Unsupervised Candidate Answer Extraction through Differentiable Masker-Reconstructor Model.
CoRR(2023)
摘要
Question generation is a widely used data augmentation approach with extensive applications, and extracting qualified candidate answers from context passages is a critical step for most question generation systems. However, existing methods for candidate answer extraction are reliant on linguistic rules or annotated data that face the partial annotation issue and challenges in generalization. To overcome these limitations, we propose a novel unsupervised candidate answer extraction approach that leverages the inherent structure of context passages through a Differentiable Masker-Reconstructor (DMR) Model with the enforcement of self-consistency for picking up salient information tokens. We curated two datasets with exhaustively-annotated answers and benchmark a comprehensive set of supervised and unsupervised candidate answer extraction methods. We demonstrate the effectiveness of the DMR model by showing its performance is superior among unsupervised methods and comparable to supervised methods.
更多查看译文
关键词
extraction,masker-reconstructor
AI 理解论文
溯源树
样例
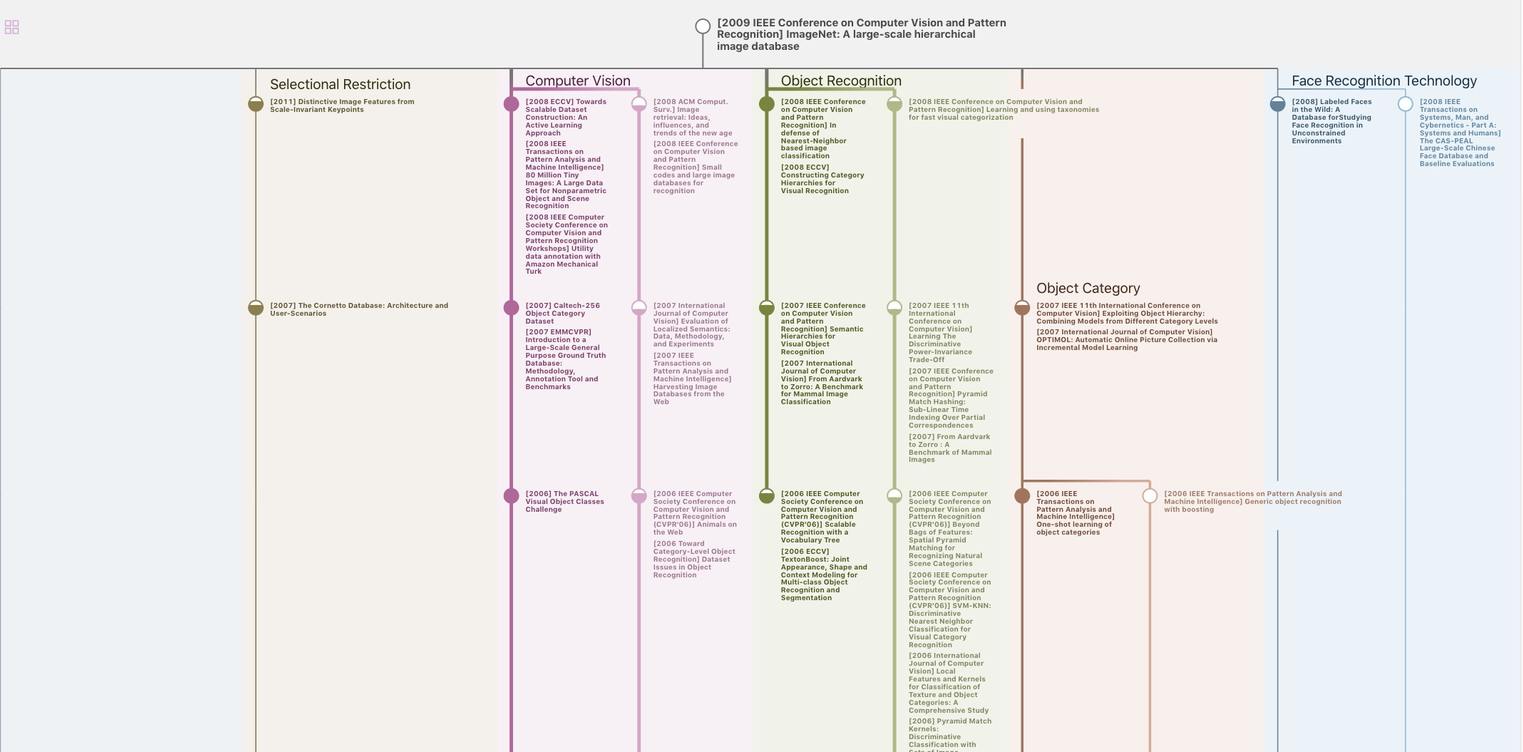
生成溯源树,研究论文发展脉络
Chat Paper
正在生成论文摘要