Multi-agent graph reinforcement learning for decentralized Volt-VAR control in power distribution systems
INTERNATIONAL JOURNAL OF ELECTRICAL POWER & ENERGY SYSTEMS(2024)
Abstract
Volt/Var control (VVC) is a crucial function in power distribution systems to minimize power loss and maintain voltages within allowable limits. However, incomplete and inaccurate information about the distribution network makes model-based VVC methods difficult to implement in practice. In this paper, we propose a novel multi-agent graph-based deep reinforcement learning (DRL) algorithm named MASAC-HGRN to address the VVC problem under partial observation constraints. Our proposed algorithm divides the power distribution system into several regions, each region treated as an agent. Unlike traditional model-based or global-observation-based DRL methods, our proposed method leverages a practical decentralized training and decentralized execution (DTDE) paradigm to address the partial observation constraints. The well-trained agents gather information only from their interconnected neighbors and realize decentralized local control. Numerical studies with IEEE 33-bus and 123-bus distribution test feeders demonstrate that our proposed MASAC-HGRN algorithm outperforms the state-of-art RL algorithms and traditional model-based approaches in terms of VVC performance. Moreover, the DTDE framework exhibits flexibility and robustness in extensive robustness experiments.
MoreTranslated text
Key words
Decentralized training,Graph network,Multi-agent deep reinforcement learning,Power distribution system,Volt/VAR control
AI Read Science
Must-Reading Tree
Example
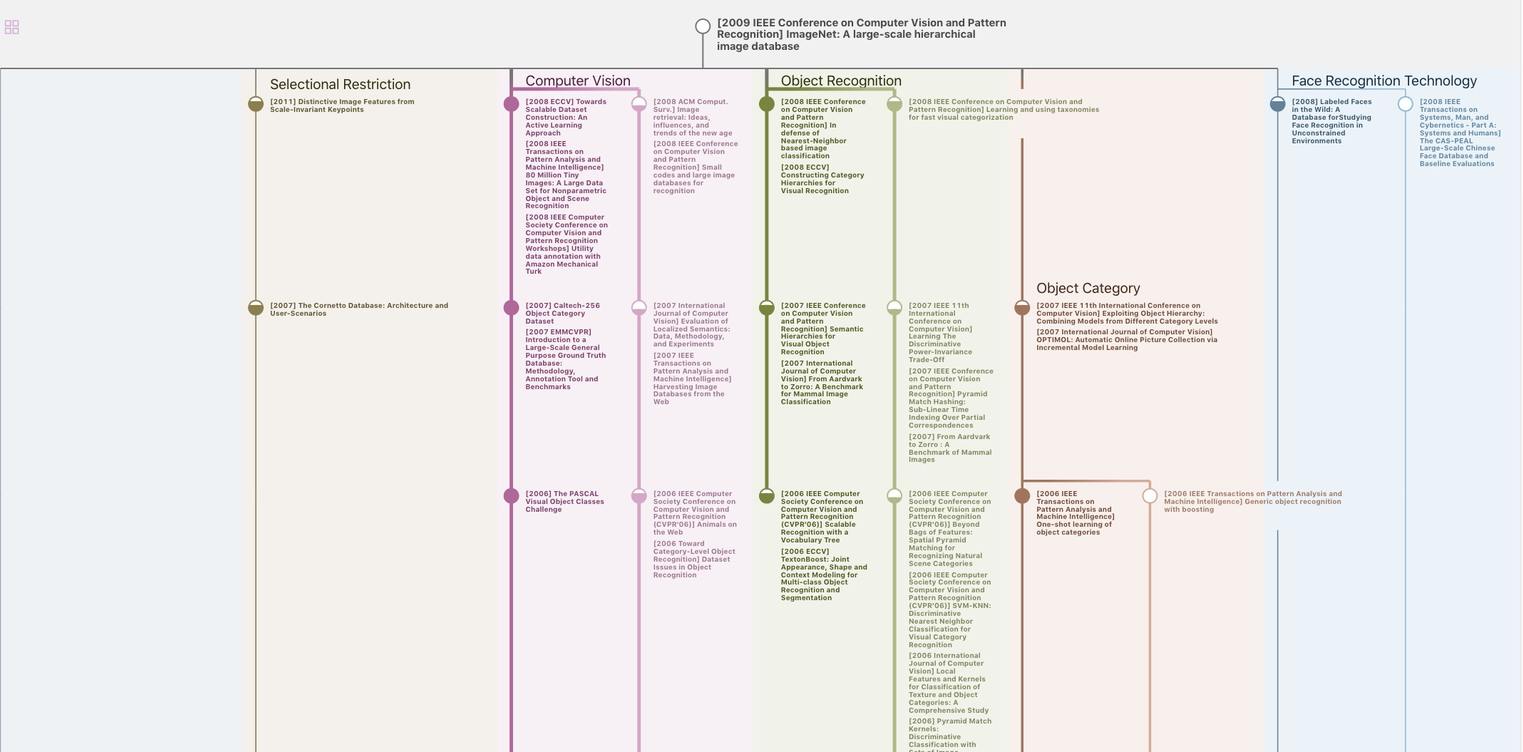
Generate MRT to find the research sequence of this paper
Chat Paper
Summary is being generated by the instructions you defined