EGNN: Energy-efficient anomaly detection for IoT multivariate time series data using graph neural network
FUTURE GENERATION COMPUTER SYSTEMS-THE INTERNATIONAL JOURNAL OF ESCIENCE(2024)
摘要
Anomaly detection has been widely applied in Internet of Things (IoT) to guarantee the health of IoT applications. Current studies on anomaly detection focus mainly on measurement design and discovery methods on the cloud, which, however, are associated with issues of computational heaviness and capacity limitation when applied at the network edge. Thus, it becomes important to ensure that the detection is not only accurate but also energy-efficient. To fill this gap, this paper proposes an accurate and Energy-efficient Graph Neural Network based anomaly detection method (EGNN) for IoT multivariate time series data. Specifically, correlations between sensory data upon different IoT devices, which are rarely considered in the literature, are explored through a developed Subgraph Generation Algorithm (SGA) based on graph structure learning. As a result, a dependency graph with multiple subgraphs and their corresponding centres is generated. Thereafter, to reduce anomaly-irrelevant sensory data transmitted in the network, only sensory data upon subgraph centres are utilized for anomaly detection by a computational-light approach, i.e., a multi-layer perceptron based forecasting method. Once an anomaly is detected, sensory data of whole subgraph data are adopted for obtaining accurate anomaly results, by a graph attention based forecasting method. This GNN- based anomaly detection strategy with Mode Switching (GMS) can greatly reduce anomaly-irrelevant data transmission, especially when anomalies occur infrequently. To validate the effectiveness of our mechanism, extensive experiments are conducted upon real-world IoT multivariate time series datasets, and comparison results demonstrate that our technique outperforms the state-of-the-art counterparts in terms of accuracy and energy-efficiency.
更多查看译文
关键词
Multivariate time series,Anomaly detection,Energy-efficient,Graph structure learning,Internet of Things (IoT)
AI 理解论文
溯源树
样例
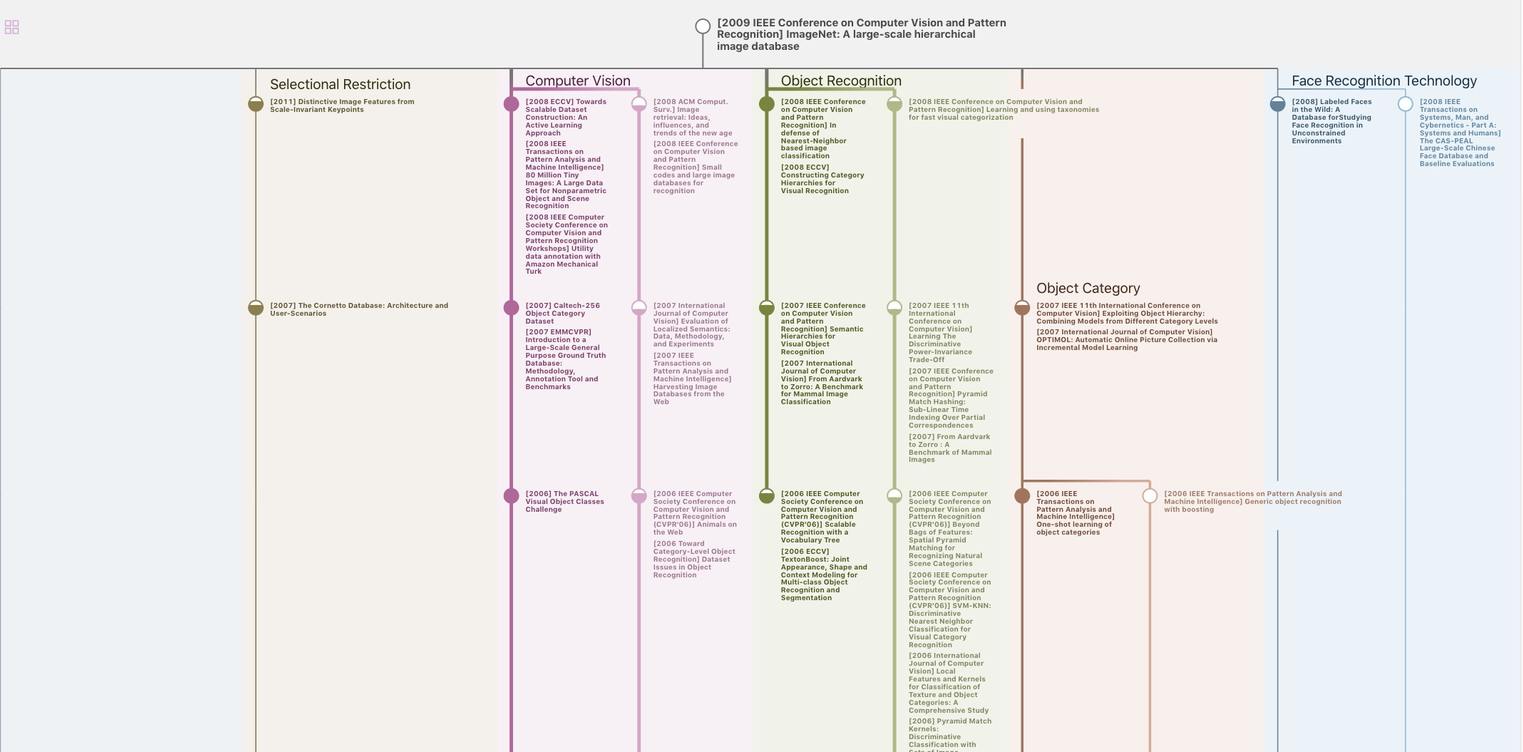
生成溯源树,研究论文发展脉络
Chat Paper
正在生成论文摘要