Product of Gaussian Mixture Diffusion Models
Journal of Mathematical Imaging and Vision(2024)
摘要
In this work, we tackle the problem of estimating the density f_X of a random variable X by successive smoothing, such that the smoothed random variable Y fulfills the diffusion partial differential equation (∂ _t - Δ _1)f_Y( · , t) = 0 with initial condition f_Y( · , 0) = f_X . We propose a product-of-experts-type model utilizing Gaussian mixture experts and study configurations that admit an analytic expression for f_Y ( · , t) . In particular, with a focus on image processing, we derive conditions for models acting on filter, wavelet, and shearlet responses. Our construction naturally allows the model to be trained simultaneously over the entire diffusion horizon using empirical Bayes. We show numerical results for image denoising where our models are competitive while being tractable, interpretable, and having only a small number of learnable parameters. As a by-product, our models can be used for reliable noise level estimation, allowing blind denoising of images corrupted by heteroscedastic noise.
更多查看译文
关键词
Diffusion models,Empirical bayes,Gaussian mixture,Blind denoising
AI 理解论文
溯源树
样例
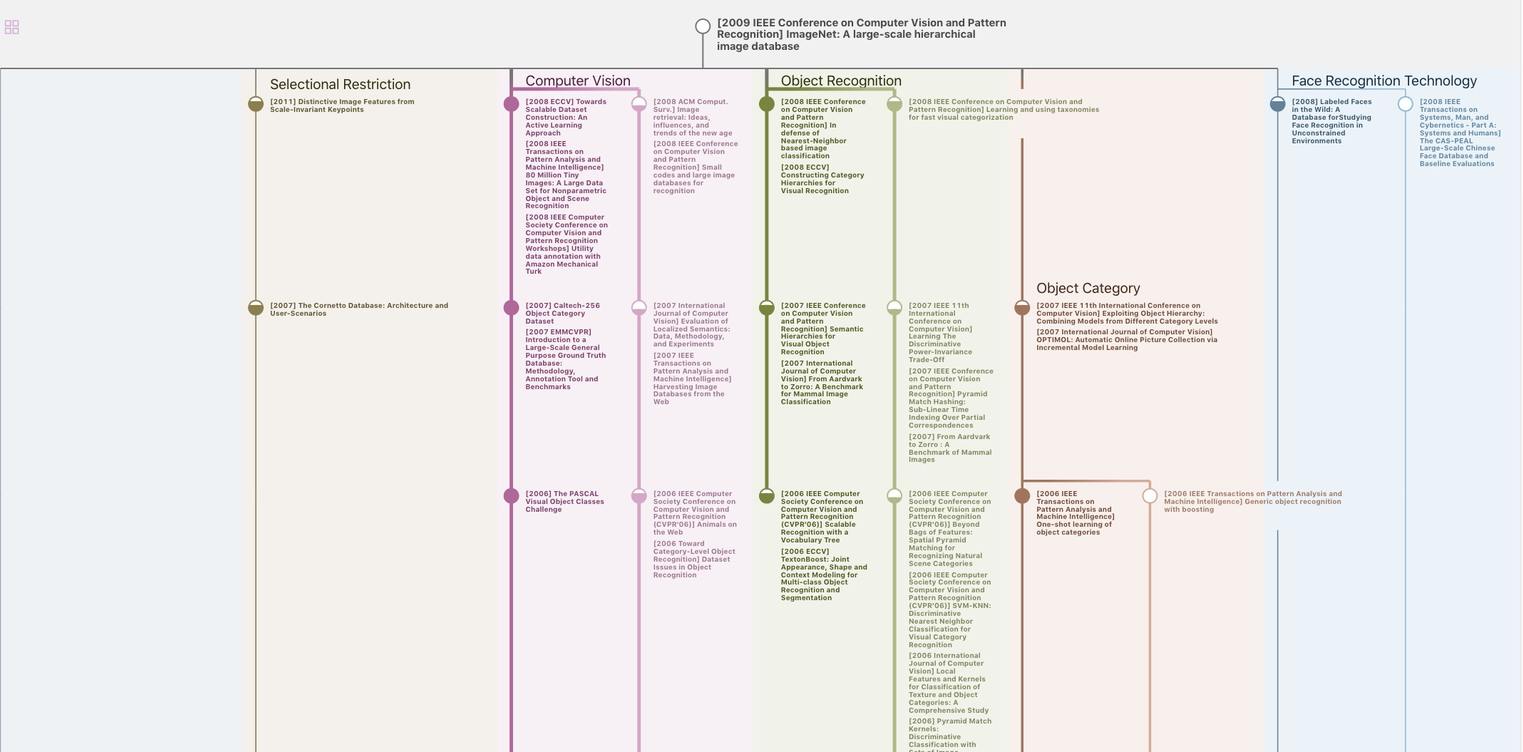
生成溯源树,研究论文发展脉络
Chat Paper
正在生成论文摘要