Transformer-based Entity Legal Form Classification
CoRR(2023)
摘要
We propose the application of Transformer-based language models for classifying entity legal forms from raw legal entity names. Specifically, we employ various BERT variants and compare their performance against multiple traditional baselines. Our evaluation encompasses a substantial subset of freely available Legal Entity Identifier (LEI) data, comprising over 1.1 million legal entities from 30 different legal jurisdictions. The ground truth labels for classification per jurisdiction are taken from the Entity Legal Form (ELF) code standard (ISO 20275). Our findings demonstrate that pre-trained BERT variants outperform traditional text classification approaches in terms of F1 score, while also performing comparably well in the Macro F1 Score. Moreover, the validity of our proposal is supported by the outcome of third-party expert reviews conducted in ten selected jurisdictions. This study highlights the significant potential of Transformer-based models in advancing data standardization and data integration. The presented approaches can greatly benefit financial institutions, corporations, governments and other organizations in assessing business relationships, understanding risk exposure, and promoting effective governance.
更多查看译文
关键词
classification,transformer-based,legal,transformer-based
AI 理解论文
溯源树
样例
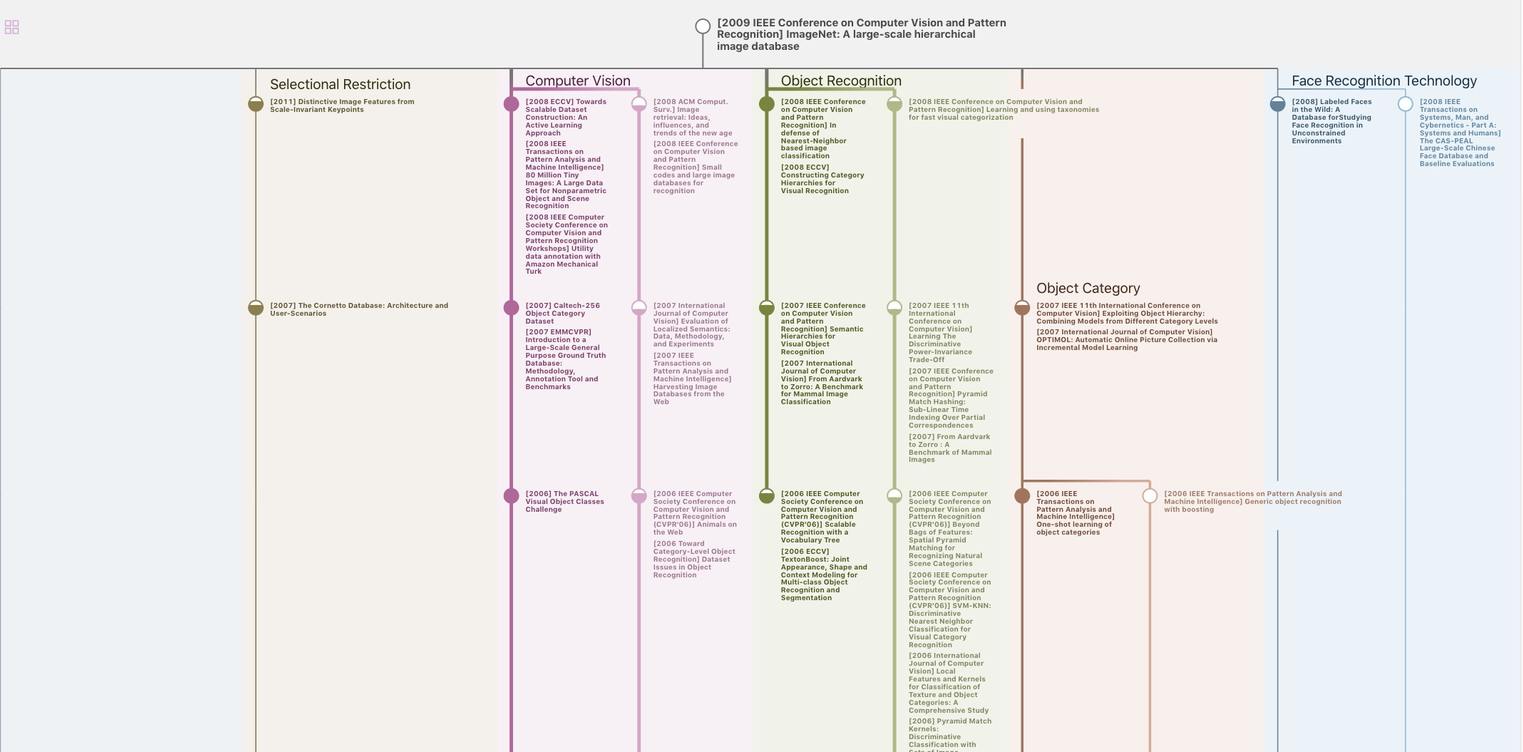
生成溯源树,研究论文发展脉络
Chat Paper
正在生成论文摘要