Counting in congested crowd scenes with hierarchical scale-aware encoder-decoder network
EXPERT SYSTEMS WITH APPLICATIONS(2024)
摘要
As an indispensable component of intelligent monitoring systems, crowd counting plays a crucial role in many fields, particularly crowd management and control during the COVID-19 pandemic. Despite the promising achievements of many methods, crowd scale variations and noise interference in congested crowd scenes remain urgent problems to be solved. In this paper, we propose a novel Hierarchical Scale-aware Encoder-Decoder Network (HSED-Net) for single-image crowd counting to handle scale variations and noise interference, thereby generating high-quality density maps. The HSED-Net is designed as an encoder-decoder architecture, which contains two core networks: Scale-Aware Encoding network (SAEnet) and Multi-path Aggregation Decoding network (MADnet). The SAEnet focuses on extracting rich multi-scale crowd features, which employs cascaded scale-aware encoding branches to collaboratively obtain high-resolution feature representations. During the encoding phase, two adaptive weight generators are proposed to filter the crowd features from different dimensions to resist the interference of noise. Instead of fusing the multi-scale and multi-level features indiscriminately, the MADnet adopts a multi-path adaptive fusion strategy and selectively emphasizes more appropriate features through the spatial and channel guidance modules, further improving the quality of density maps and the robustness of network. Extensive experiments on four challenging datasets have strongly demonstrated the superiority of our HSED-Net.
更多查看译文
关键词
Crowd counting,Density estimation,Scale-aware network,Encoder-decoder network
AI 理解论文
溯源树
样例
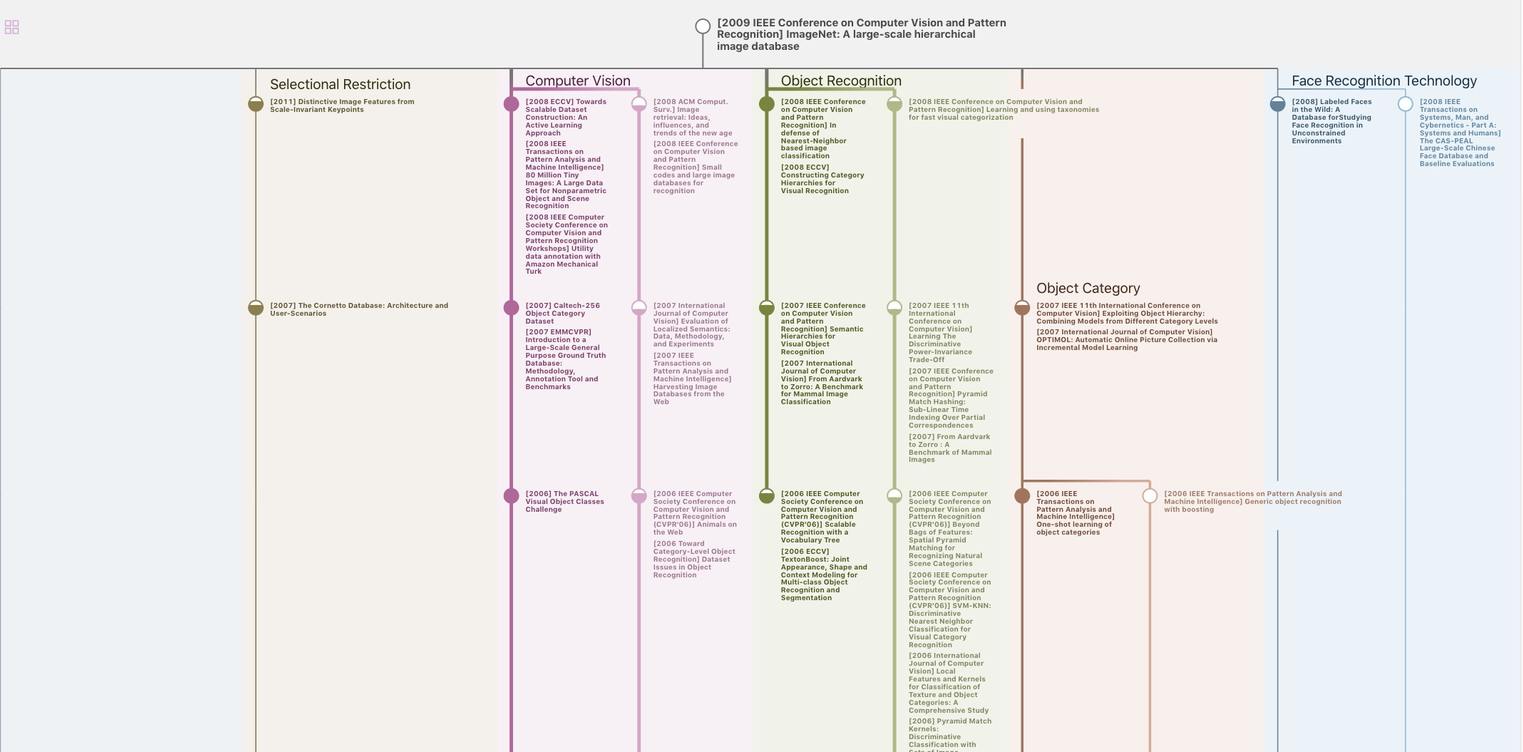
生成溯源树,研究论文发展脉络
Chat Paper
正在生成论文摘要