Bandgap prediction of ABX3-type perovskites using Broad Learning System
Materials Today Communications(2023)
摘要
Perovskite materials have attracted significant attention of scholars at home and abroad due to their excellent photoelectric properties, with the bandgap being a crucial factor that directly determines the performance of perovskite solar cells. However, density functional theory (DFT) calculations of bandgap are time-consuming and costly. To address this issue, Broad learning System (BLS), a data-driven machine learning algorithm, is proposed to predict the bandgap of hybrid organic-inorganic halide perovskites using a dataset of 227 ABX3-type perovskites with experimental bandgap values, and the stoichiometric ratio of positions A, B, X of perovskite as input features. The results demonstrate that BLS algorithm provides superior predictions compared to Support Vector Regression (SVR), Multi-Layer Perceptron (MLP) and Random Forest (RF). BLS algorithm has a lower root mean square error (RMSE), and a higher correlation coefficient (R2), with RMSE being 0.0855 eV and R2 reaching 92.24 %. This work indicates that BLS can be a powerful and potential tool for predicting the bandgap of perovskites.
更多查看译文
关键词
Bandgap, ABX3-type perovskites, Broad Learning System, Machine learning
AI 理解论文
溯源树
样例
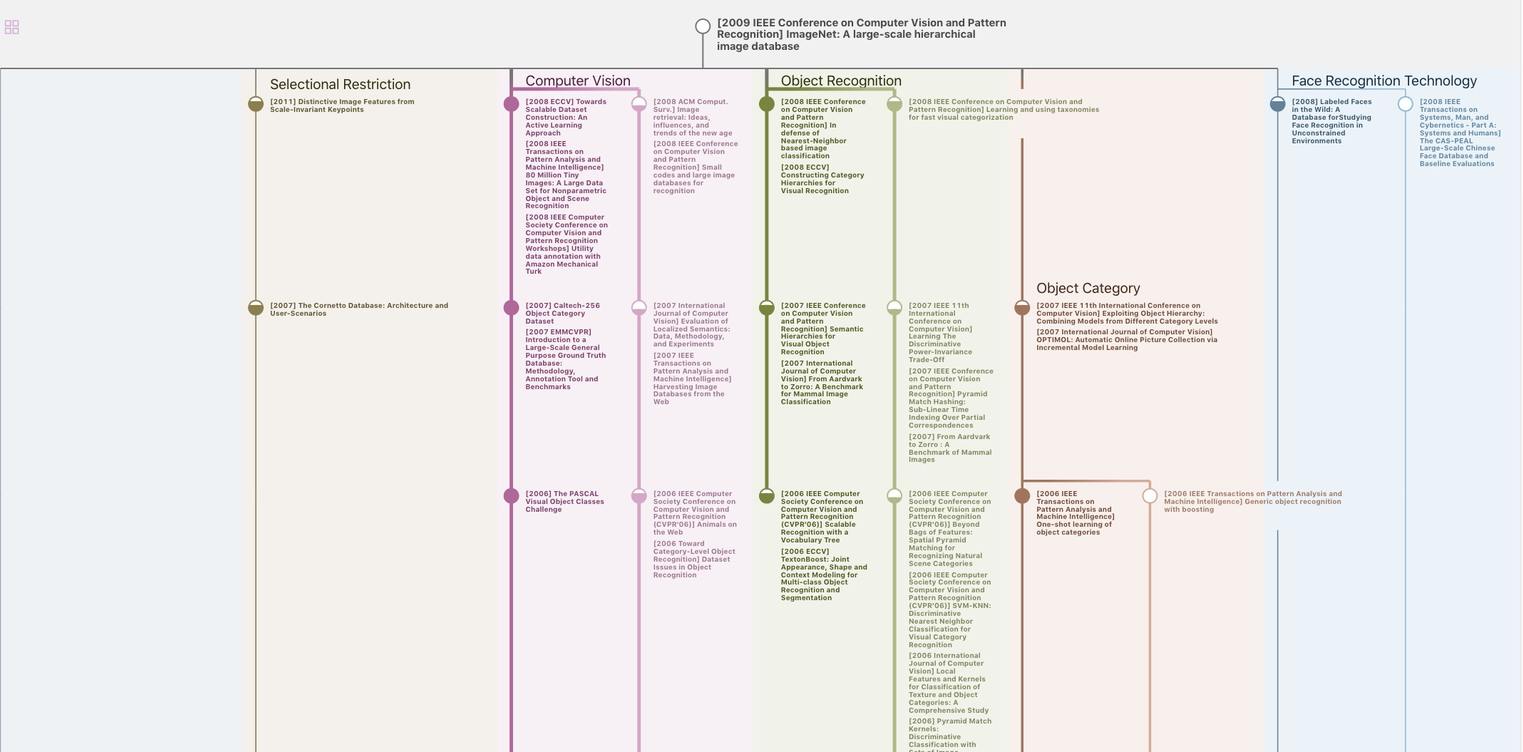
生成溯源树,研究论文发展脉络
Chat Paper
正在生成论文摘要