Reinforcement learning strategy for control of microstructure evolution in phase field models
COMPUTATIONAL MATERIALS SCIENCE(2024)
摘要
We propose a reinforcement learning-based approach for controlling microstructure evolution in phase field models. A key feature of our approach is the replacement of the high-fidelity but computationally expensive phase field simulation with an inexpensive surrogate model of acceptable fidelity. We apply our approach to a 2D model problem based on the Allen-Cahn equation that represents a two-phase microstructure with a single, scalar order parameter. The control parameters for this model are spatially and temporally varying temperature (T) and bias fields (h). Our approach generates sets of T and h parameters that yield final microstructures qualitatively matching target microstructures. We discuss further developments required to apply this approach to optimal processing of designer microstructures.
更多查看译文
关键词
Reinforcement learning,Phase field simulation,Microstructure control
AI 理解论文
溯源树
样例
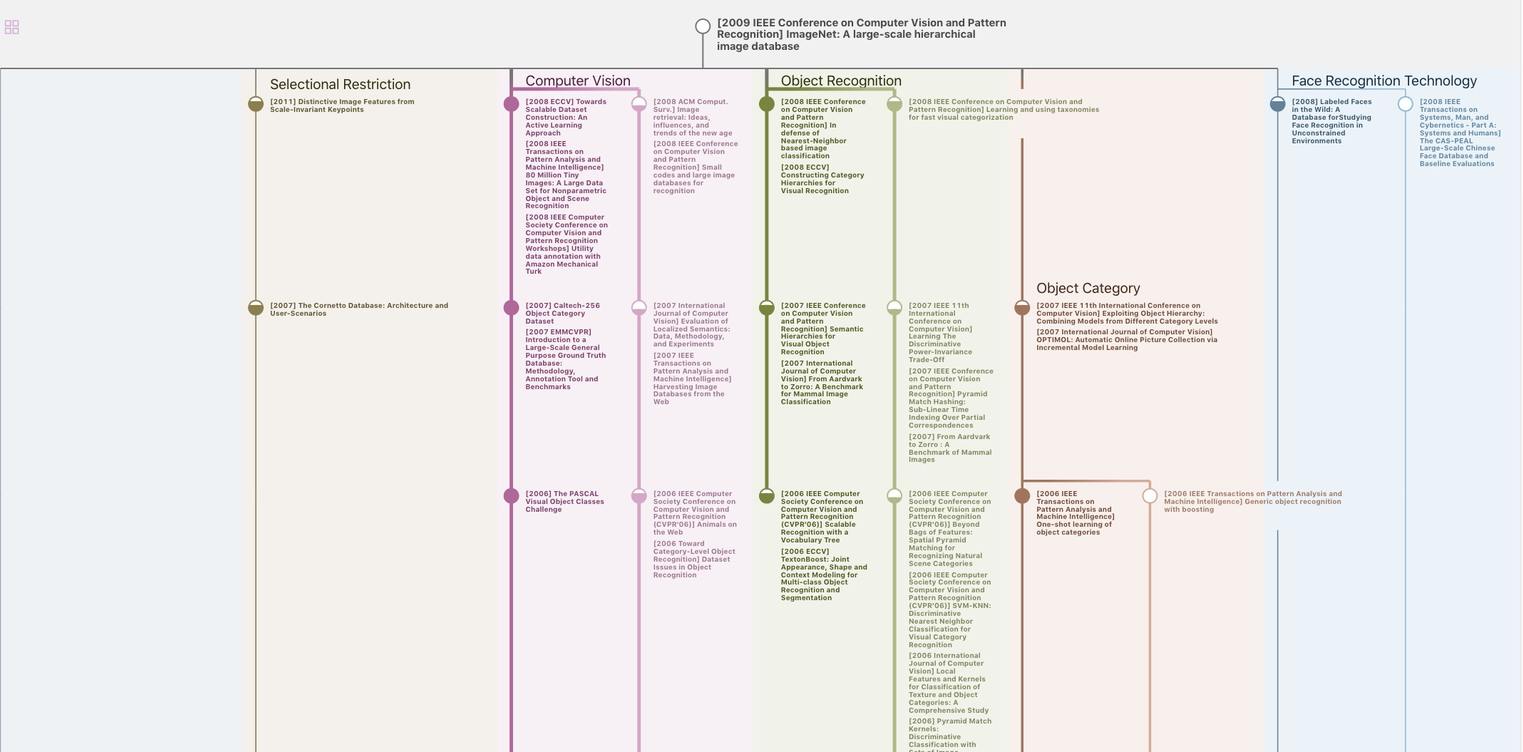
生成溯源树,研究论文发展脉络
Chat Paper
正在生成论文摘要