Bayesian Two-Stage Sequential Change Diagnosis via Sensor Arrays
IEEE Transactions on Information Theory(2023)
摘要
In this paper, we formulate and solve a two-stage Bayesian sequential change diagnosis (SCD) problem in a multi-sensor setting. In the considered problem, a change first occurs at a sensor and then propagates across the sensor array gradually. After a change is detected, we are allowed to continue observing more samples so that we can identify the distribution after the change more accurately. Our goal is to minimize the total cost including delay, false alarm, and misdiagnosis probabilities. We first characterize the optimal SCD rule. Moreover, to address the high computational complexity issue of the optimal SCD rule, we propose a low-complexity threshold SCD rule. We further analyze the asymptotic optimality of the threshold SCD rule. In addition, we investigate how increasing the number of sensors can improve the performance of the proposed threshold SCD rule. Our analysis holds for different sensor array structures, including linear sensor arrays and 2D lattice sensor arrays.
更多查看译文
关键词
sensor arrays,bayesian,two-stage
AI 理解论文
溯源树
样例
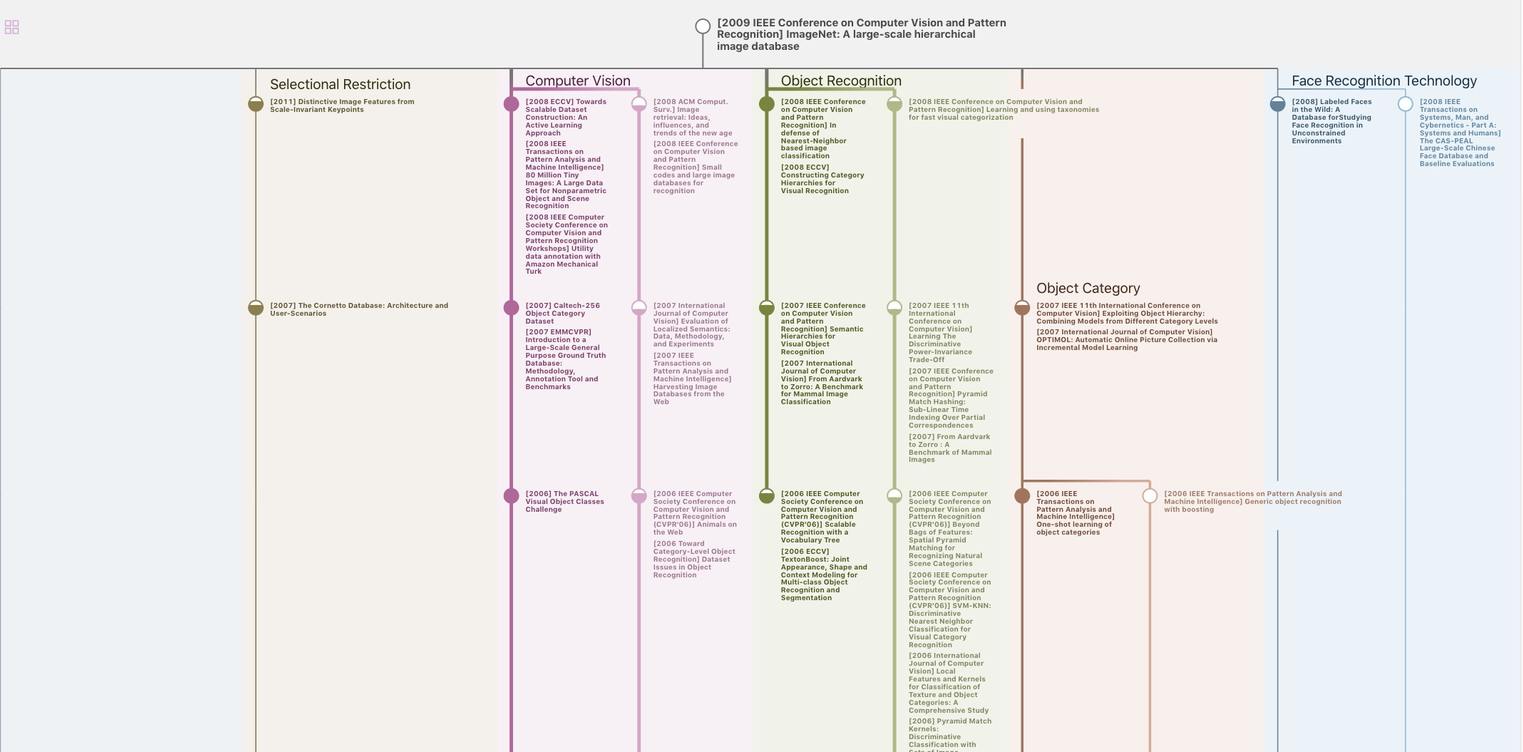
生成溯源树,研究论文发展脉络
Chat Paper
正在生成论文摘要