You are your friends: Detecting malware via guilt-by-association and exempt-by-reputation
COMPUTERS & SECURITY(2024)
摘要
With the increase in the prevalence of Security Information and Event Management Systems (SIEMs) in today's organizations, there is a growing interest in data-driven threat detection. In this research, we formulate malware detection as a large-scale graph mining and inference problem using host-level system events/logs. Our approach is built on two basic principles: guilt-by-association and exempt-by-reputation, with the intuition, that an adversary's resources are limited; hence, reusing infrastructures and techniques is inevitable. We present MalLink, a system that models all host-level process activities as a Heterogeneous Information Network (HIN). The HIN emphasizes shared characteristics of processes/files across the enterprise, e.g., parent/sub-processes, written/read files, loaded libraries, registry entries, and network connections. MalLink then propagates maliciousness from a set of previously known malicious entities to obtain a set of previously unknowns. MalLink was deployed in a real-world setting, next to the SIEM system of a large international enterprise, and evaluated using 8 days (20 TB) of EDR logs collected from all endpoints within the organization. The results demonstrate high detection performance (F1-score of 0.83), particularly when manually investigating the 50 highest scored files with no prior, 37 are found malicious. This demonstrates MalLink's capability to detect previously unknown malicious files.
更多查看译文
关键词
Malware detection,Graph mining,Graph inference,Guilt-by-association,SIEM,EDR,Provenance graph,Cybersecurity knowledge graph
AI 理解论文
溯源树
样例
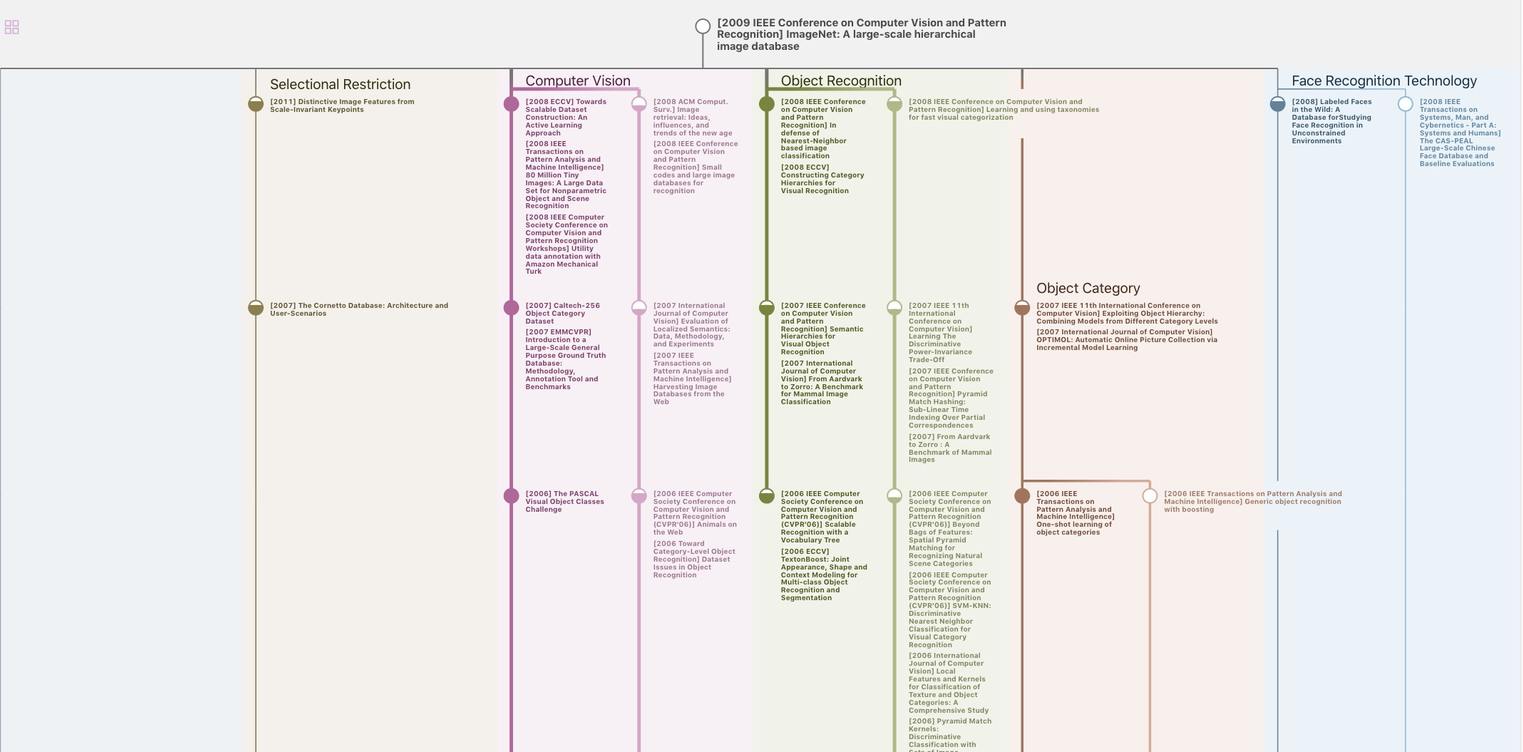
生成溯源树,研究论文发展脉络
Chat Paper
正在生成论文摘要