SigFormer: Signature Transformers for Deep Hedging
PROCEEDINGS OF THE 4TH ACM INTERNATIONAL CONFERENCE ON AI IN FINANCE, ICAIF 2023(2023)
摘要
Deep hedging is a promising direction in quantitative finance, incorporating models and techniques from deep learning research. While giving excellent hedging strategies, models inherently requires careful treatment in designing architectures for neural networks. To mitigate such difficulties, we introduce SIGFORMER, a novel deep learning model that combines the power of path signatures and transformers to handle sequential data, particularly in cases with irregularities. Path signatures effectively capture complex data patterns, while transformers provide superior sequential attention. Our proposed model is empirically compared to existing methods on synthetic data, showcasing faster learning and enhanced robustness, especially in the presence of irregular underlying price data. Additionally, we validate our model performance through a real-world backtest on hedging the S&P 500 index, demonstrating positive outcomes.
更多查看译文
关键词
signature transformers,sigformer,deep
AI 理解论文
溯源树
样例
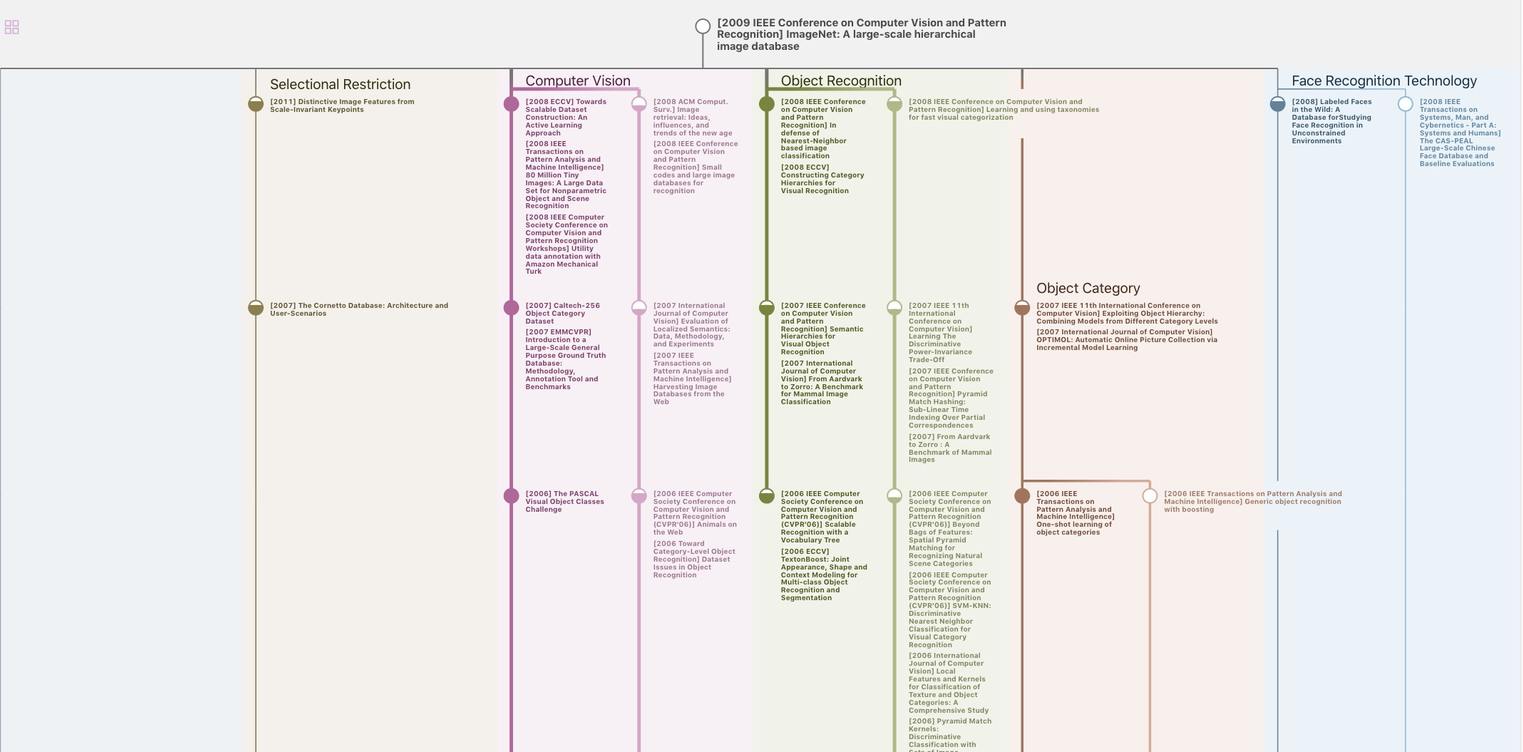
生成溯源树,研究论文发展脉络
Chat Paper
正在生成论文摘要