Julearn: an easy-to-use library for leakage-free evaluation and inspection of ML models.
GigaByte (Hong Kong, China)(2024)
Abstract
The fast-paced development of machine learning (ML) and its increasing adoption in research challenge researchers without extensive training in ML. In neuroscience, ML can help understand brain-behavior relationships, diagnose diseases and develop biomarkers using data from sources like magnetic resonance imaging and electroencephalography. Primarily, ML builds models to make accurate predictions on unseen data. Researchers evaluate models' performance and generalizability using techniques such as cross-validation (CV). However, choosing a CV scheme and evaluating an ML pipeline is challenging and, if done improperly, can lead to overestimated results and incorrect interpretations. Here, we created julearn, an open-source Python library allowing researchers to design and evaluate complex ML pipelines without encountering common pitfalls. We present the rationale behind julearn's design, its core features, and showcase three examples of previously-published research projects. Julearn simplifies the access to ML providing an easy-to-use environment. With its design, unique features, simple interface, and practical documentation, it poses as a useful Python-based library for research projects.
MoreTranslated text
Key words
models,inspection,evaluation,easy-to-use,leakage-free
AI Read Science
Must-Reading Tree
Example
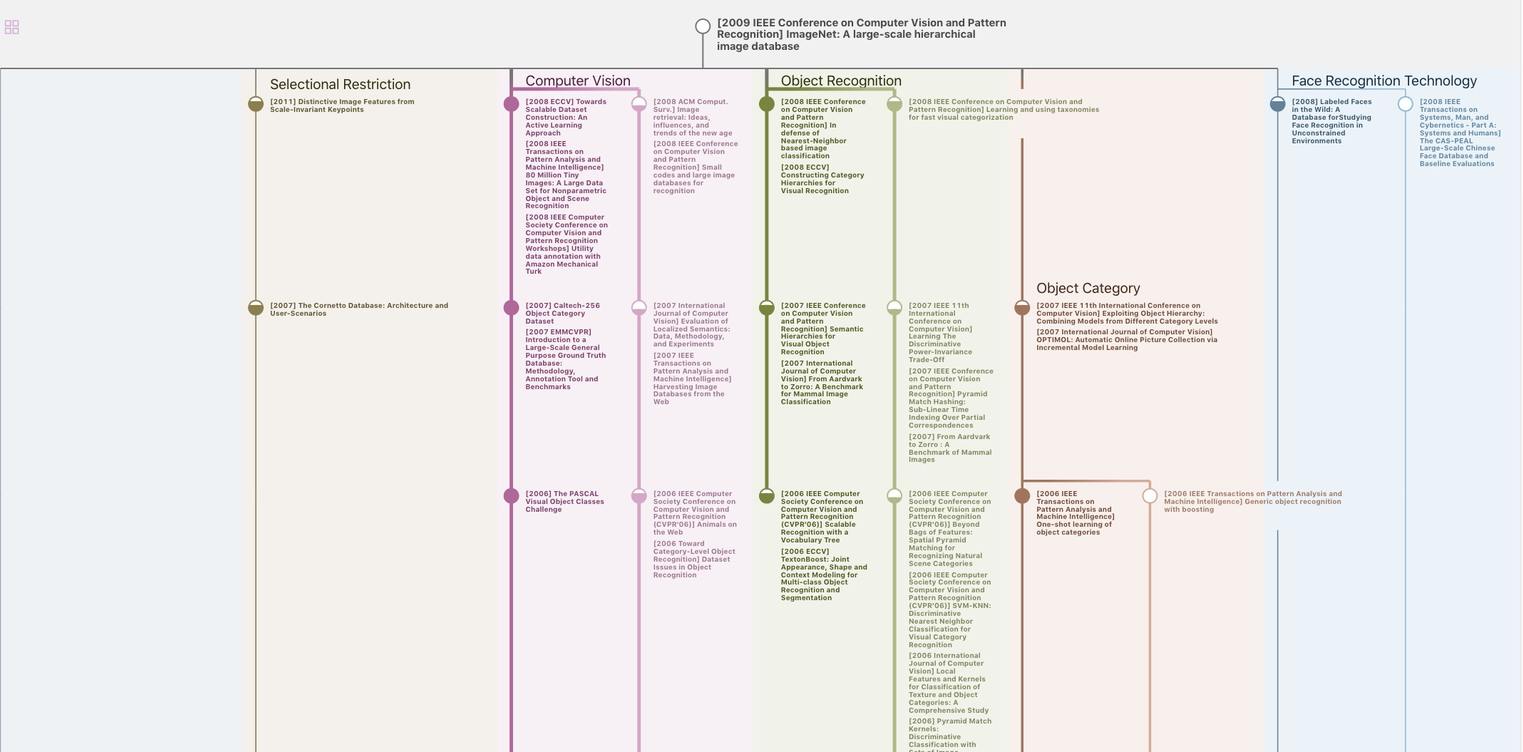
Generate MRT to find the research sequence of this paper
Chat Paper
Summary is being generated by the instructions you defined