A clustering approach to improve our understanding of the genetic and phenotypic complexity of chronic kidney disease
medrxiv(2023)
Abstract
Chronic kidney disease (CKD) is a complex disorder that causes a gradual loss of kidney function, affecting approximately 9.1% of the world’s population. Here, we use a soft-clustering algorithm to deconstruct its genetic heterogeneity. First, we selected 322 CKD-associated independent genetic variants from published genome-wide association studies (GWAS) and added association results for 229 traits from the GWAS catalog. We then applied nonnegative matrix factorization (NMF) to discover overlapping clusters of related traits and variants. We computed cluster-specific polygenic scores and validated each cluster with a phenome-wide association study (PheWAS) on the BioMe biobank (n=31,701). NMF identified nine clusters that reflect different aspects of CKD, with the top-weighted traits signifying areas such as kidney function, type 2 diabetes (T2D), and body weight. For most clusters, the top-weighted traits were confirmed in the PheWAS analysis. Results were found to be more significant in the cross-ancestry analysis, although significant ancestry-specific associations were also identified. While all alleles were associated with a decreased kidney function, associations with CKD-related diseases (e.g., T2D) were found only for a smaller subset of variants and differed across genetic ancestry groups. Our findings leverage genetics to gain insights into the underlying biology of CKD and investigate population-specific associations.
### Competing Interest Statement
Claudia Schurmann is a paid employee of Bayer AG, Pharmaceuticals. All other authors do not have any competing interest.
### Funding Statement
This work was supported in part through the computational and data resources and staff expertise provided by Scientific Computing and Data at the Icahn School of Medicine at Mount Sinai and supported by the Clinical and Translational Science Awards (CTSA) grant UL1TR004419 from the National Center for Advancing Translational Sciences. Additionally, this work was supported by the Office of Research Infrastructure of the National Institutes of Health under award number S10OD026880, which allowed us to use Mount Sinai Data Warehouse (MSDW) data. Regarding HPI.MS resources, funding was provided by the Hasso Plattner Foundation (HPF). Additionally, the research leading to these results has received funding from the Horizon 2020 Programme of the European Commission under Grant Agreement No. 826117 (Smart4Health). The Mount Sinai BioMe Biobank has been supported by The Andrea and Charles Bronfman Philanthropies and in part by Federal funds from the NHLBI and NHGRI (U01HG00638001; U01HG007417; X01HL134588).
### Author Declarations
I confirm all relevant ethical guidelines have been followed, and any necessary IRB and/or ethics committee approvals have been obtained.
Yes
The details of the IRB/oversight body that provided approval or exemption for the research described are given below:
The IRB of the Mount Sinai Health System (NYC, NY, USA) gave ethical approval for this work.
I confirm that all necessary patient/participant consent has been obtained and the appropriate institutional forms have been archived, and that any patient/participant/sample identifiers included were not known to anyone (e.g., hospital staff, patients or participants themselves) outside the research group so cannot be used to identify individuals.
Yes
I understand that all clinical trials and any other prospective interventional studies must be registered with an ICMJE-approved registry, such as ClinicalTrials.gov. I confirm that any such study reported in the manuscript has been registered and the trial registration ID is provided (note: if posting a prospective study registered retrospectively, please provide a statement in the trial ID field explaining why the study was not registered in advance).
Yes
I have followed all appropriate research reporting guidelines, such as any relevant EQUATOR Network research reporting checklist(s) and other pertinent material, if applicable.
Yes
All publicly available data (input variants, trait-variant associations) used to support the findings of this study are included in this published article (and its Supplementary Information files) and are also available from the cited publications and GWAS Catalog. Additional data generated for the analysis steps, including source code and intermediate results, are available from the corresponding author upon reasonable request. The data used to validate the findings of this study are available from BioMe biobank (), but restrictions apply to their availability. To access the data, please reach out to biomebiobank{at}mssm.edu.
MoreTranslated text
AI Read Science
Must-Reading Tree
Example
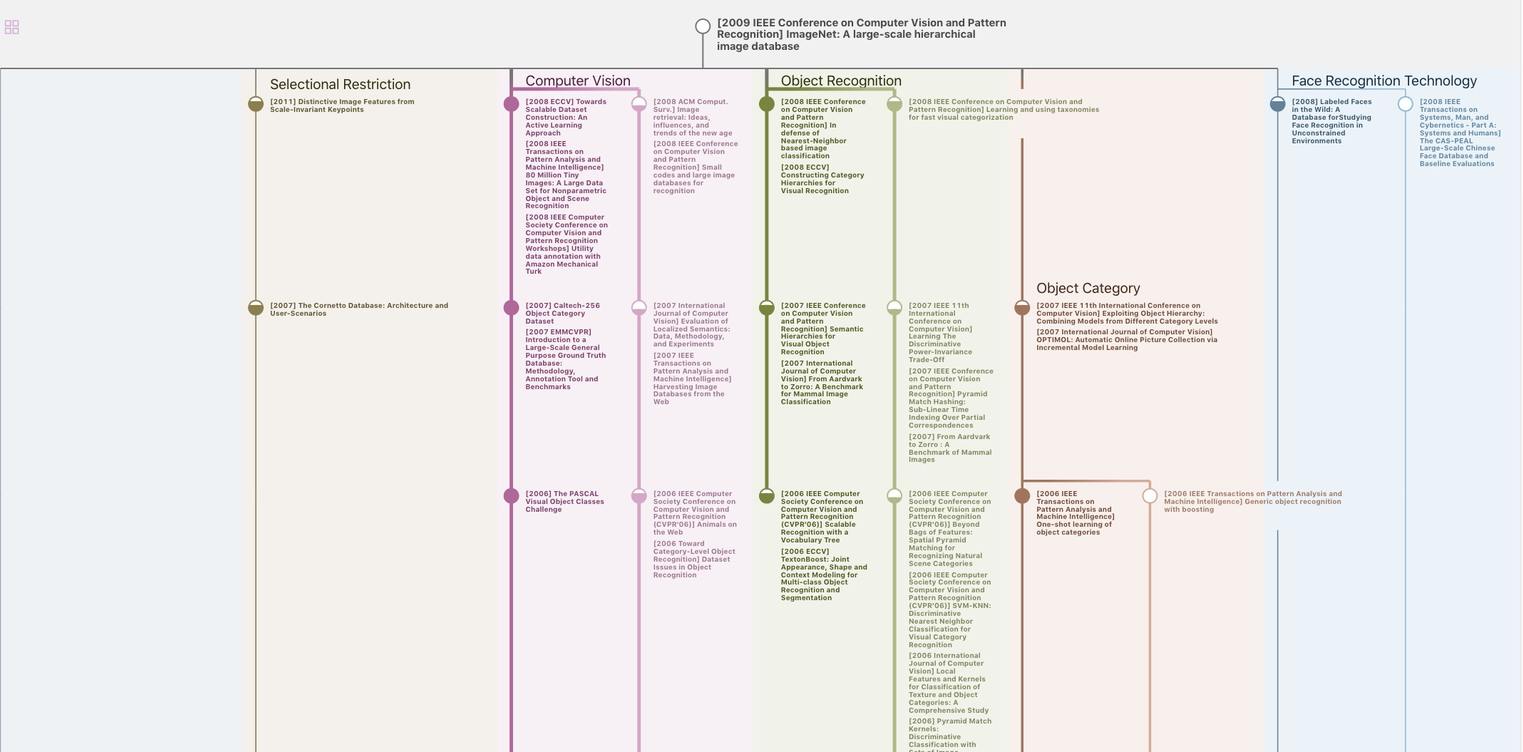
Generate MRT to find the research sequence of this paper
Chat Paper
Summary is being generated by the instructions you defined