Efficient Detection of Noise Reviews Over a Large Number of Places
IEEE Access(2023)
Abstract
User reviews have been widely used to extract opinions, complaints, and requirements about a given place or product from the users’ point of view. In the process of collecting user reviews, a lot of reviews irrelevant to a given search keyword are included in the collection result. Such irrelevant reviews can easily be detected using supervised learning algorithms. However, situation changes when the number of places or products that need to be analyzed increases because manual labeling is required for each collected review. This paper presents a method to detect irrelevant reviews efficiently when a large number of places and reviews need to be analyzed. The basic idea of the proposed method is to expand the target of the learning from an individual place to a group consisting of multiple places. The method can be applied properly to any place whose number of reviews is not enough to perform the training because a classifier obtained by training sufficient reviews included in the places of a group can be used for the places with insufficient reviews in the group. As an initial study of this approach, we tried to check through experiments whether the proposed method can provide higher accuracy than the conventional method where the training is performed for individual places. Our experimental results showed that the average f1-score of the group learning was about 0.931 over real data collected online. For the places with less than 100 reviews, the f1-score of the conventional and proposed methods was 0.651 and 0.865, respectively, showing that 21.4% performance improvement.
MoreTranslated text
Key words
noise reviews,efficient detection
AI Read Science
Must-Reading Tree
Example
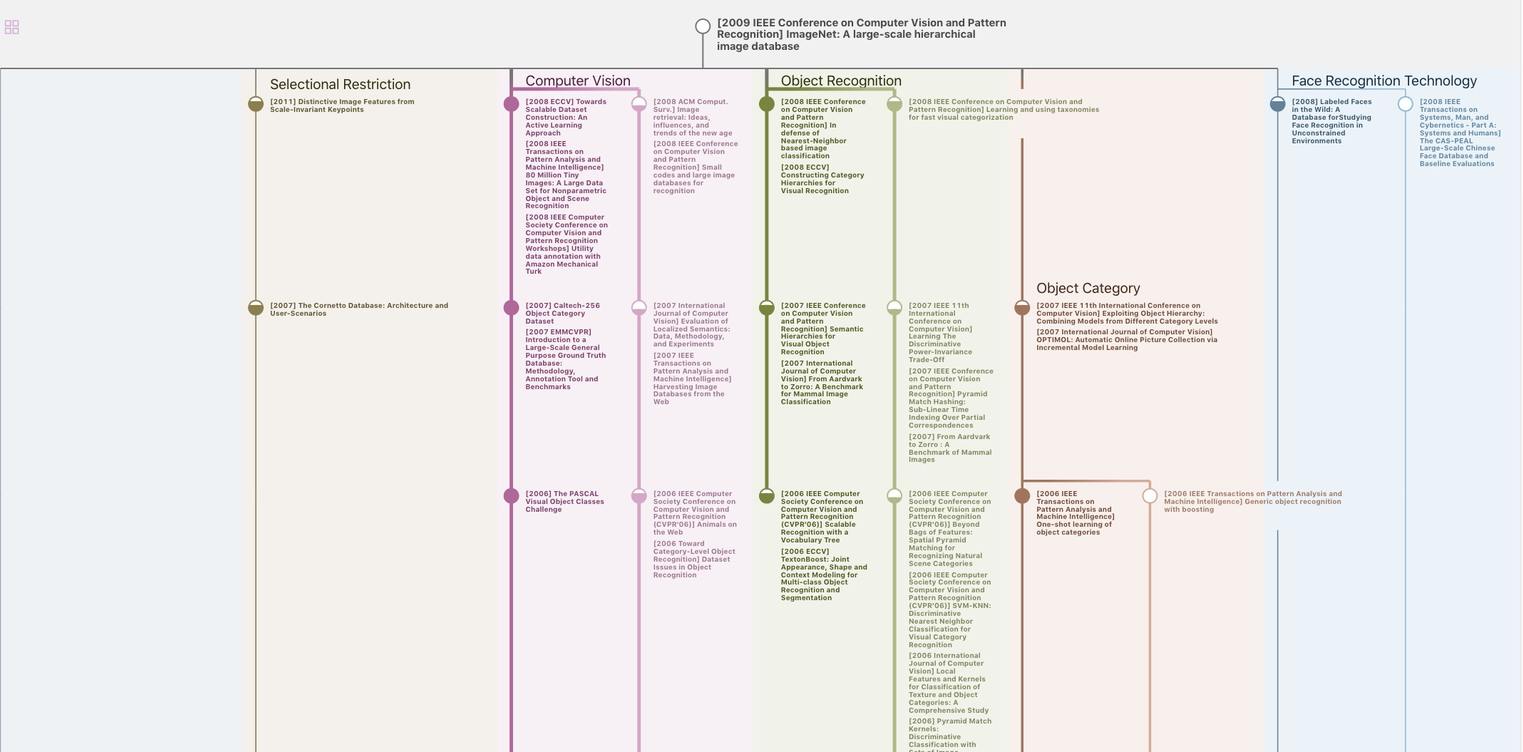
Generate MRT to find the research sequence of this paper
Chat Paper
Summary is being generated by the instructions you defined