A novel autoencoder modeling method for intelligent assessment of bearing health based on Short-Time Fourier Transform and ensemble strategy
PRECISION ENGINEERING-JOURNAL OF THE INTERNATIONAL SOCIETIES FOR PRECISION ENGINEERING AND NANOTECHNOLOGY(2024)
摘要
In recent years, deep learning (DL) has developed rapidly in mechanical fault diagnosis. However, in the process of developing rolling bearing fault diagnosis DL model, especially for multiple-category fault diagnosis, the selection of hyperparameters of DL models is critical and difficult. This paper proposed a novel method for an intelligent assessment of bearing health based on Short-Time Fourier Transform (STFT) and ensemble strategy, namely Ensemble STFT-multi-AEs networks (ESAEs). The method was composed of four steps. Firstly, the vibration signal was converted into three-channel time-frequency maps by the STFT. Secondly, three multiautoencoders (multi-AEs) networks were established using the three-channel time-frequency maps, and the multi-channel features extracted by the three networks were fused. Thirdly, a series of multi-AE networks were built based on 11 activation functions (AFs) with different characteristics such as output ranges, monotonicity, smoothness, etc. The consensus strategy was adopted to integrate the modeling results of each sub-model, and the advantages of each member model were analyzed. Finally, a pyramid grading strategy was applied to the member models with larger weights in the 11 sub-models for the second allocation of weights to eliminate the influence of extreme models on the stability of ensemble results. The results show that the ESAEs method can effectively and automatically extract the deep vibration features of bearings, reducing the parameter selection steps brought by AFs. The pyramid grading strategy can effectively balance the difference of prediction results of AE models built with different AFs. The ESAEs model achieved the best detection results for locomotive bearing defects with an accuracy of 99.2%. In addition, the ESAEs method still maintains good detection performance and stability in the fault diagnosis of data imbalance.
更多查看译文
关键词
Mechanical fault diagnosis,Short -time fourier transform,Activation functions,Multiple autoencoders,Ensemble strategy
AI 理解论文
溯源树
样例
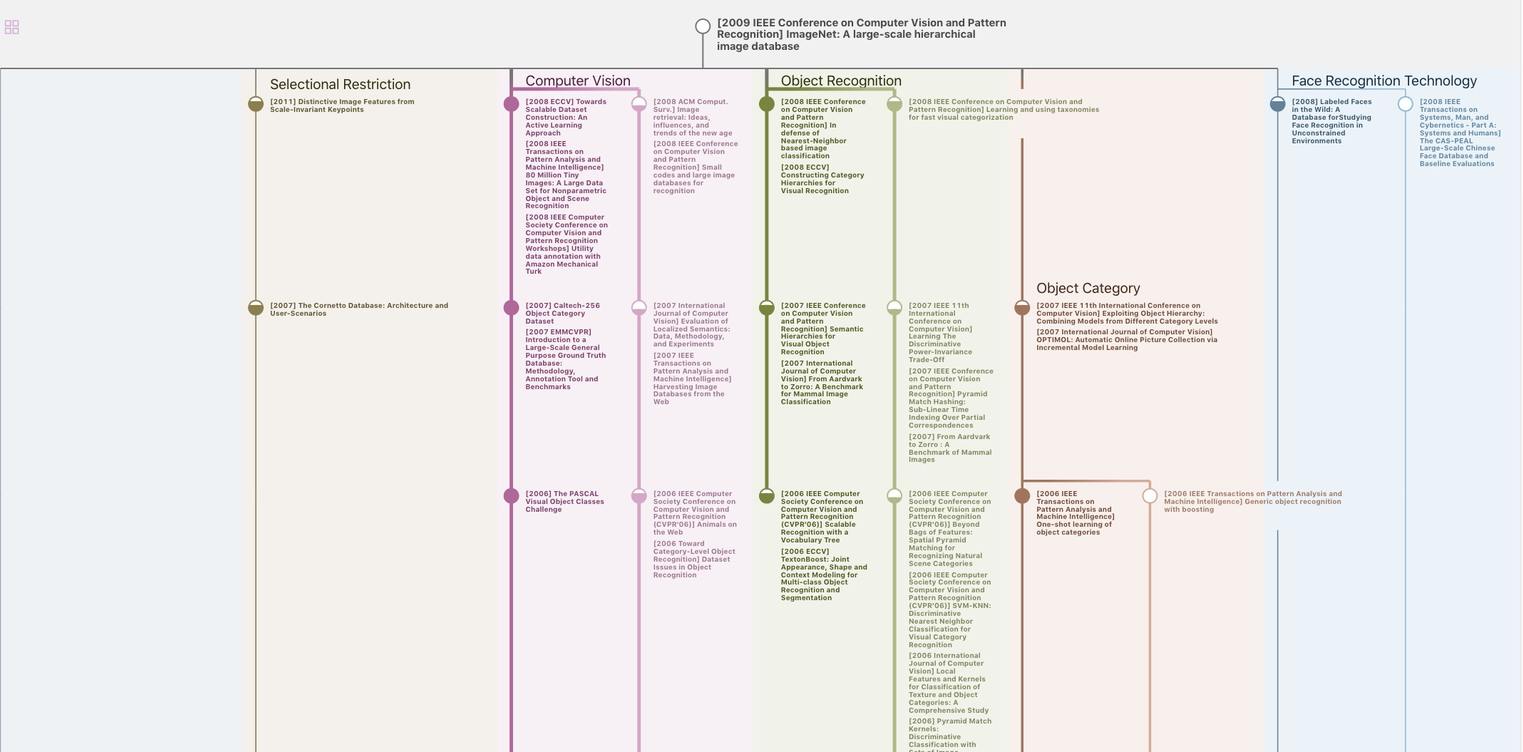
生成溯源树,研究论文发展脉络
Chat Paper
正在生成论文摘要