Data-assisted, physics-informed propagators for recurrent flows
PHYSICAL REVIEW FLUIDS(2024)
摘要
An alternative approach to simulate dynamic, recurrent flows with very large time steps is presented. Data -driven forecasts based on the method of analogs are made employing a set of precomputed time series segments of flow fields. These predictions are then corrected with the evolved deviation between initial flow state and its nearest neighbor in the database. The evolution of these deviations is obtained from the convolution with the propagator of the linearized Navier-Stokes equations, where linearization takes place around the most similar state in the database. The error Elin due to this approximation implies the appropriate distance function to determine nearest -neighbor fields. We tested the method on the simple case of 2D vortex shedding behind a cylinder at Reynolds numbers in the range of 200 with step sizes of up to 200 times larger than those used to generate the underlying data with the PISO algorithm. Short-term forecasts showed a high degree of accuracy with errors correlating well with Elin for a number of case variations. But also after many steps, the predicted flow fields remained in close agreement with high-fidelity CFD calculations with relative errors on the order of 1% for the temporal averages. Furthermore, excellent conservation behavior of global properties like total momentum and kinetic energy was observed.
更多查看译文
关键词
propagators,flows,data-assisted,physics-informed
AI 理解论文
溯源树
样例
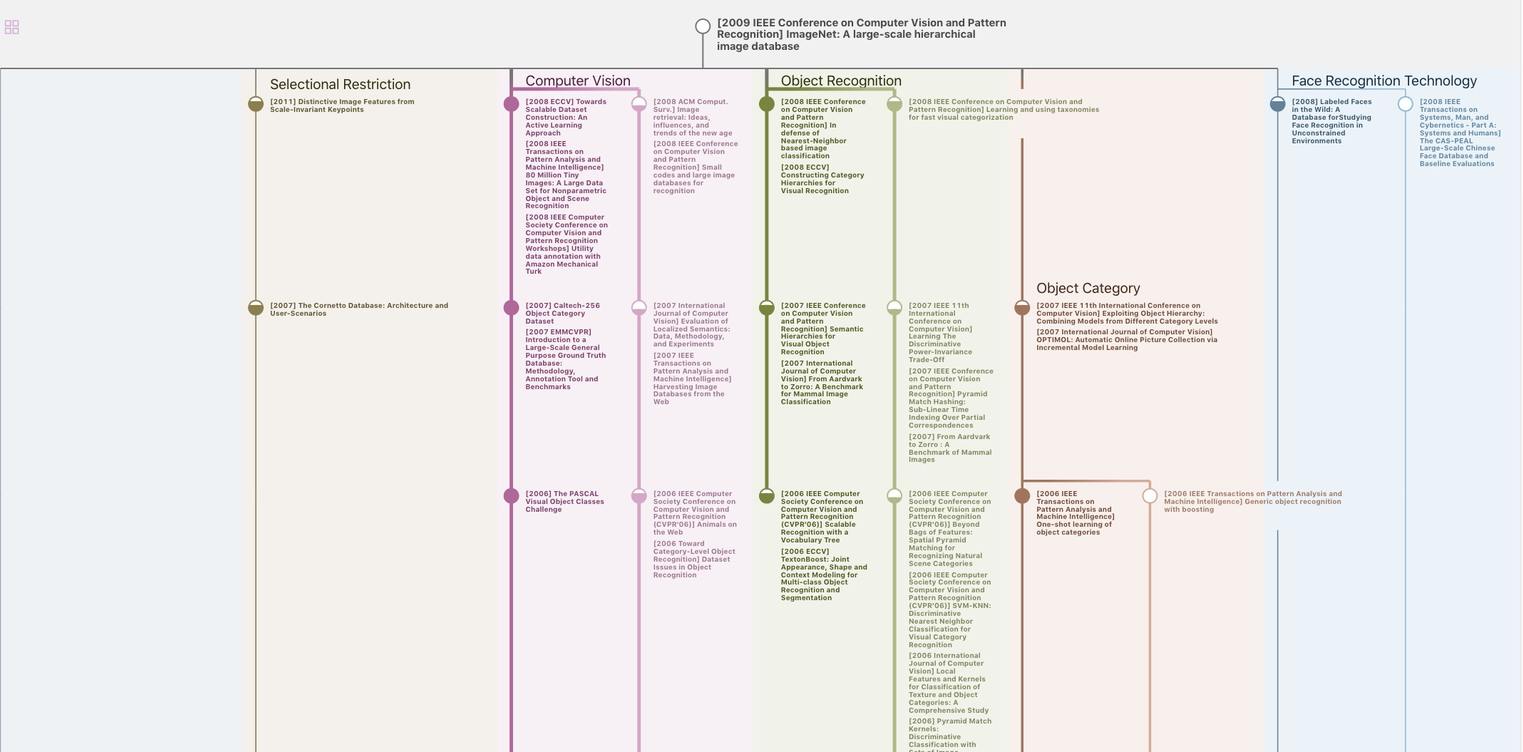
生成溯源树,研究论文发展脉络
Chat Paper
正在生成论文摘要