Anomaly detection in telemetry data using a jointly optimal one-class support vector machine with dictionary learning
RELIABILITY ENGINEERING & SYSTEM SAFETY(2024)
摘要
Anomaly detection based on telemetry data is a major issue in satellite health monitoring, given that it can identify unusual or unexpected events to avoid serious accidents and ensure the safety and reliability of operations. This study proposes a jointly optimal one-class support vector machine with dictionary learning (JODL-OC) framework for multivariate anomaly detection in telemetry. Dictionary learning (DL) extracts the correlation and local dynamics of time series into a sparse matrix, and the one-class support vector machine (OCSVM) detects anomalies in the transformed sparse space. The optimisation objective of DL is modified by introducing a novel regularisation term called soft-boundary OCSVM, so that the JODL-OC seamlessly integrates DL, sparse representation, and classifier training into a unified model to jointly learn the features and decision boundary. Furthermore, we propose an iterative approach to solve the optimisation function and obtain the optimal JODL-OC framework for anomaly detection. A case analysis based on satellite antenna telemetry data reveals that the proposed method improves the detection precision, F1-score, FPR and FAR compared to other existing anomaly detection methods, particularly to illustrate the superior performance in correlation anomalies.
更多查看译文
关键词
Anomaly detection,Telemetry data,Dictionary learning,OCSVM,Time series
AI 理解论文
溯源树
样例
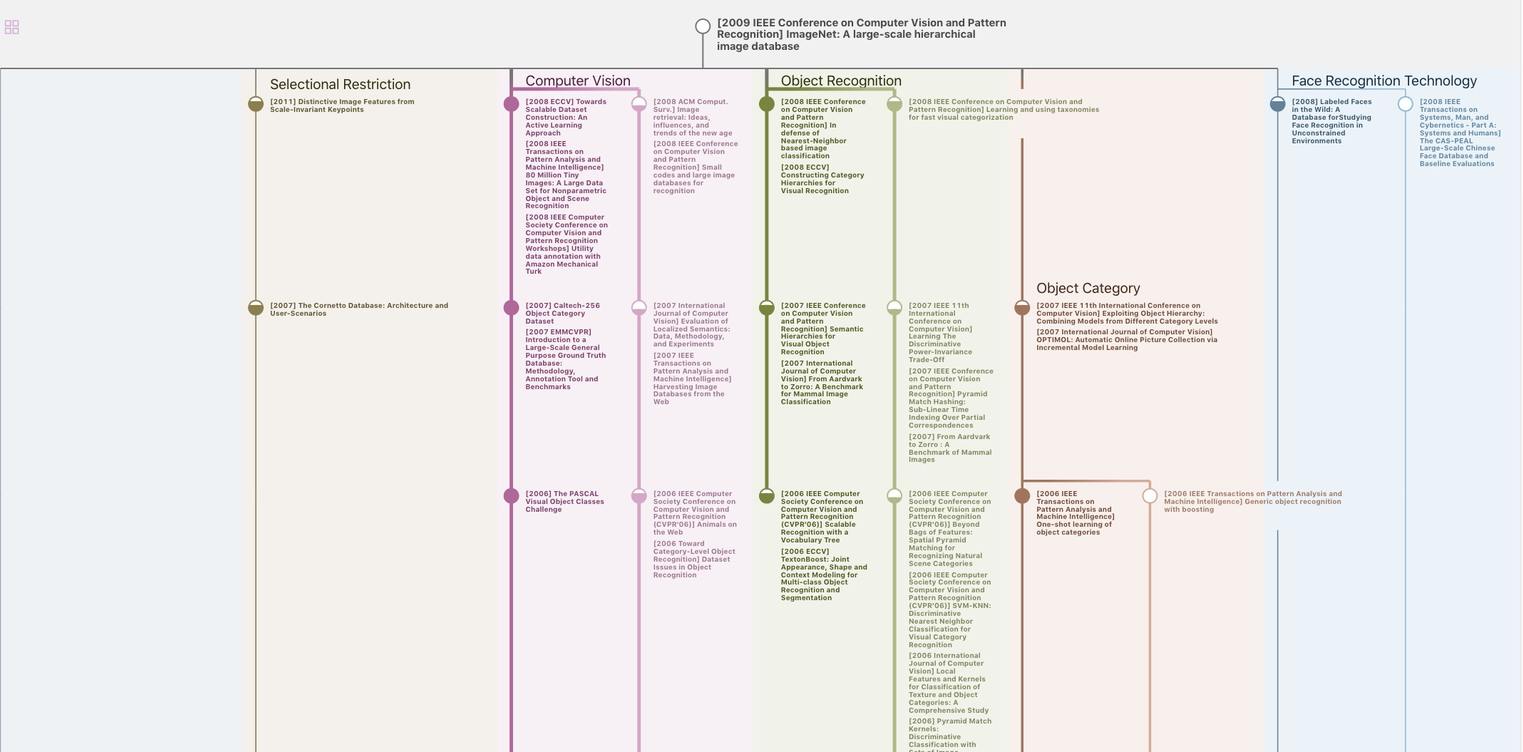
生成溯源树,研究论文发展脉络
Chat Paper
正在生成论文摘要