FLEE-GNN: A Federated Learning System for Edge-Enhanced Graph Neural Network in Analyzing Geospatial Resilience of Multicommodity Food Flows
PROCEEDINGS OF THE 6TH ACM SIGSPATIAL INTERNATIONAL WORKSHOP ON AI FOR GEOGRAPHIC KNOWLEDGE DISCOVERY, GEOAI 2023(2023)
摘要
Understanding and measuring the resilience of food supply networks is a global imperative to tackle increasing food insecurity. However, the complexity of these networks, with their multidimensional interactions and decisions, presents significant challenges. This paper proposes FLEE-GNN, a novel Federated Learning System for Edge-Enhanced Graph Neural Network, designed to overcome these challenges and enhance the analysis of geospatial resilience of multicommodity food flow network, which is one type of spatial networks. FLEE-GNN addresses the limitations of current methodologies, such as entropy-based methods, in terms of generalizability, scalability, and data privacy. It combines the robustness and adaptability of graph neural networks with the privacy-conscious and decentralized aspects of federated learning on food supply network resilience analysis across geographical regions. This paper also discusses FLEE-GNN's innovative data generation techniques, experimental designs, and future directions for improvement. The results show the advancements of this approach to quantifying the resilience of multicommodity food flow networks, contributing to efforts towards ensuring global food security using AI methods. The developed FLEE-GNN has the potential to be applied in other spatial networks with spatially heterogeneous sub-network distributions.
更多查看译文
关键词
federated learning,smart foodsheds,food supply networks,GeoAI
AI 理解论文
溯源树
样例
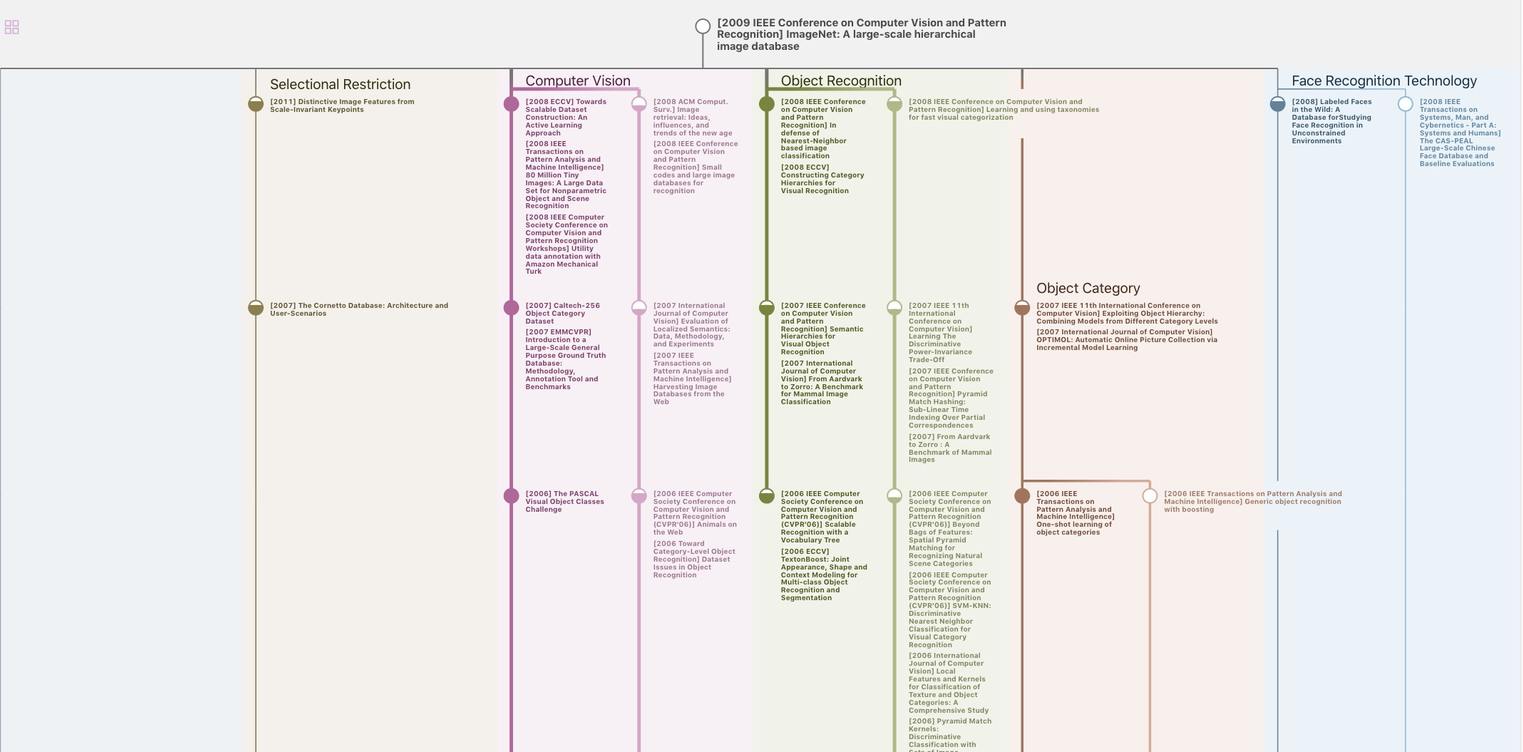
生成溯源树,研究论文发展脉络
Chat Paper
正在生成论文摘要