Application of machine learning for optical emission spectroscopy data in NAGDIS-II
Fusion Engineering and Design(2023)
摘要
In this study, we applied machine learning to optical emission spectroscopy (OES) data and device parameters from the linear plasma device NAGDIS-II to explore the potential application of machine learning for predicting electron density, ne, and temperature, Te. The covered ranges of ne and Te, which were measured by an electrostatic probe, are 3.6 × 1017–2.4×1019 m−3 and 0.3–7.1eV, respectively. A three hidden layer neural network (NN) is introduced to model the relationship between ne/Te and the combination of line intensities, radial position, and device parameters. It is shown that the errors in ne and Te become 18.0 and 18.8%, respectively, which were almost the same level for the electrostatic probe, using all available data. Lasso regression and greedy algorithm are used to select the necessary line emissions. It is shown that four or five line intensities are sufficient to obtain almost the same quality as the one with all the other lines.
更多查看译文
关键词
Optical emission spectroscopy,Machine learning,Diagnostics,Plasma
AI 理解论文
溯源树
样例
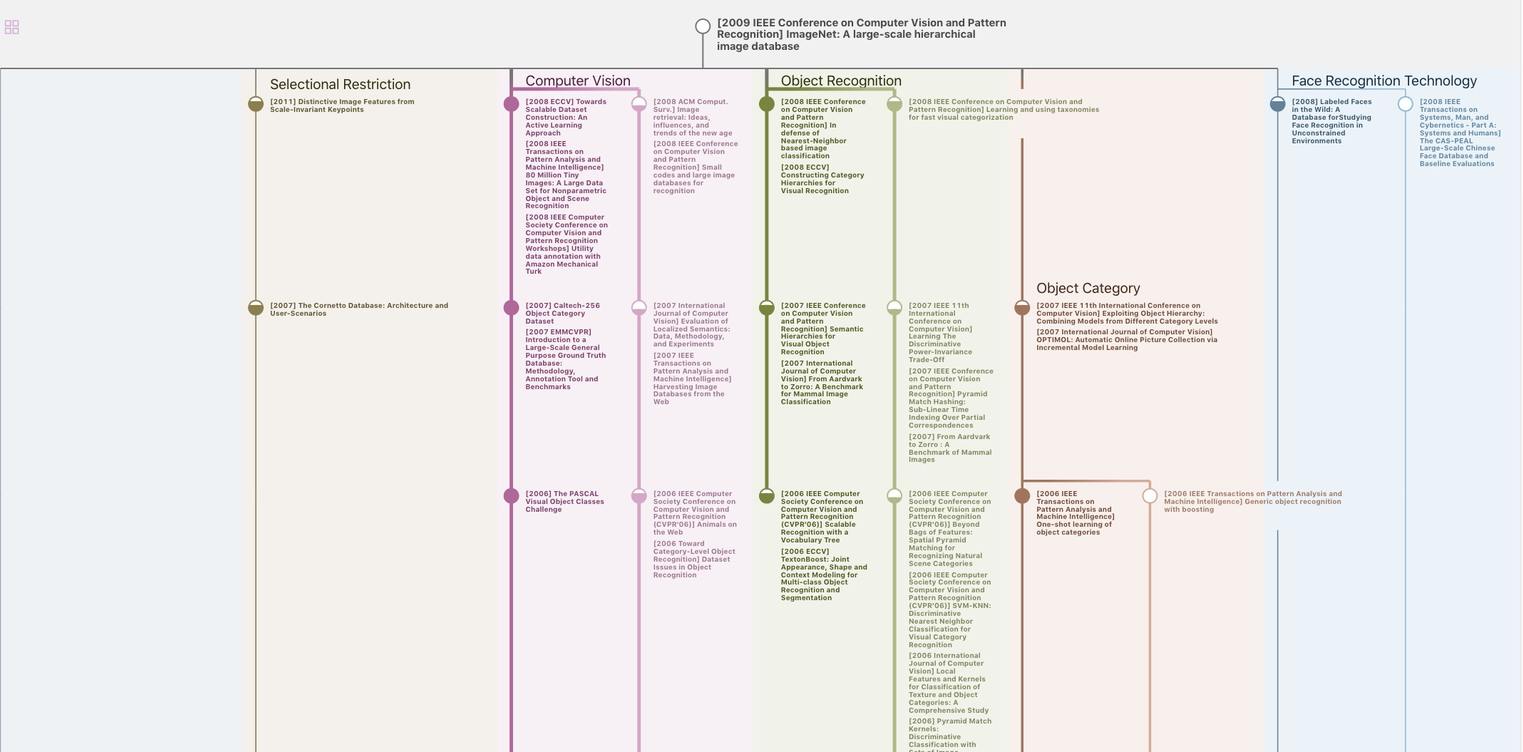
生成溯源树,研究论文发展脉络
Chat Paper
正在生成论文摘要