A hybrid model for post-treatment mortality rate classification of patients with breast cancer
Healthcare Analytics(2023)
摘要
Terminal cancer is not curable and eventually results in death. Breast cancer (BC) is a prevalent malignancy affecting women. Although there are prognostic indicators, BC prognosis is still challenging because of the intricate connections between various survival factors and influencing factors. This study proposes an ensemble classifier for predicting BC survivability using a new BC post-treatment dataset for the number of survivals. However, the classes survival cases dataset for BC is skewed, which caused a sub-optimal classification performance. Hence, a hybrid sampling scheme of Synthetic Minority Over-Sampling TEchnique (SMOTE) and Wilson's Edited Nearest Neighbor (ENN) is employed to treat the class imbalance in the dataset. Random Forest (RF) ensemble classifier is for classifying the dataset. The proposed framework performs well in terms of accuracy, recall of the two classes, Receiver Operating Characteristics (ROC) and Kappa Statistic (KS) metric on the dataset. The results demonstrated that the RF, with 97.0% accuracy on the holdout sample, is the best predictor. This prediction accuracy is superior to any noted in the literature, compared with Logistic Regression (LR) and Bagging classifiers.
更多查看译文
关键词
Machine learning,Class imbalance,Breast cancer,Sampling,Ensemble learners,Random forest
AI 理解论文
溯源树
样例
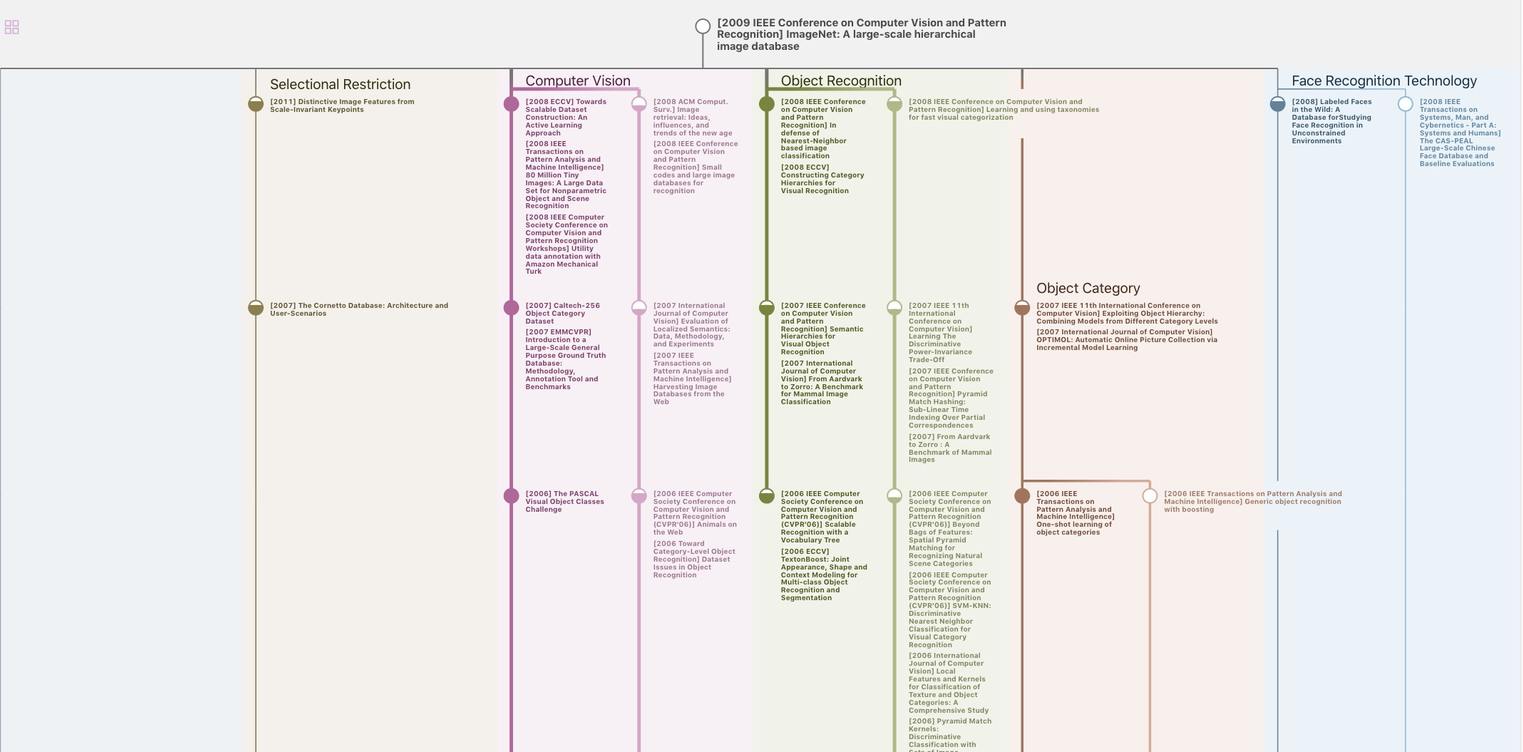
生成溯源树,研究论文发展脉络
Chat Paper
正在生成论文摘要