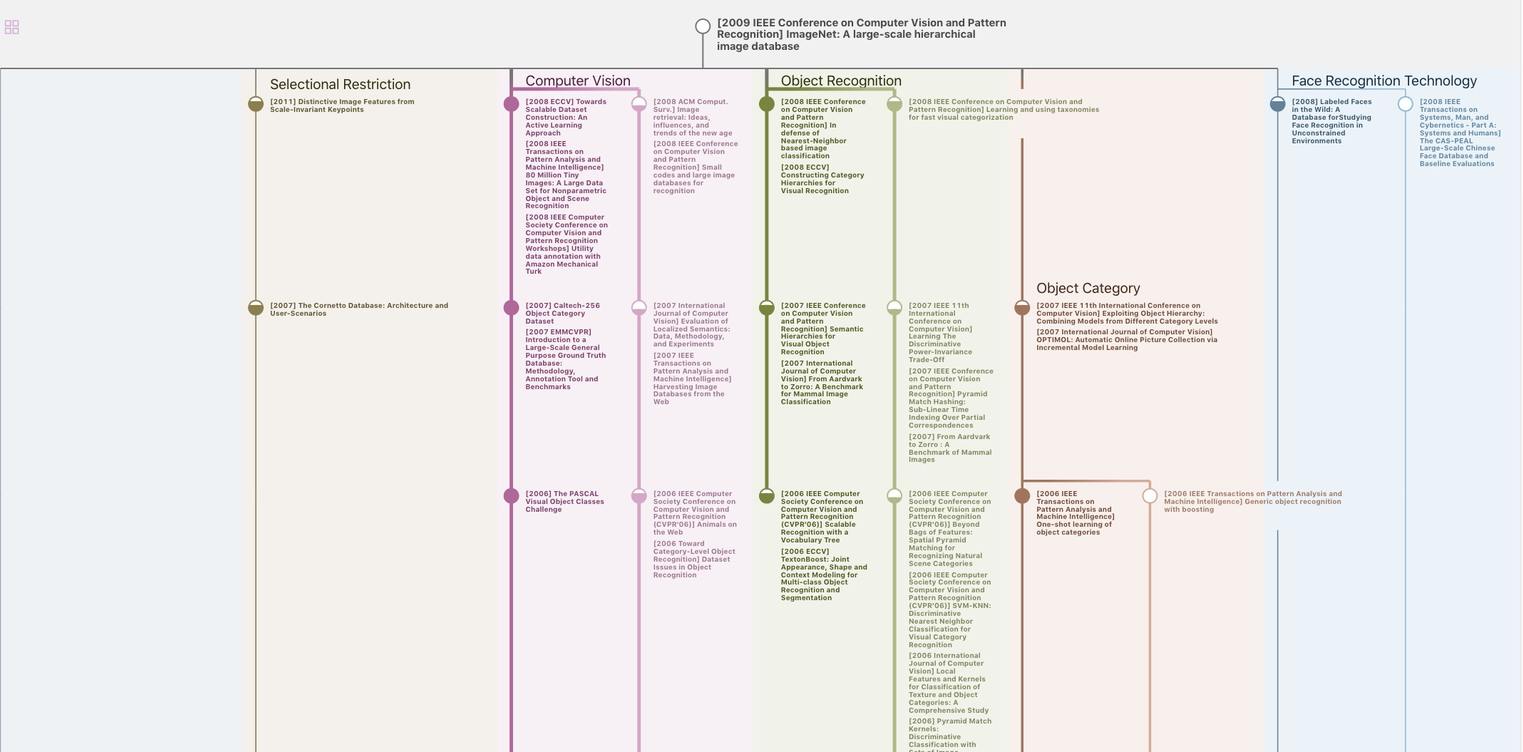
Generate MRT to find the research sequence of this paper
Chat Paper
Summary is being generated by the instructions you defined