Error homogenization in physics-informed neural networks for modeling in manufacturing
Journal of Manufacturing Systems(2023)
摘要
Physics-informed neural networks (PINNs) have demonstrated effectiveness in solving partial differential equations (PDEs) associated with manufacturing scenarios, due to their physically interpretable training logic. One limitation has been that PINNs often exhibit heterogenous error maps and high-error “hot spots” throughout the solution domain, which reduce not only the solutions’ accuracy but also their overall consistency with the physical laws. This study addresses this limitation by presenting an efficient and error-aware PINN ensembling technique for error homogenization in solving manufacturing problems. Specifically, a PINN is first established by constraining its training process through a manufacturing specific PDE and corresponding boundary conditions to ensure physical consistency. Next, the loss landscape in the neighborhood of three PINNs trained with varying network parameter initialization is sampled to generate a PINN ensemble. Finally, the outputs of the ensemble members are combined through an inverse error-weighted average to yield the prediction of the PDE solution. Evaluation using the Allen-Cahn PDE, which describes phase separation in the solidification of metallic alloys, shows that the developed method reduces the average prediction error by 63% and error standard deviation by 30% across the solution space, demonstrating its effectiveness for PINN error reduction and homogenization. Additionally, the method has also demonstrated 96% reduction in the computational time as compared to conventional ensembling methods.
更多查看译文
关键词
Physics-informed neural network,Ensemble,Partial differential equation,Error homogenization,Allen-Cahn equation
AI 理解论文
溯源树
样例
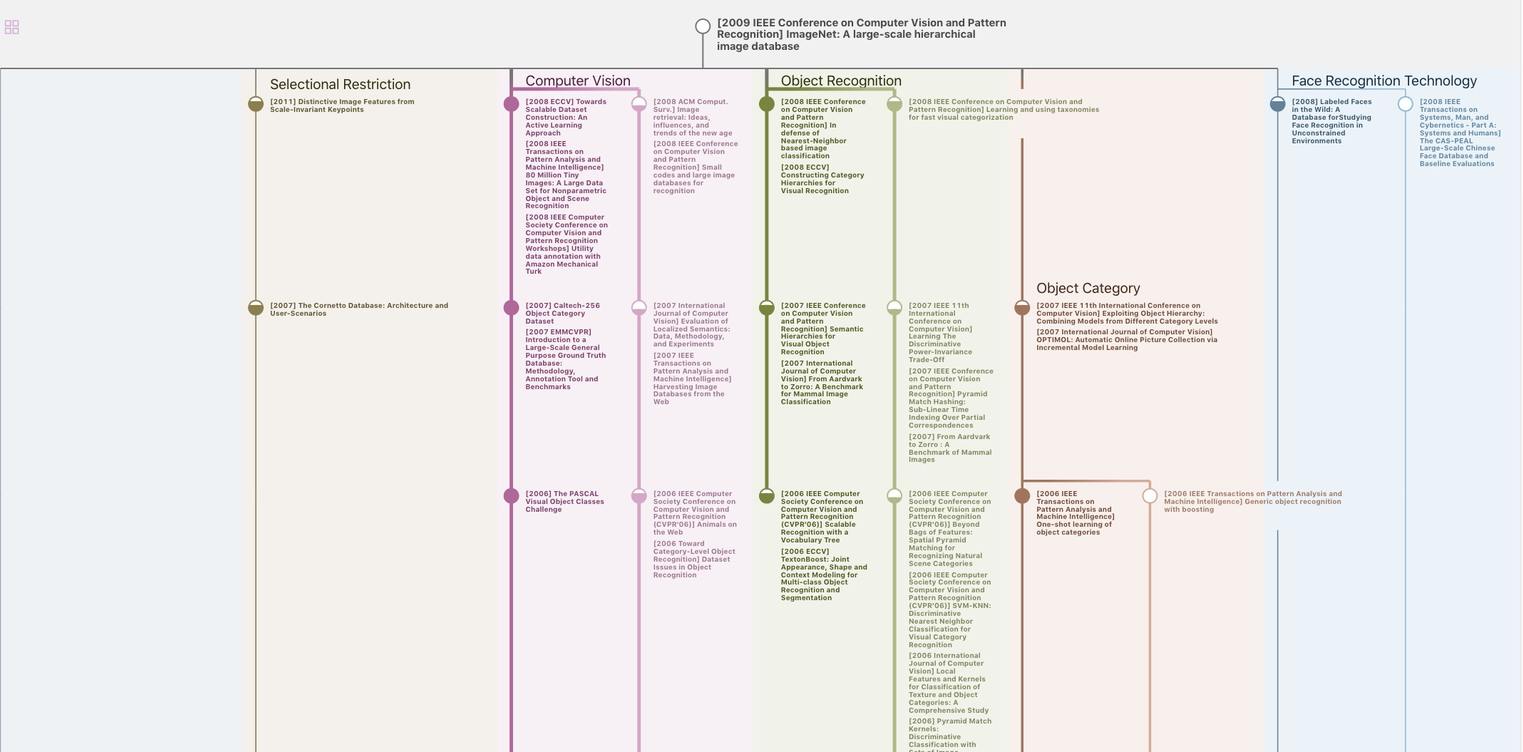
生成溯源树,研究论文发展脉络
Chat Paper
正在生成论文摘要