Fast anchor graph preserving projections
PATTERN RECOGNITION(2024)
摘要
The existing graph-based dimensionality reduction algorithms need to learn an adjacency matrix or construct it in advance, therefore the time complexity of the graph-based dimensionality reduction algorithms is not less than O(n(2) d) , where n denotes the number of samples, d denotes the number of dimensions. Moreover, the existing dimensionality reduction algorithms do not consider the cluster information in the original space, resulting in the weakening or even loss of valuable information after dimensionality reduction. To address the above problems, we propose Fast Anchor Graph Preserving Projections (FAGPP), which learns the projection matrix, the anchors and the membership matrix at the same time. Especially, FAGPP has a built-in Principal Component Analysis (PCA) item, which makes our model not only deal with the cluster information of data, but also deal with the global information of data. The time complexity of FAGPP is O(nmd) , where m denotes the number of the anchors and m is much less than n. We propose a novel iterative algorithm to solve the proposed model and the convergence of the algorithm is proved theoretically. The experimental results on a large number of high-dimensional benchmark image data sets demonstrate the efficiency of FAGPP. The data sets and the source code are available from https://github.com/511lab/FAGPP.
更多查看译文
关键词
Dimensionality reduction,Principal component analysis,Anchor graph,Unsupervised learning
AI 理解论文
溯源树
样例
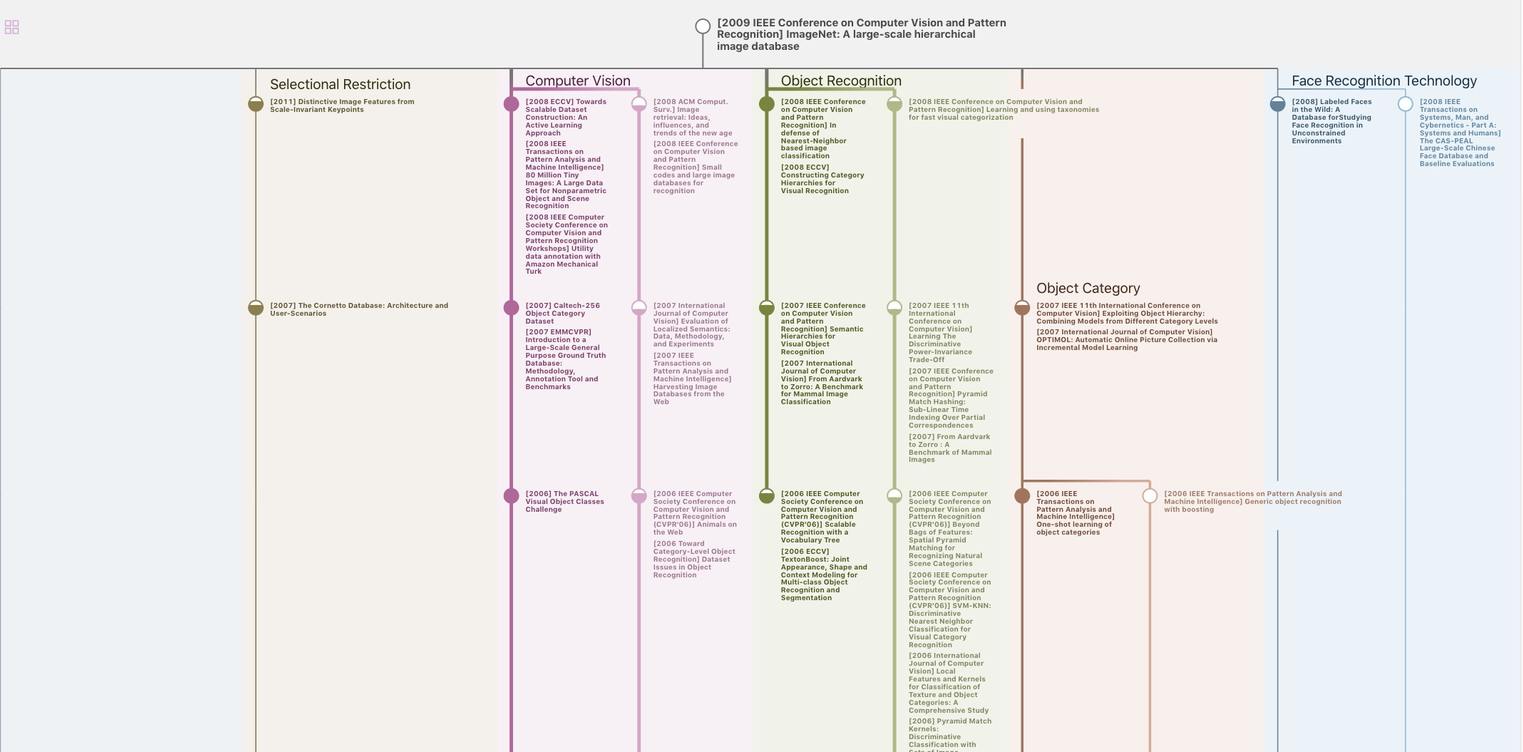
生成溯源树,研究论文发展脉络
Chat Paper
正在生成论文摘要