Toward a machine learning approach for sea ice detection from high resolution ascat measurements
IGARSS 2023 - 2023 IEEE INTERNATIONAL GEOSCIENCE AND REMOTE SENSING SYMPOSIUM(2023)
摘要
In this paper, we present supervised machine learning employed with high-resolution ASCAT data to detect sea ice in the Alaska region for our ultra-high-resolution wind and sea ice ASCAT product. Two machine learning algorithms, Gaussian Naive Bayes (GNB) and Support Vector Machine (SVM) were tested in this analysis. GNB utilizes a feature vector consisting of six ASCAT variables, while SVM employed the same inputs but with additional standardization prior to training. The training target consisted of collocated GDAS ice flag and ASMR-2 ice concentration data. The dataset was balanced and divided into training and testing sets for each model. The results showed that GNB achieved 94% accuracy, while SVM achieved 98% with reduced noise. Consequently, we chose SVM as the final algorithm for near real-time sea ice flag processing. SVM exhibited improved accuracy in identifying the sea ice edge, allowing us to enhance the usability of ultra-high-resolution ASCAT wind data in polar regions.
更多查看译文
关键词
ASCAT,Sea Ice detection,High-Resolution
AI 理解论文
溯源树
样例
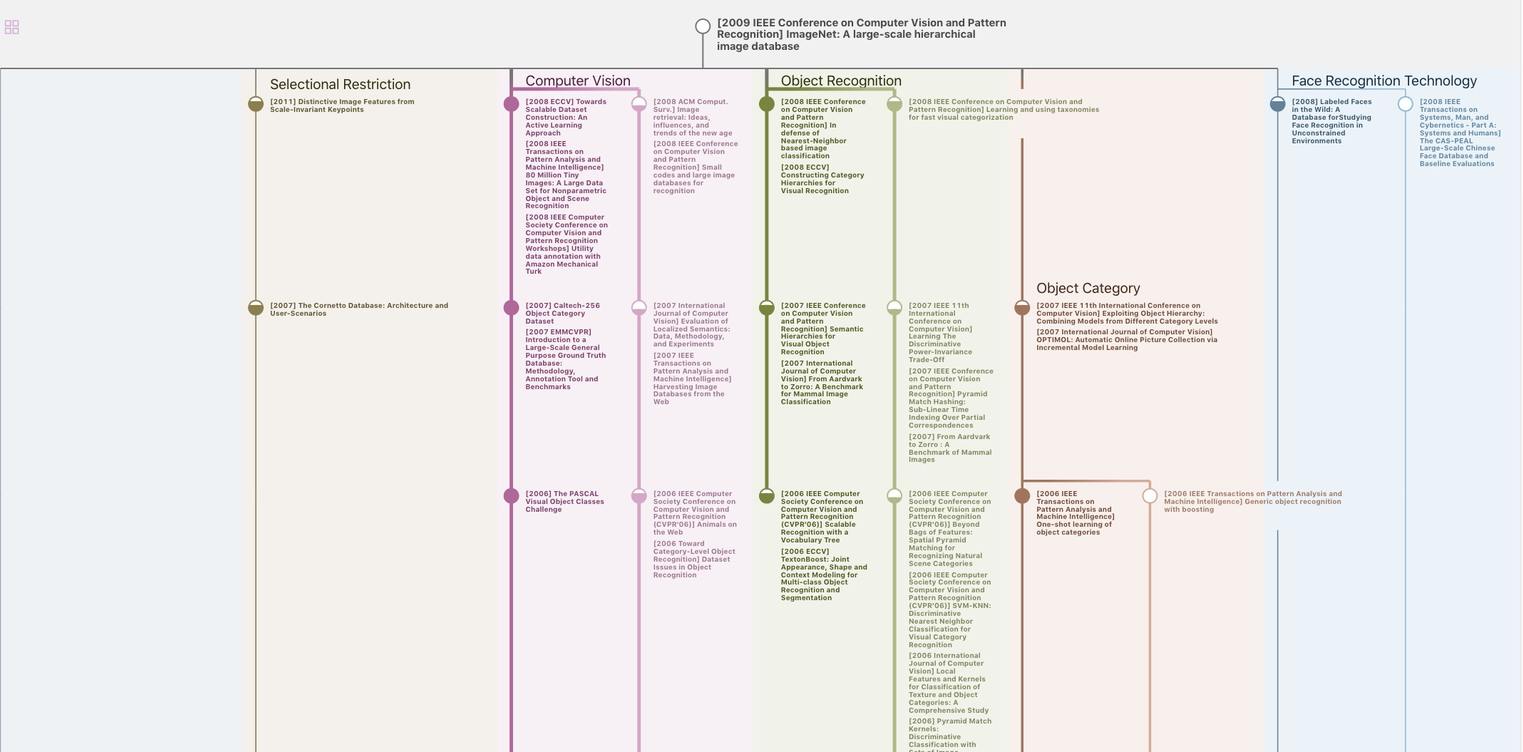
生成溯源树,研究论文发展脉络
Chat Paper
正在生成论文摘要