Next waves in veridical network embedding*
Periodicals(2021)
Abstract
AbstractAbstractEmbedding nodes of a large network into a metric (e.g., Euclidean) space has become an area of active research in statistical machine learning, which has found applications in natural and social sciences. Generally, a representation of a network object is learned in a Euclidean geometry and is then used for subsequent tasks regarding the nodes and/or edges of the network, such as community detection, node classification and link prediction. Network embedding algorithms have been proposed in multiple disciplines, often with domain‐specific notations and details. In addition, different measures and tools have been adopted to evaluate and compare the methods proposed under different settings, often dependent of the downstream tasks. As a result, it is challenging to study these algorithms in the literature systematically. Motivated by the recently proposed PCS framework for Veridical Data Science, we propose a framework for network embedding algorithms and discuss how the principles of predictability, computability, and stability (PCS) apply in this context. The utilization of this framework in network embedding holds the potential to motivate and point to new directions for future research.
MoreTranslated text
Key words
feature engineering, latent variable models, network embedding, representational learning, veridical data science
AI Read Science
Must-Reading Tree
Example
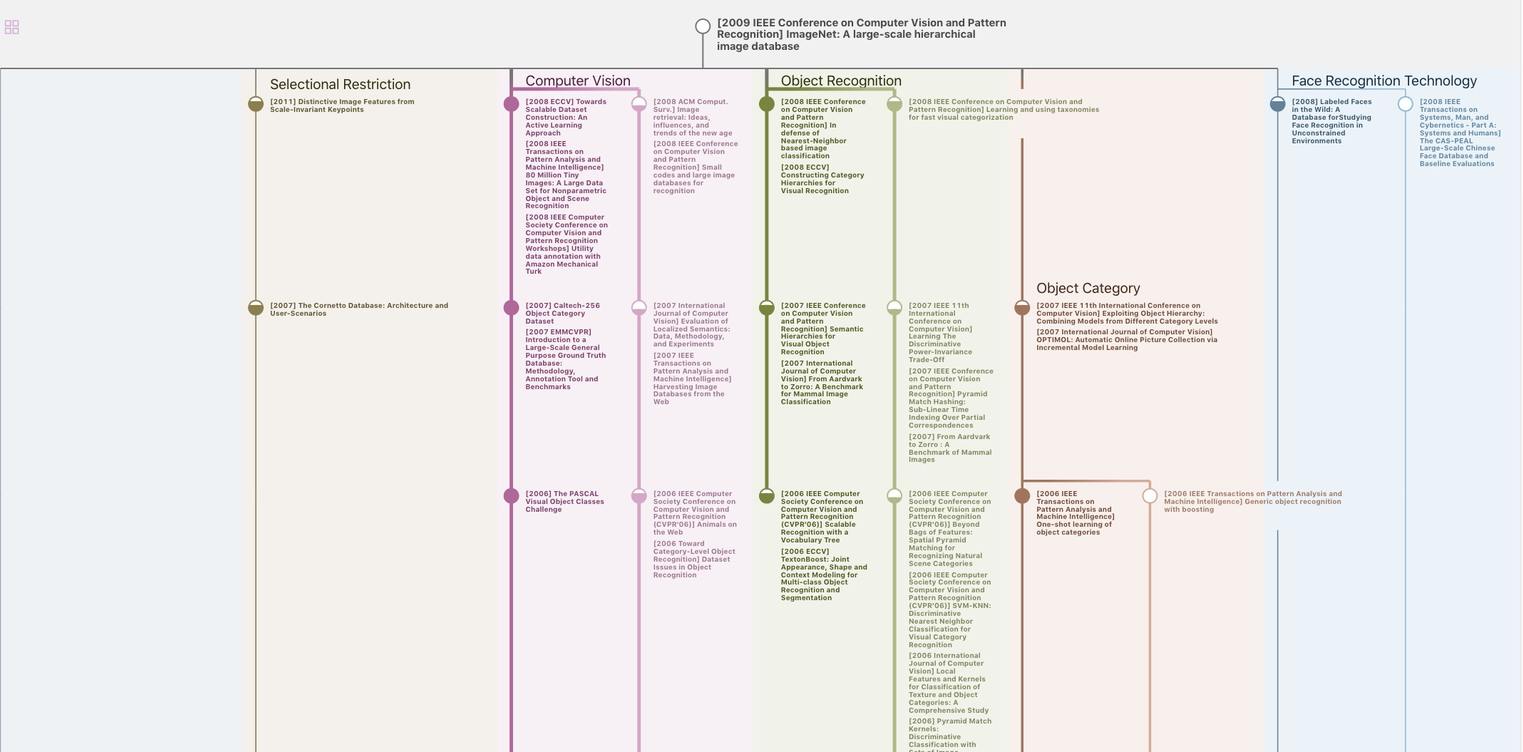
Generate MRT to find the research sequence of this paper
Chat Paper
Summary is being generated by the instructions you defined