Anomaly detection in the presence of irrelevant features
Journal of High Energy Physics(2024)
Abstract
bstract Experiments at particle colliders are the primary source of insight into physics at microscopic scales. Searches at these facilities often rely on optimization of analyses targeting specific models of new physics. Increasingly, however, data-driven model-agnostic approaches based on machine learning are also being explored. A major challenge is that such methods can be highly sensitive to the presence of many irrelevant features in the data. This paper presents Boosted Decision Tree (BDT)-based techniques to improve anomaly detection in the presence of many irrelevant features. First, a BDT classifier is shown to be more robust than neural networks for the Classification Without Labels approach to finding resonant excesses assuming independence of resonant and non-resonant observables. Next, a tree-based probability density estimator using copula transformations demonstrates significant stability and improved performance over normalizing flows as irrelevant features are added. The results make a compelling case for further development of tree-based algorithms for more robust resonant anomaly detection in high energy physics.
MoreTranslated text
Key words
Automation,Jets and Jet Substructure
AI Read Science
Must-Reading Tree
Example
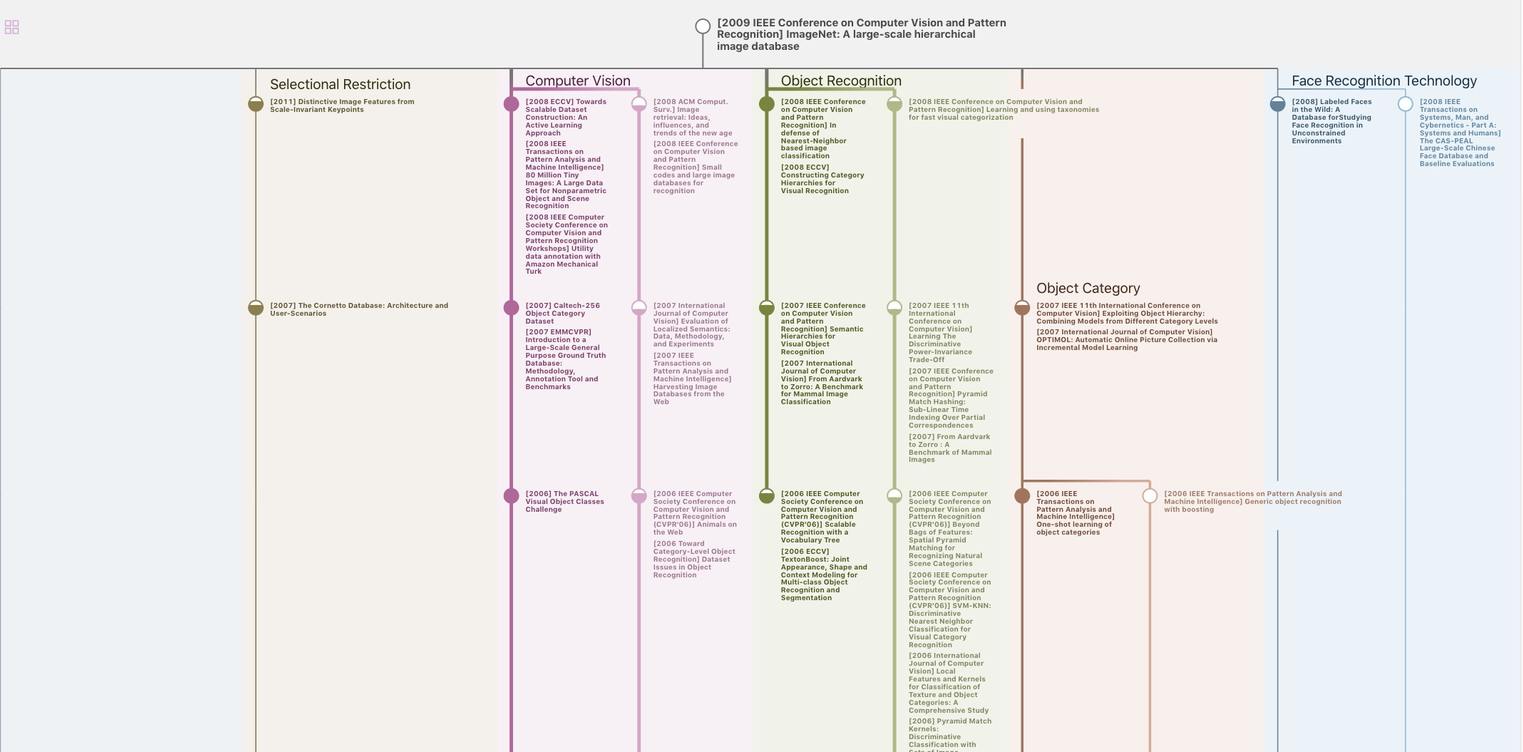
Generate MRT to find the research sequence of this paper
Chat Paper
Summary is being generated by the instructions you defined