Machine learning-driven hyperspectral imaging for non-destructive origin verification of green coffee beans across continents, countries, and regions
FOOD CONTROL(2024)
Abstract
Coffee is a target for geographical origin fraud. More rapid, cost-effective, and sustainable traceability solutions are needed. The potential of hyperspectral imaging-near-infrared (HSI-NIR) and advanced machine learning models for rapid and non-destructive origin classification of coffee was explored for the first time (i) to understand the sensitivity of HSI-NIR for classification across various origin scales (continental, country, regional), and (ii) to identify discriminant wavelength regions. HSI-NIR analysis was conducted on green coffee beans from three continents, eight countries, and 22 regions. The classification performance of four different machine learning models (PLS-DA, SVM, RBF-SVM, Random Forest) was compared. Linear SVM provided near-perfect classification performance at the continental, country, and regional levels, and enabled a feature selection opportunity. This study demonstrates the feasibility of using HSI-NIR with machine learning for rapid and nondestructive screening of coffee origin, eliminating the need for sample processing.
MoreTranslated text
Key words
Green coffee bean,Origin traceability,Non-destructive,Hyperspectral,Classification,Machine learning
AI Read Science
Must-Reading Tree
Example
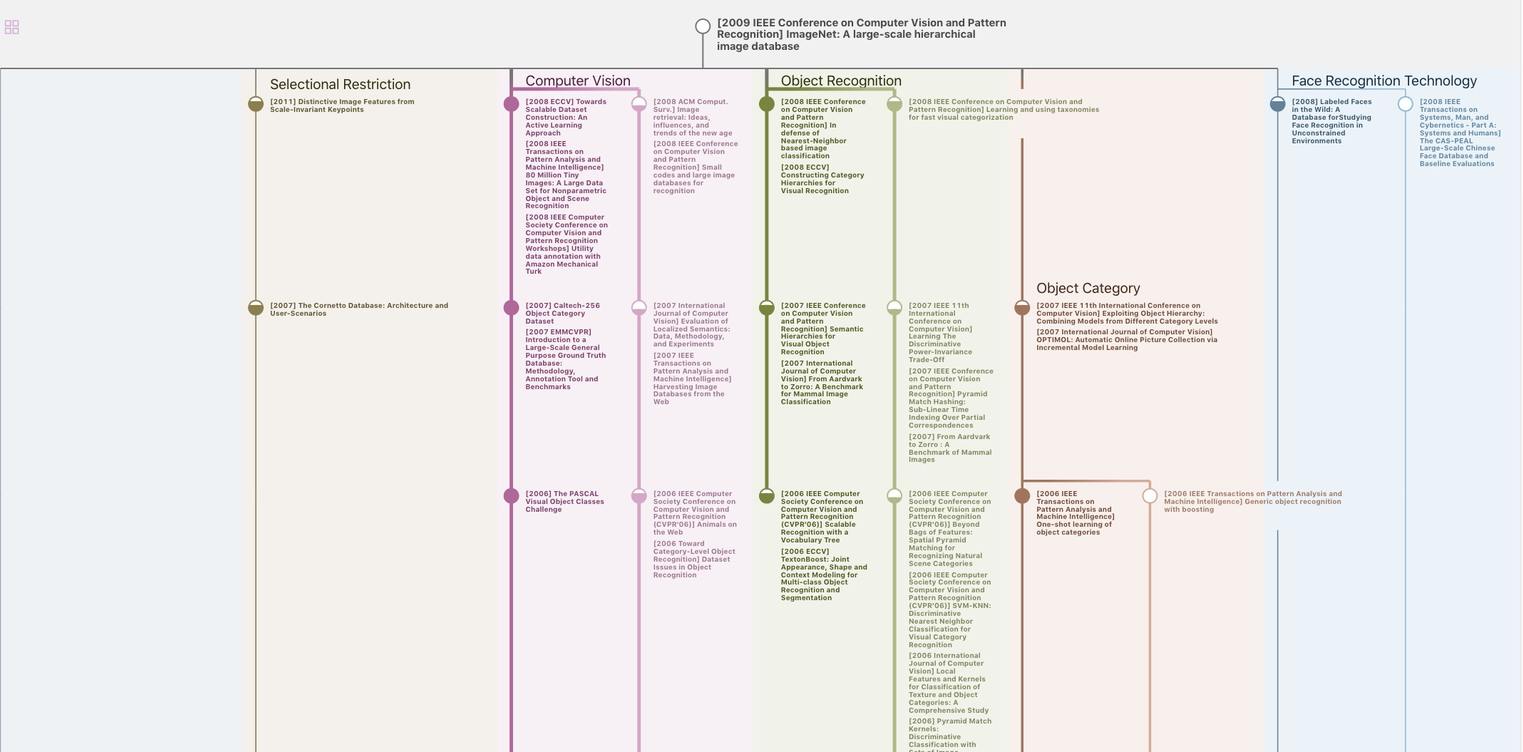
Generate MRT to find the research sequence of this paper
Chat Paper
Summary is being generated by the instructions you defined