An inexact semismooth Newton method with application to adaptive randomized sketching for dynamic optimization
FINITE ELEMENTS IN ANALYSIS AND DESIGN(2024)
Abstract
In many applications, one can only access the inexact gradients and inexact hessian times vector products. Thus it is essential to consider algorithms that can handle such inexact quantities with a guaranteed convergence to solution. An inexact adaptive and provably convergent semismooth Newton method is considered to solve constrained optimization problems. In particular, dynamic optimization problems, which are known to be highly expensive, are the focus. A memory efficient semismooth Newton algorithm is introduced for these problems. The source of efficiency and inexactness is the randomized matrix sketching. Applications to optimization problems constrained by partial differential equations are also considered.
MoreTranslated text
Key words
Nonsmooth optimization,Inexact gradient and Hessian,Semismooth Newton,Adaptivity,Convergence analysis,Compression methods,Randomized sketching,Measure control,Variational discretization
AI Read Science
Must-Reading Tree
Example
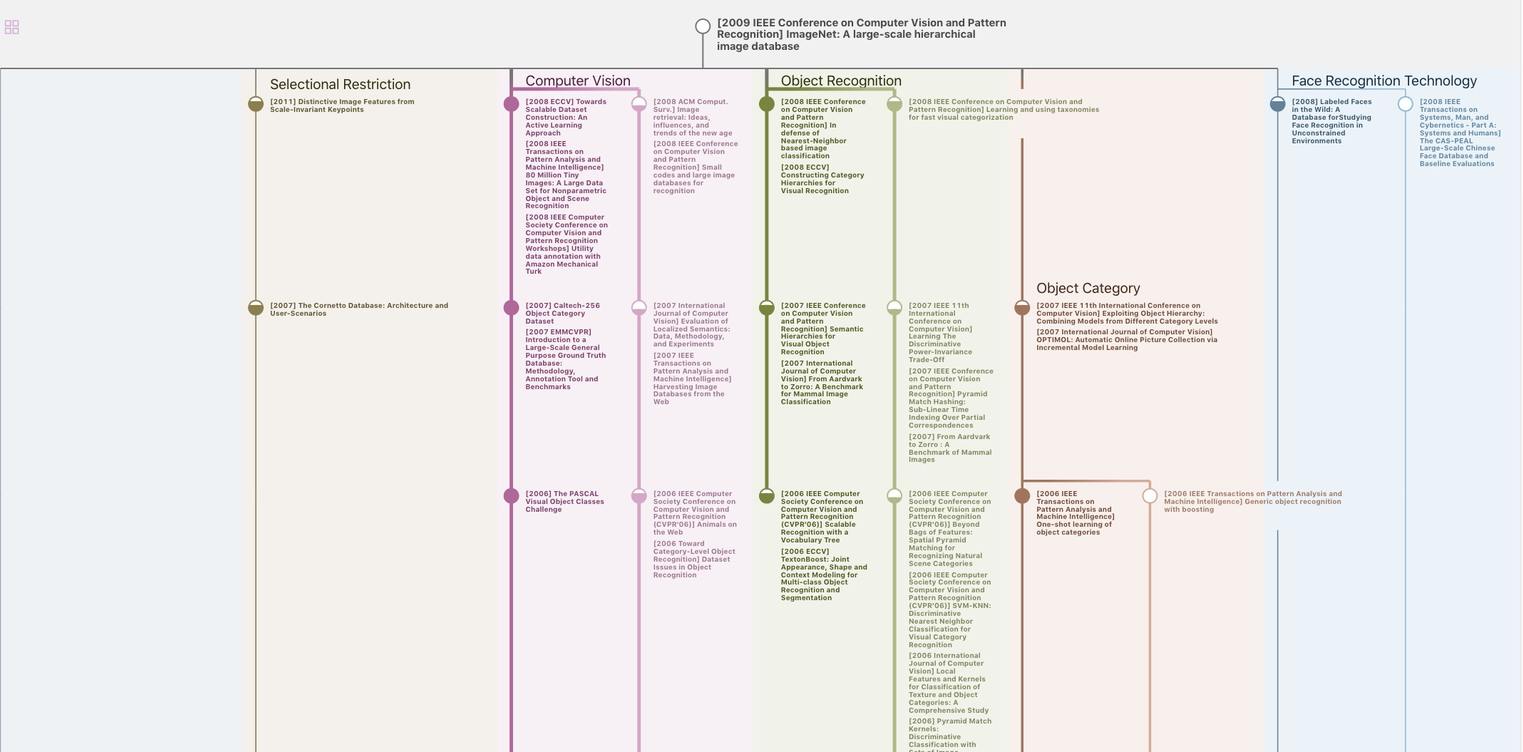
Generate MRT to find the research sequence of this paper
Chat Paper
Summary is being generated by the instructions you defined