Prediction of key milk biomarkers in dairy cows through milk MIR spectra and international collaborations
Journal of dairy science(2023)
摘要
At the individual cow level, sub-optimum fertility, mastitis, negative energy balance and ketosis are major issues in dairy farming. These problems are widespread on dairy farms and have an important economic impact. The objectives of this study were: 1) to assess the potential of milk Mid Infrared (MIR) spectra to predict key biomarkers of energy deficit (citrate, isocitrate, glucose-6P, free glucose), ketosis (BHB and acetone), mastitis (NAGase and LDH), and fertility (progesterone); 2) to test alternative methodologies to partial least square regression (PLS) to better account for the specific asymmetric distribution of the biomarkers; and 3) to create robust models by merging large data sets from 5 international or national projects. Benefiting from this international collaboration, the data set comprised a total of 9,143 milk samples from 3,758 cows located in 589 herds across 10 countries and represented 7 breeds. The samples were analyzed by reference chemistry for biomarker contents while the MIR analyses were performed on 30 instruments from different models and brands, with spectra harmonized into a common format. Four quantitative methodologies were evaluated to address the strongly skewed distribution of some biomarkers. PLS was used as the reference basis, and compared with a random modification of distribution associated with PLS (Random-downsampling-PLS), an optimized modification of distribution associated with PLS (KennardStone-downsampling-PLS) and Support Vector Machine (SVM). When the ability of MIR to predict biomarkers was too low for quantification, different qualitative methodologies were tested to discriminate low vs high values of biomarkers. For each biomarker, 20% of the herds were randomly removed within all countries to be used as the validation data set. The remaining 80% of herds were used as the calibration data set. In calibration, the 3 alternative methodologies outperform the PLS performances for the majority of biomarkers. However, in the external herd validation, PLS provided the best results for isocitrate, glucose-6P, free glucose and LDH (R2v = 0.48, 0.58, 0.28, and 0.24). For other molecules, PLS-Random-downsampling and PLS-KennardStone-downsampling outperformed PLS in the majority of cases, but the best results were provided by SVM for citrate, BHB, acetone, NAGase and progesterone (R2v = 0.94, 0.58, 0.76, 0.68, and 0.15). Hence, PLS and SVM based on the entire data set provided the best results for normal and skewed distributions, respectively. Complementary to the quantitative methods, the qualitative discriminant models enabled the discrimination of high and low values for BHB, acetone, and NAGase with a global accuracy around 90%, and glucose-6P with an accuracy of 83%. In conclusion, MIR spectra of milk can enable quantitative screening of citrate as a biomarker of energy deficit and discrimination of low and high values of BHB, acetone, and NAGase, as biomarkers of ketosis and mastitis. Finally, progesterone could not be predicted with sufficient accuracy from milk MIR spectra to be further considered. Consequently, MIR spectrometry can bring valuable information regarding the occurrence of energy deficit, ketosis and mastitis in dairy cows, which in turn have major influences on their fertility and survival.
更多查看译文
关键词
Fourier transform mid-infrared spectrometry,ketosis,negative energy balance,mastitis,fertility
AI 理解论文
溯源树
样例
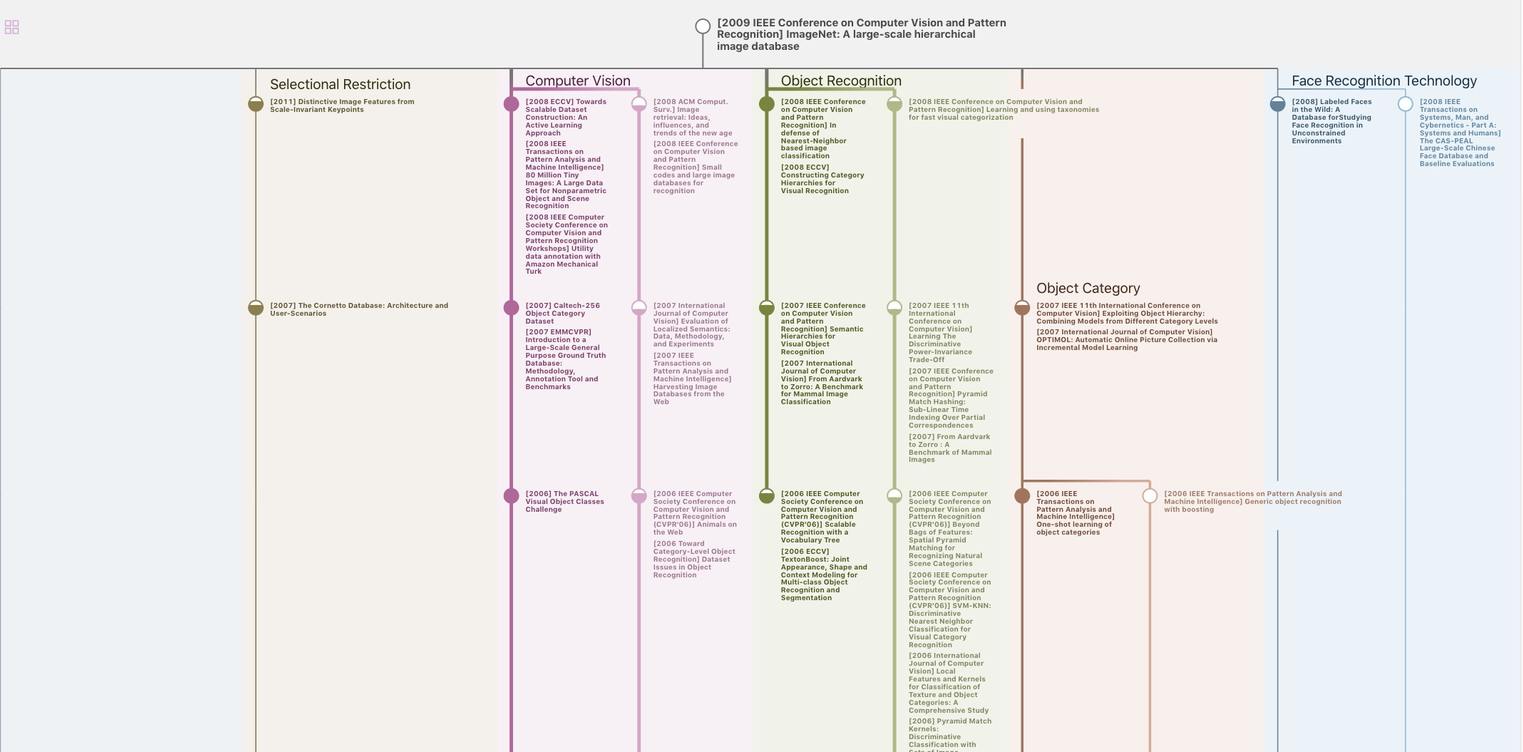
生成溯源树,研究论文发展脉络
Chat Paper
正在生成论文摘要