Deep-learning image enhancement and fibre segmentation from time-resolved computed tomography of fibre-reinforced composites
Composites Science and Technology(2023)
摘要
Monitoring the microstructure and damage development of fibre-reinforced composites during loading is crucial to understanding their mechanical properties. Time-resolved X-ray computed tomography enables such an in -situ, non-destructive study. However, the photon flux and fibre-matrix contrast limit its achievable spatial and temporal resolution. In this paper, we push the limits of temporal and spatial resolution for the microstructural analysis of unidirectional continuous carbon fibre-reinforced epoxy composites by establishing a new pipeline based on CycleGAN for unsupervised super-resolution and denoising and U-Net-id for individual fibre segmen-tation. After illustrating the benefits of a 3D CycleGAN over a 2D one, we show that data enhanced by this pipeline can yield similar segmentation quality to that of a slow-acquisition, high-quality scan that took up to 200 times longer to acquire. This pipeline, therefore, enables more robust data extraction from fast time-resolved X-ray tomography, removing a critical stumbling block for this technique.
更多查看译文
关键词
A. Polymer -matrix composites,A. Carbon fibre,D. X-ray computed tomography,D. Non-destructive testing,Deep learning
AI 理解论文
溯源树
样例
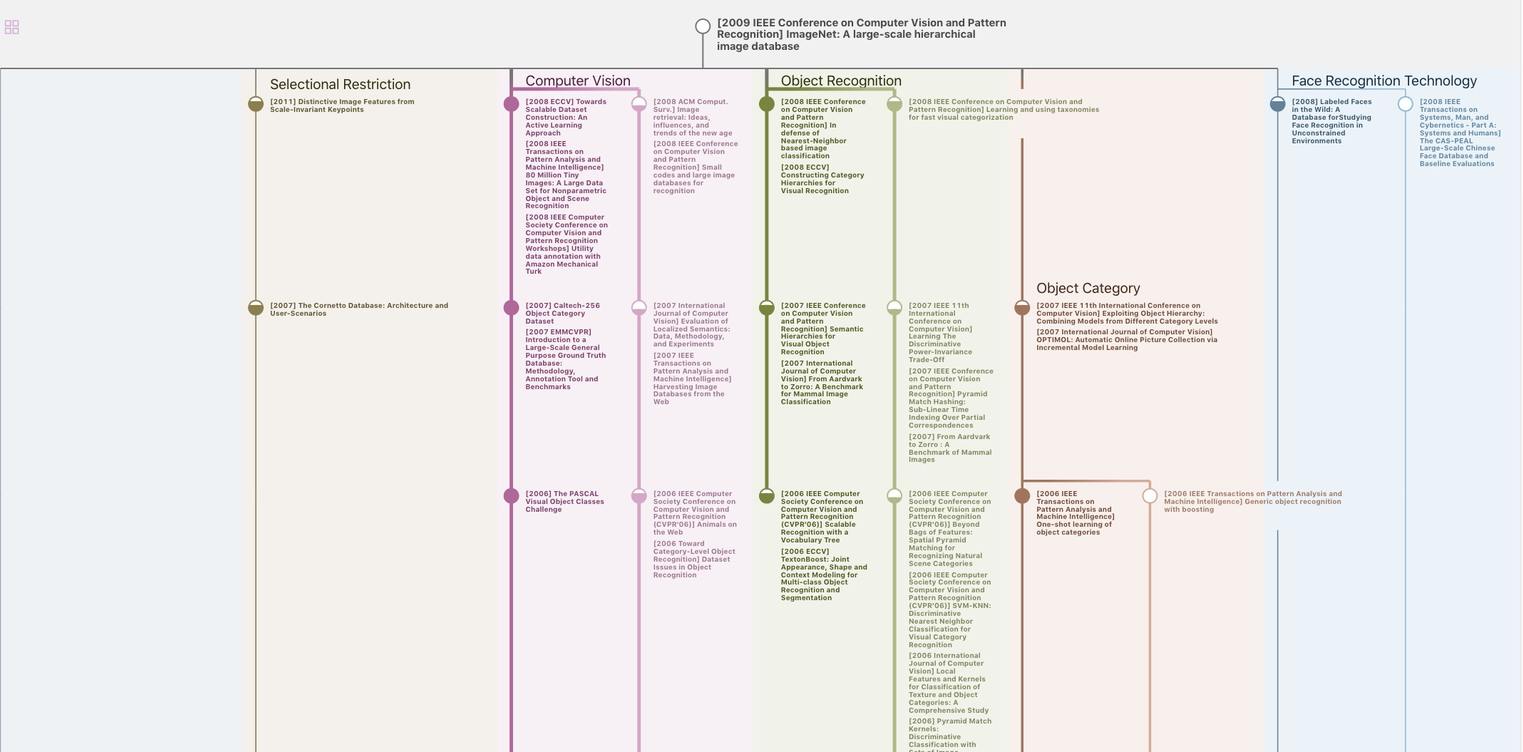
生成溯源树,研究论文发展脉络
Chat Paper
正在生成论文摘要