Defending Wireless Receivers Against Adversarial Attacks on Modulation Classifiers
IEEE INTERNET OF THINGS JOURNAL(2023)
摘要
Deep learning has been adopted for a wide range of wireless communication tasks, including modulation classification, because of its great classification capability. However, deep learning models have been shown to also introduce risks and vulnerabilities. For instance, adversarial attacks craft and introduce imperceptible perturbations that compromise the accuracy of deep learning-based modulation classifiers on wireless receivers. Therefore, in this article, we propose a novel wireless receiver architecture that enhances deep learning-based modulation classifiers to defend them against adversarial attacks. Our experimental results show that our defense technique significantly diminishes the accuracy reduction that is caused by adversarial attacks by protecting modulation classifiers at least 18% more than existing defense techniques.
更多查看译文
关键词
Perturbation methods,Modulation,Wireless communication,Training,Receivers,Communication system security,Resistance,Adversarial attacks,deep learning,machine learning,modulation classification,secure communications,security and privacy,wireless security
AI 理解论文
溯源树
样例
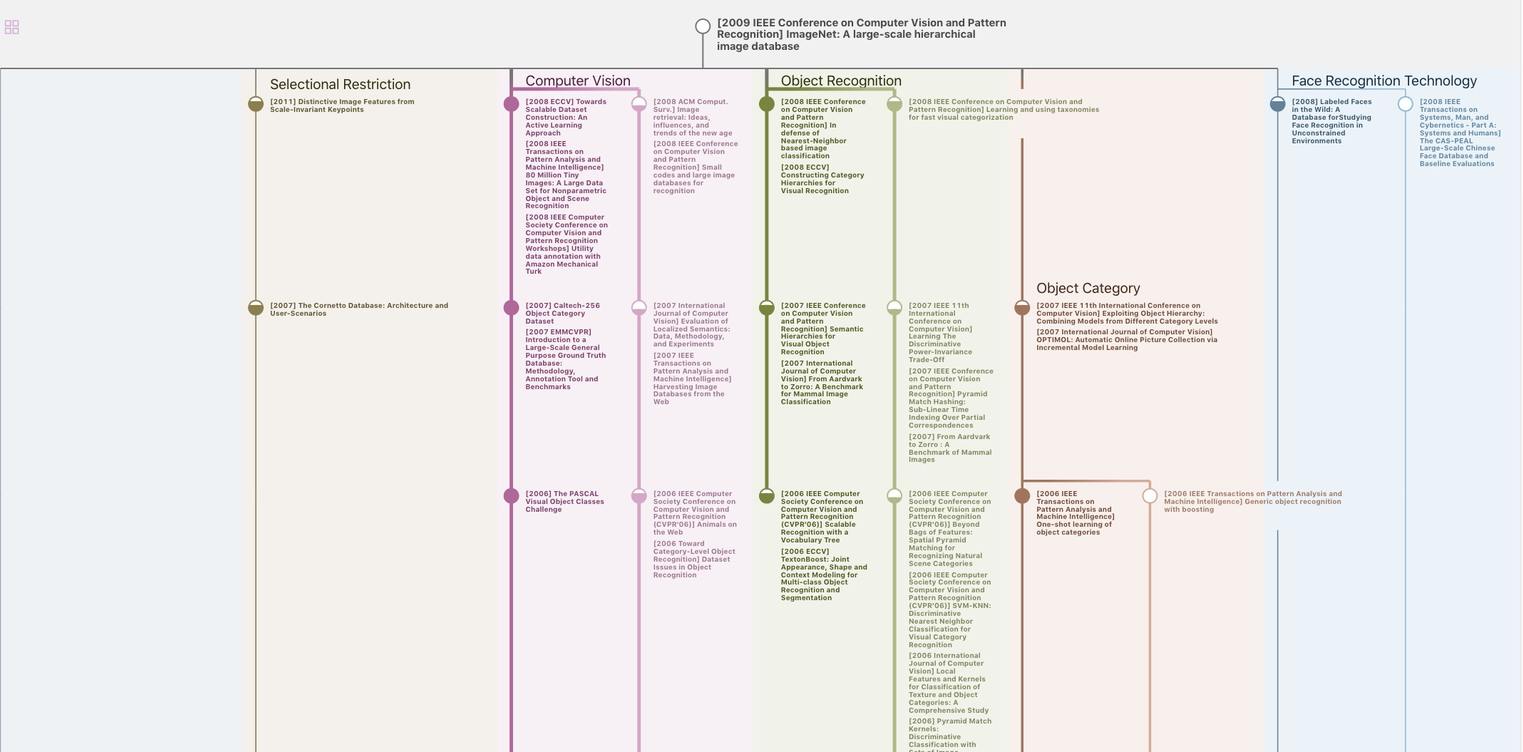
生成溯源树,研究论文发展脉络
Chat Paper
正在生成论文摘要