Using Bayesian threshold model and machine learning method to improve the accuracy of genomic prediction for ordered categorical traits in fish
Agriculture Communications(2023)
摘要
Ordered categorical traits are commonly used in fish breeding programs as they are easier to obtain than continuous observations. However, most studies treat ordered categorical traits as linear traits and analyze them using linear models, which can lead to a serious reduction in prediction accuracy by violating the basic assumptions of linear models. The aim of this study was to evaluate the advantages of Bayesian threshold model and machine learning method in genomic prediction of ordered categorical traits in fish. The study was based on the analyses of simulated data and real data of Atlantic salmon. Ordinal categorical traits were simulated with varying numbers of categories (2, 3 and 4) and levels of heritabilities (0.1, 0.3 and 0.5). Linear and threshold models with BayesA and BayesCπ methods, as well as a machine learning method, support vector regression with default (SVRdef) and tuning (SVRtuning) hyperparameters were used to investigate their prediction abilities. The results showed that Bayesian threshold models yielded 2.1%, 2.6% and 2.9% higher prediction accuracies on average for 2-, 3- and 4-category traits, respectively, than Bayesian linear models. Furthermore, SVRtuning produced higher prediction accuracy compared with SVRdef and Bayesian threshold models in all scenarios. For real data, Bayesian threshold models yielded 1.2% higher prediction accuracy than Bayesian linear models, and SVRdef and SVRtuning yielded 3.3% and 6.6% higher prediction accuracies than Bayesian methods, respectively. In conclusion, the use of Bayesian threshold model and machine learning method was beneficial for genomic prediction of ordered categorical traits in fish.
更多查看译文
关键词
genomic prediction,categorical traits,bayesian threshold model,machine learning method,fish
AI 理解论文
溯源树
样例
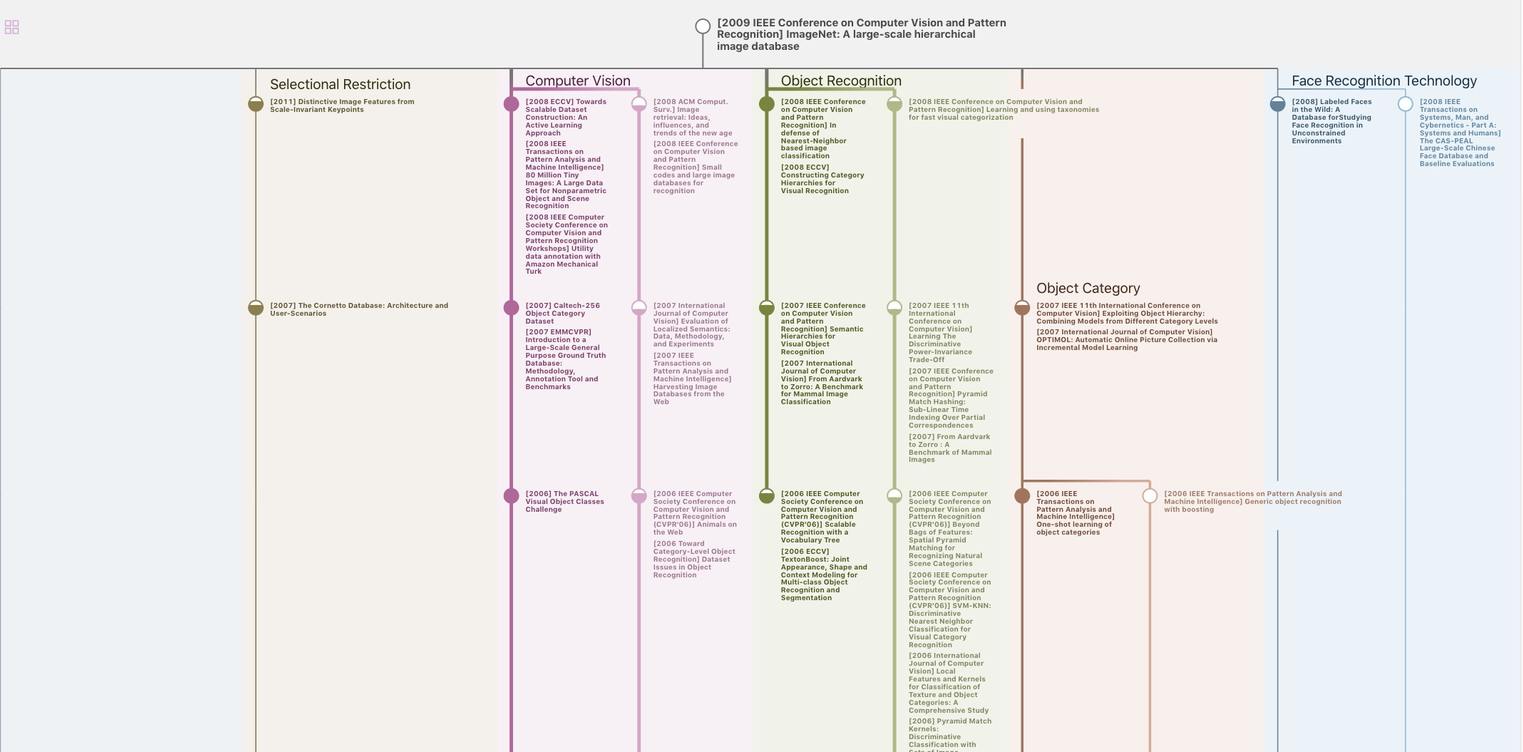
生成溯源树,研究论文发展脉络
Chat Paper
正在生成论文摘要