GAM-based individual difference measures for L2 ERP studies
Research Methods in Applied Linguistics(2023)
摘要
ERPs (Event-Related Potentials) have become a widely-used measure to study second language (L2) processing. To study individual differences, traditionally a component outcome measure is calculated by averaging the amplitude of a participant's brain response in a pre-specified time window of the ERP waveform in different conditions (e.g., the ‘Response Magnitude Index’; Tanner, Mclaughlin, Herschensohn & Osterhout, 2013). This approach suffers from the problem that the definition of such time windows is rather arbitrary, and that the result is sensitive to outliers as well as participant variation in latency. The latter is particularly problematic for studies on L2 processing. Furthermore, the size of the ERP response (i.e., amplitude difference) of an L2 speaker may not be the best indicator of near-native proficiency, as native speakers also show a great deal of variability in this respect, with the ‘robustness’ of an L2 speaker's ERP response (i.e., how consistently they show an amplitude difference) potentially being a more useful indicator. In this paper we introduce a novel method for the extraction of a set of individual difference measures from ERP waveforms. Our method is based on participants’ complete waveforms for a given time series, modelled using generalized additive modelling (GAM; Wood, 2017). From our modelled waveform, we extract a set of measures which are based on amplitude, area and peak effects. We illustrate the benefits of our method compared to the traditional Response Magnitude Index with data on the processing of grammatical gender violations in 66 Slavic L2 speakers of German and 29 German native speakers. One of our measures in particular appears to outperform the others in characterizing differences between native speakers and L2 speakers, and captures proficiency differences between L2 speakers: the ‘Normalized Modelled Peak’. This measure reflects the height of the (modelled) peak, normalized against the uncertainty of the modelled signal, here in the P600 search window. This measure may be seen as a measure of peak robustness, that is, how reliable the individual is able to show a P600 effect, largely independently of where in the P600 window this occurs. We discuss implications of our results and offer suggestions for future studies on L2 processing. The code to implement these analyses is available for other researchers.
更多查看译文
关键词
individual difference measures,gam-based
AI 理解论文
溯源树
样例
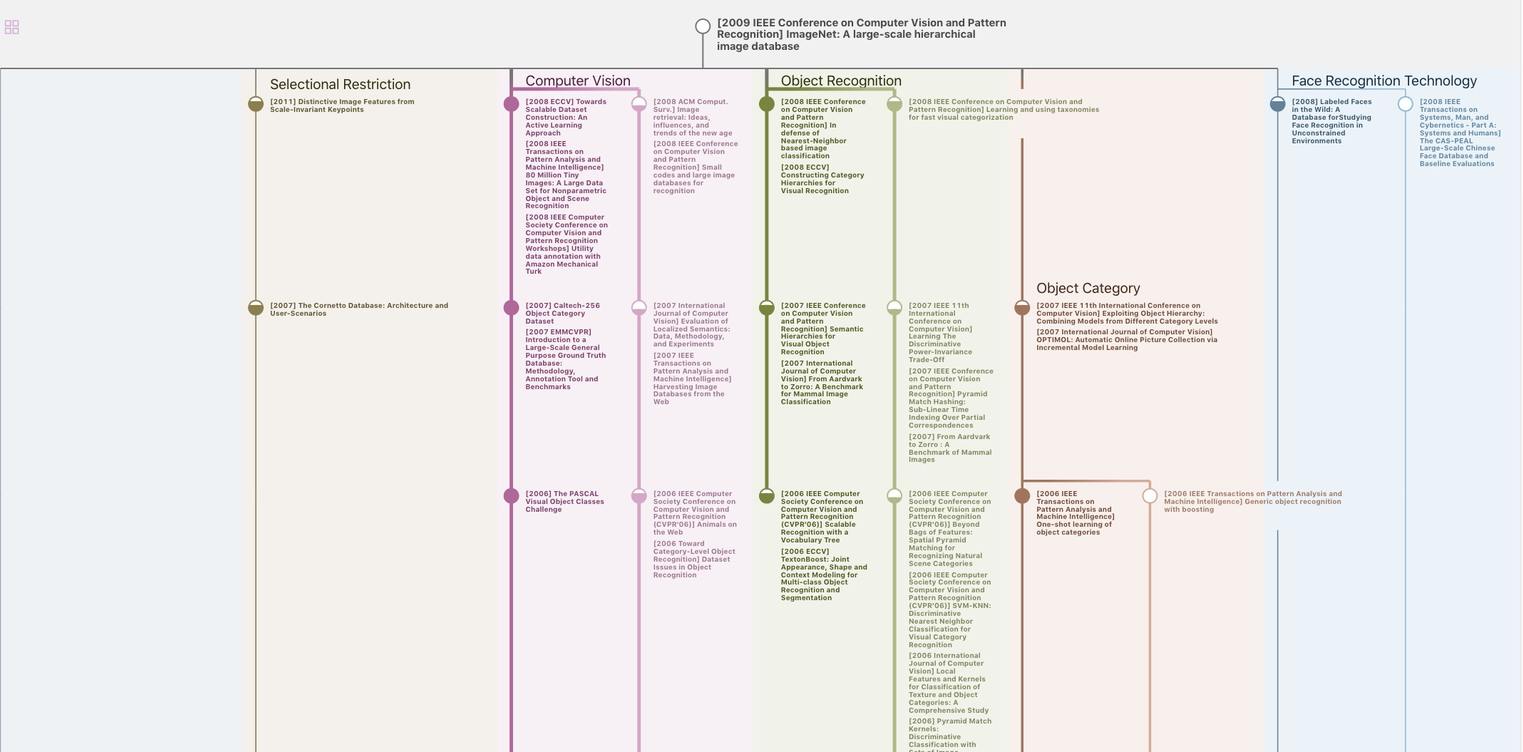
生成溯源树,研究论文发展脉络
Chat Paper
正在生成论文摘要