Enhancing urban real-time PM2.5 monitoring in street canyons by machine learning and computer vision technology
SUSTAINABLE CITIES AND SOCIETY(2024)
Abstract
During peak hours, both pedestrians and drivers face extended exposure to road air pollution, raising the risk of respiratory diseases. Variations in traffic volume, building attributes, and weather conditions in street canyons lead to differing exposure levels across road sections and intersections. Though deploying numerous sensors provides real-time access to PM2.5 levels in each road section, they cannot interpret the impact of weather, traffic, and buildings on these levels. Therefore, in Lanzhou City, we attempted to use Computer Vision Technology (CVT) to extract real-time traffic volume and street-view features from traffic images for PM2.5 concentration prediction, and to interpret the impact of road environment changes on PM2.5 levels. Results show that by using the Extreme Gradient Boosting (XGB) regression model, the coefficient of determination for PM2.5 prediction reaches R2=0.956. Meteorological conditions, traffic volume, and buildings are key variables in predicting road PM2.5 concentrations. Meteorological conditions control the continuous fluctuation of road PM2.5 levels, while traffic volume can lead to sudden changes in PM2.5 levels. The research indicates that the combination of traffic cameras and CVT can acquire road PM2.5 concentrations, contributing significantly to rapidly understanding road pollution status, identifying highly polluted roads, and conducting exposure assessments of roadways.
MoreTranslated text
Key words
PM2.5,Urban roads,Street view,Traffic image,Computer vision technology,Extreme gradient boosting
AI Read Science
Must-Reading Tree
Example
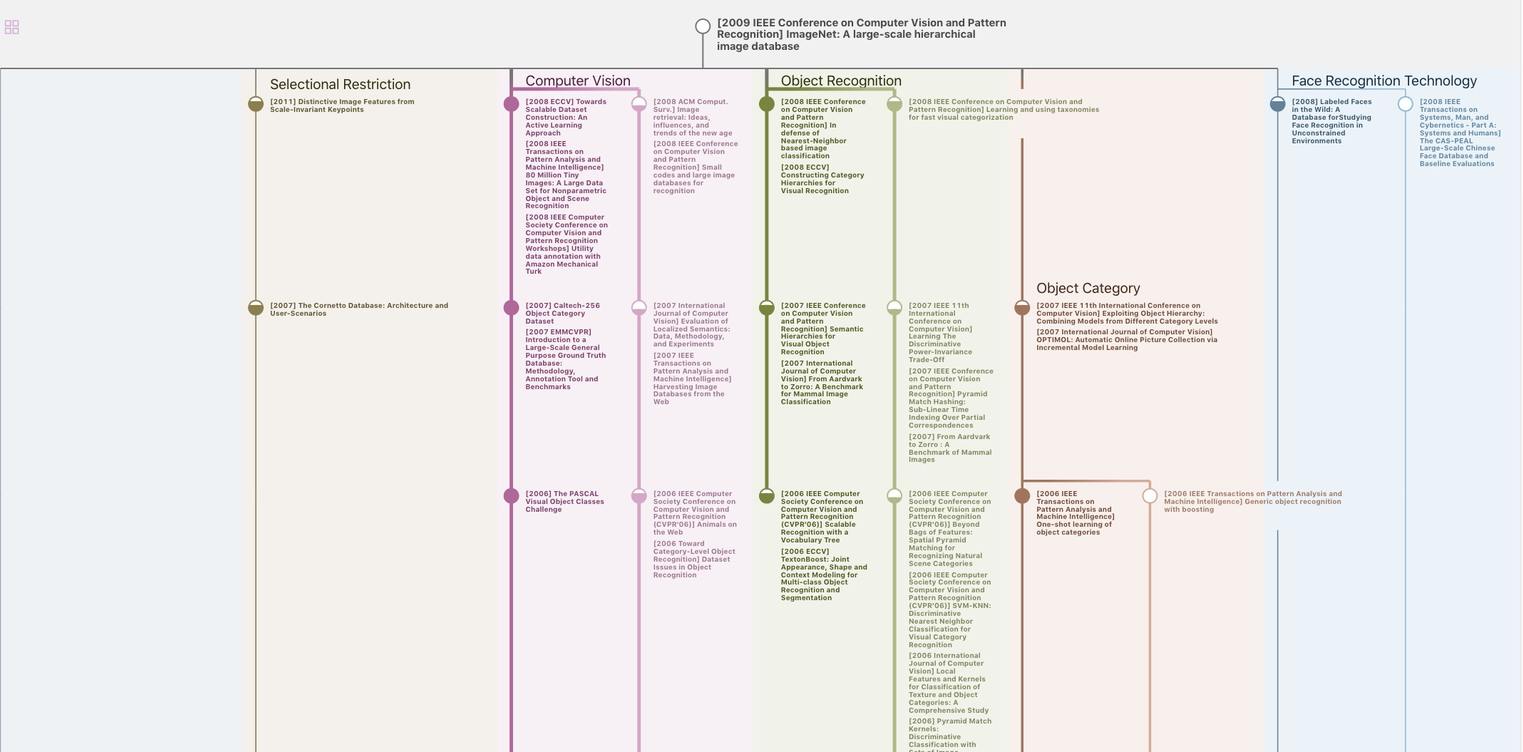
Generate MRT to find the research sequence of this paper
Chat Paper
Summary is being generated by the instructions you defined