Closed-Loop Motion Planning for Differentially Flat Systems: A Time-Varying Optimization Framework
CoRR(2023)
Abstract
Motion planning and control are two core components of the robotic systems autonomy stack. The standard approach to combine these methodologies comprises an offline/open-loop stage, planning, that designs a feasible and safe trajectory to follow, and an online/closed-loop stage, tracking, that corrects for unmodeled dynamics and disturbances. Such an approach generally introduces conservativeness into the planning stage, which becomes difficult to overcome as the model complexity increases and real-time decisions need to be made in a changing environment. This work addresses these challenges for the class of differentially flat nonlinear systems by integrating planning and control into a cohesive closed-loop task. Precisely, we develop an optimization-based framework that aims to steer a differentially flat system to a trajectory implicitly defined via a constrained time-varying optimization problem. To that end, we generalize the notion of feedback linearization, which makes non-linear systems behave as linear systems, and develop controllers that effectively transform a differentially flat system into an optimization algorithm that seeks to find the optimal solution of a (possibly time-varying) optimization problem. Under sufficient regularity assumptions, we prove global asymptotic convergence for the optimization dynamics to the minimizer of the time-varying optimization problem. We illustrate the effectiveness of our method with two numerical examples: a multi-robot tracking problem and a robot obstacle avoidance problem.
MoreTranslated text
Key words
differentially flat systems,planning,optimization,closed-loop,time-varying
AI Read Science
Must-Reading Tree
Example
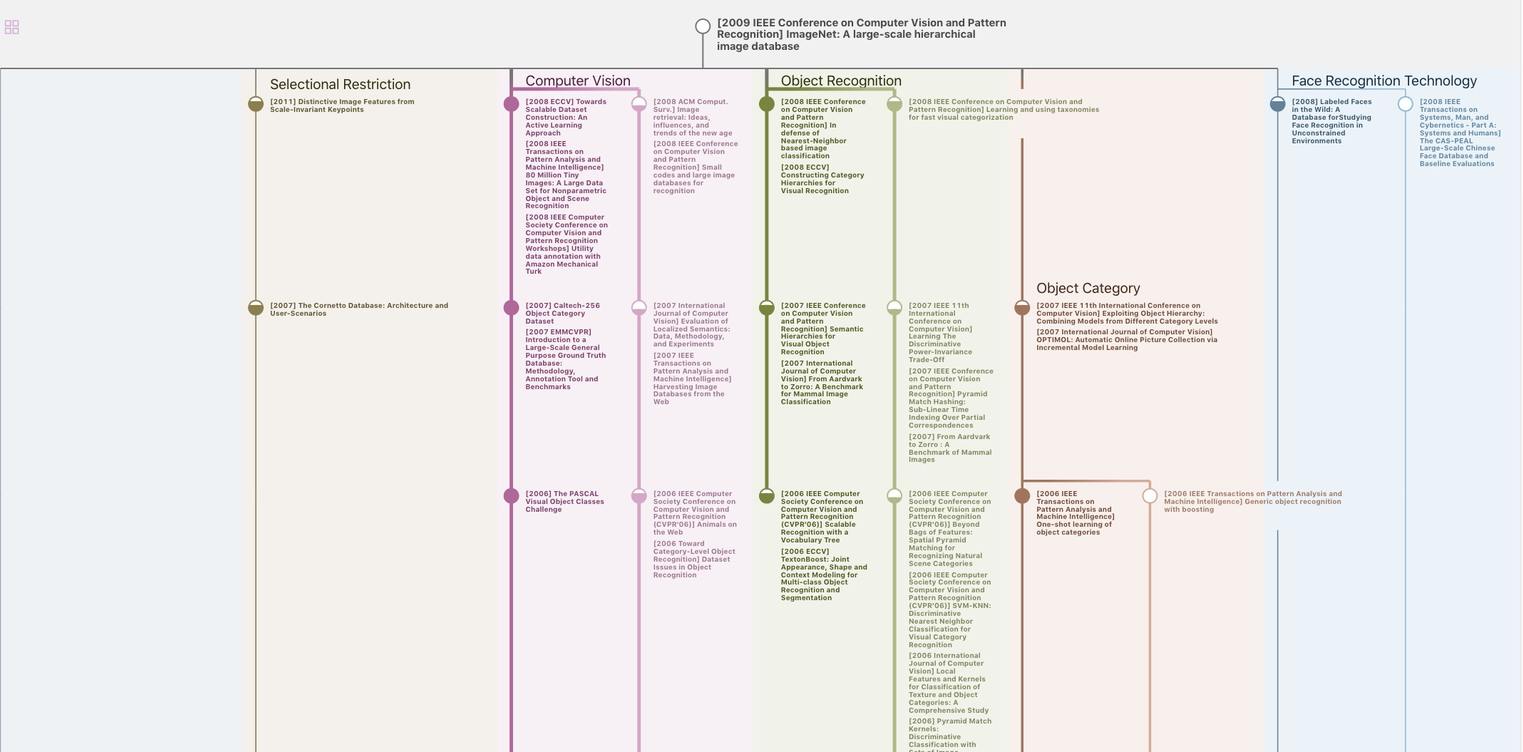
Generate MRT to find the research sequence of this paper
Chat Paper
Summary is being generated by the instructions you defined