Addressing data scarcity in protein fitness landscape analysis: A study on semi-supervised and deep transfer learning techniques
INFORMATION FUSION(2024)
摘要
This paper presents a comprehensive analysis of deep transfer learning methods, supervised methods, and semi-supervised methods in the context of protein fitness prediction, with a focus on small datasets. The analysis includes the exploration of the combination of different data sources to enhance the performance of the models. While deep learning and deep transfer learning methods have shown remarkable performance in situations with abundant data, this study aims to address the more realistic scenario faced by wet lab researchers, where labeled data is often limited. The novelty of this work lies in its examination of deep transfer learning in the context of small datasets and its consideration of semi-supervised methods and multi-view strategies. While previous research has extensively explored deep transfer learning in large dataset scenarios, little attention has been given to its efficacy in small dataset settings or its comparison with semi-supervised approaches. Our findings suggest that deep transfer learning, exemplified by ProteinBERT, shows promising performance in this context compared to the rest of the methods across various evaluation metrics, not only in small dataset contexts but also in large dataset scenarios. This highlights the robustness and versatility of deep transfer learning in protein fitness prediction tasks, even with limited labeled data. The results of this study shed light on the potential of deep transfer learning as a state-of-the-art approach in the field of protein fitness prediction. By leveraging pre-trained models and fine-tuning them on small datasets, researchers can achieve competitive performance surpassing traditional supervised and semi -supervised methods. These findings provide valuable insights for wet lab researchers who face the challenge of limited labeled data, enabling them to make informed decisions when selecting the most effective methodology for their specific protein fitness prediction tasks. Additionally, the study investigated the combination of two different sources of information (encodings) through our enhanced semi-supervised methods, yielding noteworthy results improving their base model and providing valuable insights for further research. The presented analysis contributes to a better understanding of the capabilities and limitations of different learning approaches in small dataset scenarios, ultimately aiding in the development of improved protein fitness prediction methods.
更多查看译文
关键词
Bioinformatics,Machine learning,Transfer learning,Semi-supervised learning,Protein fitness prediction,Small datasets
AI 理解论文
溯源树
样例
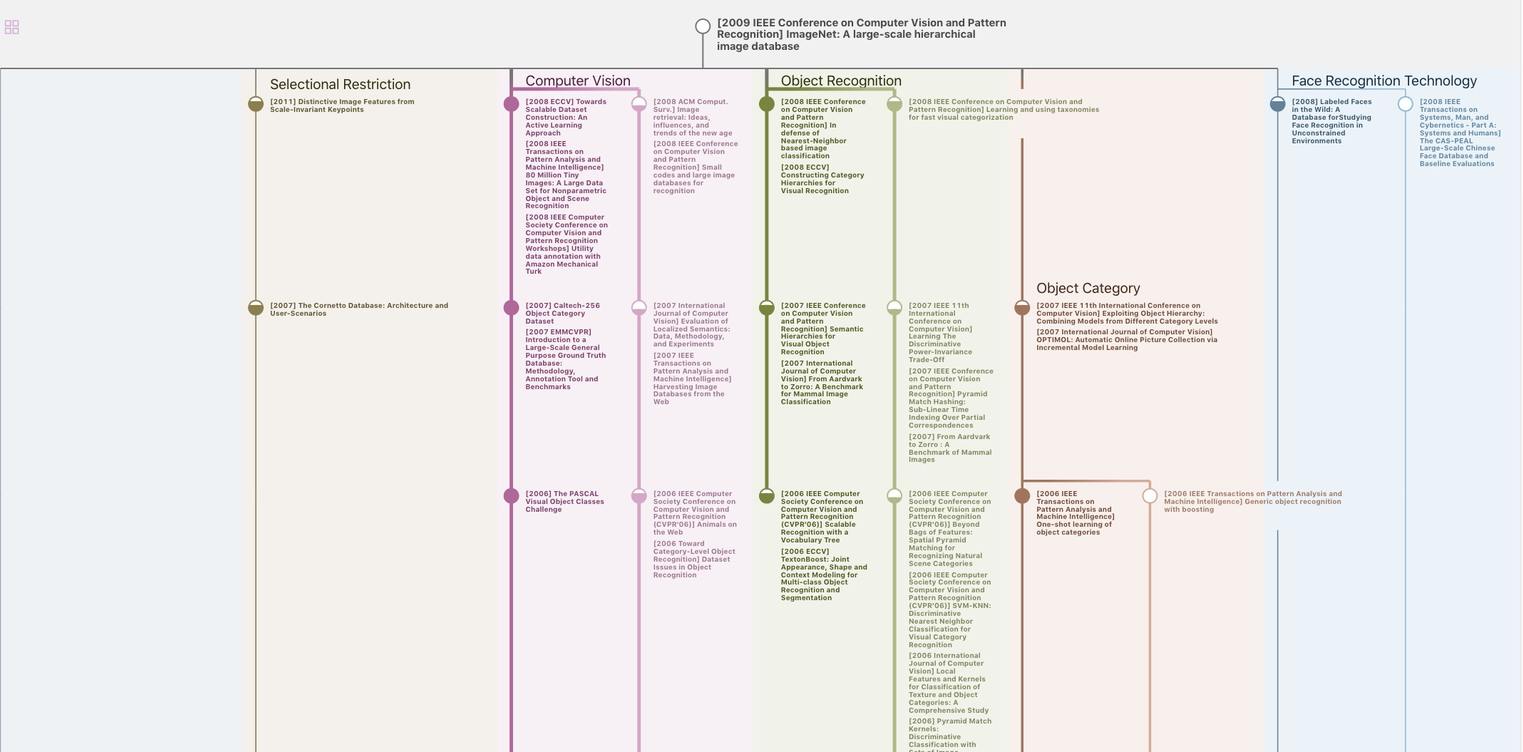
生成溯源树,研究论文发展脉络
Chat Paper
正在生成论文摘要