A novel self-attention model based on cosine self-similarity for cancer classification of protein mass spectrometry
International Journal of Mass Spectrometry(2023)
摘要
Mass spectrometry has become a popular tool for cancer classification. A novel self-attention deep learning model based on cosine self-similarity was proposed to classify cancer by mass spectrometry. First, a primary feature vector is dimensionally reduced by two fully connected layers. Second, the feature vector is transformed into the 2D feature matrix, which can be used to calculate the cosine self-similarity matrix of the self-attention model. Next, three convolutional layers are used to extract the refined feature matrix. Finally, the refined feature matrix is fed into the multi-layer fully-connected network to classify the mass spectra. Experimental results of ovarian and prostate cancer demonstrate that the proposed method outperforms the other methods.
更多查看译文
关键词
protein mass spectrometry,mass spectrometry,cancer classification,self-attention,self-similarity
AI 理解论文
溯源树
样例
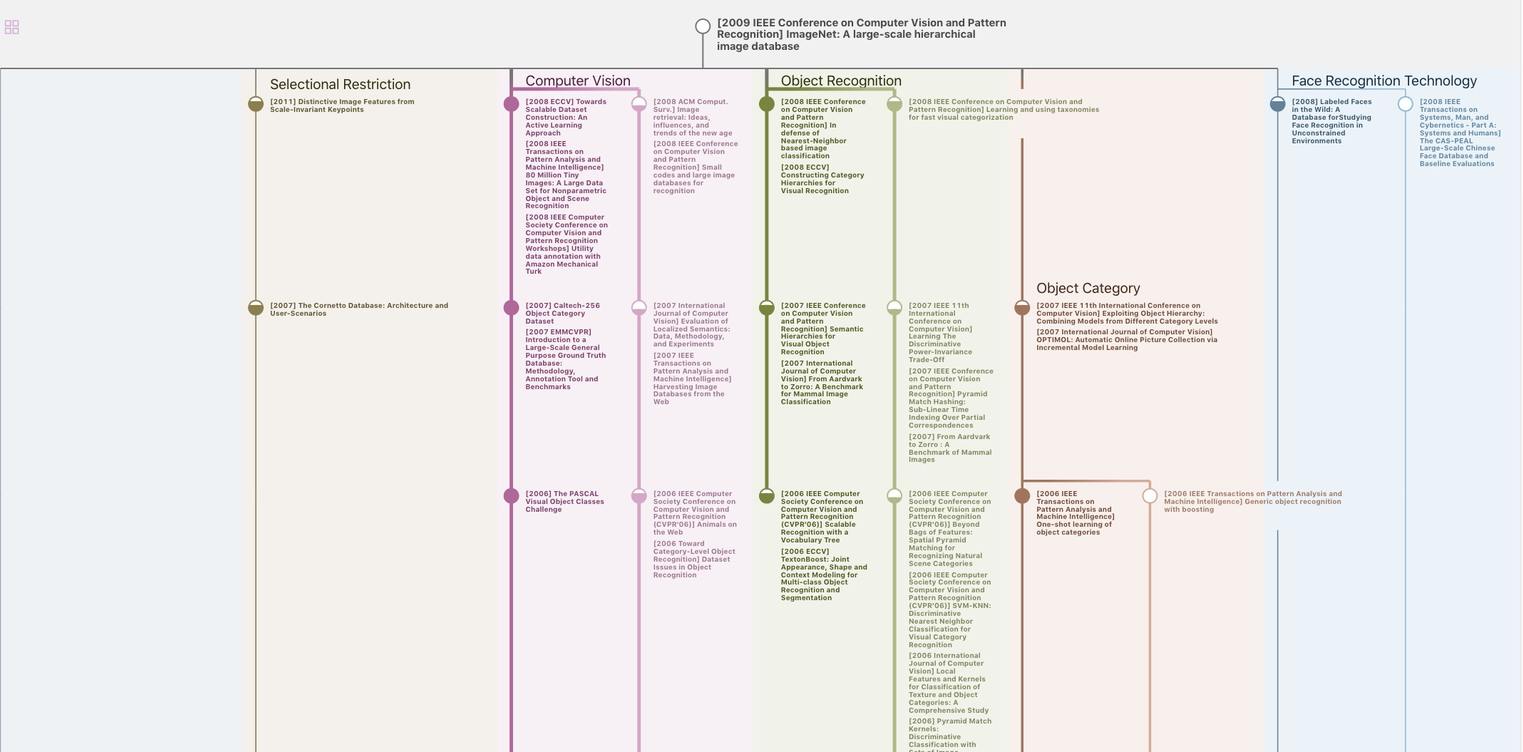
生成溯源树,研究论文发展脉络
Chat Paper
正在生成论文摘要