MJAR: A novel joint generalization-based diagnosis method for industrial robots with compound faults
ROBOTICS AND COMPUTER-INTEGRATED MANUFACTURING(2024)
摘要
Compound faults inevitably occur in multi-joint industrial robots resulting in excessive vibration. Intelligent diagnosis for the occurrence and position of fault joints can efficiently reduce the maintenance cost. Compared with laboratory disassembly parts, joint diagnosis of in-situ industrial robots is more challenging due to coupling of multiple subsystems and the industrial noise interference. This paper proposes a novel joint generalization-based fault diagnosis method for industrial robots via the multi-joint attention residual network (MJAR) model. Each joint signals are independently input to parallel residual convolution with unidirectional matrix kernel (ResCUM) in multi-joint decoupling attention (MJDA) module, which is exclusively designed combining residual and attention mechanism to provide decoupling capability of compound faults. And the multi-spatial reconstruction (MSR) module based on sparse sampling is design to provide multi-scale feature extraction adapted to real industrial signals. MJAR can diagnose unseen compound fault combinations without using any transfer strategy as the robot joint generalization-based fault diagnosis (RJGFD) framework. Integrated experiment results show that the MJAR is superior to some advanced congener DL methods.
更多查看译文
关键词
Industrial robots,Fault diagnosis,Convolutional Neural Networks,Joints,Compound faults
AI 理解论文
溯源树
样例
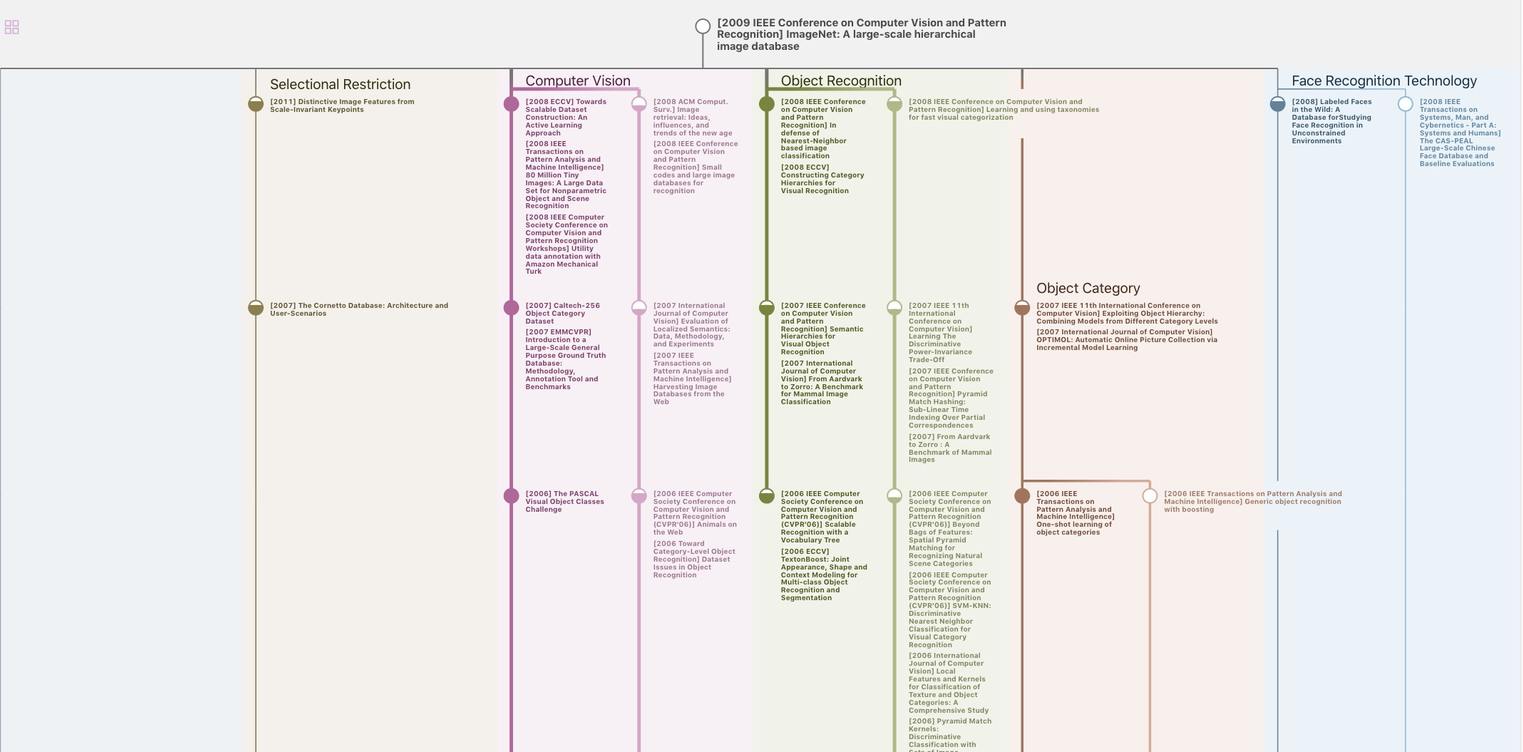
生成溯源树,研究论文发展脉络
Chat Paper
正在生成论文摘要