Reinforcement learning and working memory in mood disorders: A computational analysis in a developmental transdiagnostic sample
JOURNAL OF AFFECTIVE DISORDERS(2024)
Abstract
Background: Mood disorders commonly onset during adolescence and young adulthood and are conceptually and empirically related to reinforcement learning abnormalities. However, the nature of abnormalities associated with acute symptom severity versus lifetime diagnosis remains unclear, and prior research has often failed to disentangle working memory from reward processes. Methods: The present sample (N = 220) included adolescents and young adults with a lifetime history of unipolar disorders (n = 127), bipolar disorders (n = 28), or no history of psychopathology (n = 62), and varying severity of mood symptoms. Analyses fitted a reinforcement learning and working memory model to an instrumental learning task that varied working memory load, and tested associations between model parameters and diagnoses or current symptoms.Results: Current severity of manic or anhedonic symptoms negatively correlated with task performance. Participants reporting higher severity of current anhedonia, or with lifetime unipolar or bipolar disorders, showed lower reward learning rates. Participants reporting higher severity of current manic symptoms showed faster working memory decay and reduced use of working memory. Limitations: Computational parameters should be interpreted in the task environment (a deterministic reward learning paradigm), and developmental population. Future work should test replication in other paradigms and populations.Conclusions: Results indicate abnormalities in reinforcement learning processes that either scale with current symptom severity, or correspond with lifetime mood diagnoses. Findings may have implications for understanding reward processing anomalies related to state-like (current symptom) or trait-like (lifetime diagnosis) aspects of mood disorders.
MoreTranslated text
Key words
Reward learning,Executive functions,Mood disorders,Computational modeling
AI Read Science
Must-Reading Tree
Example
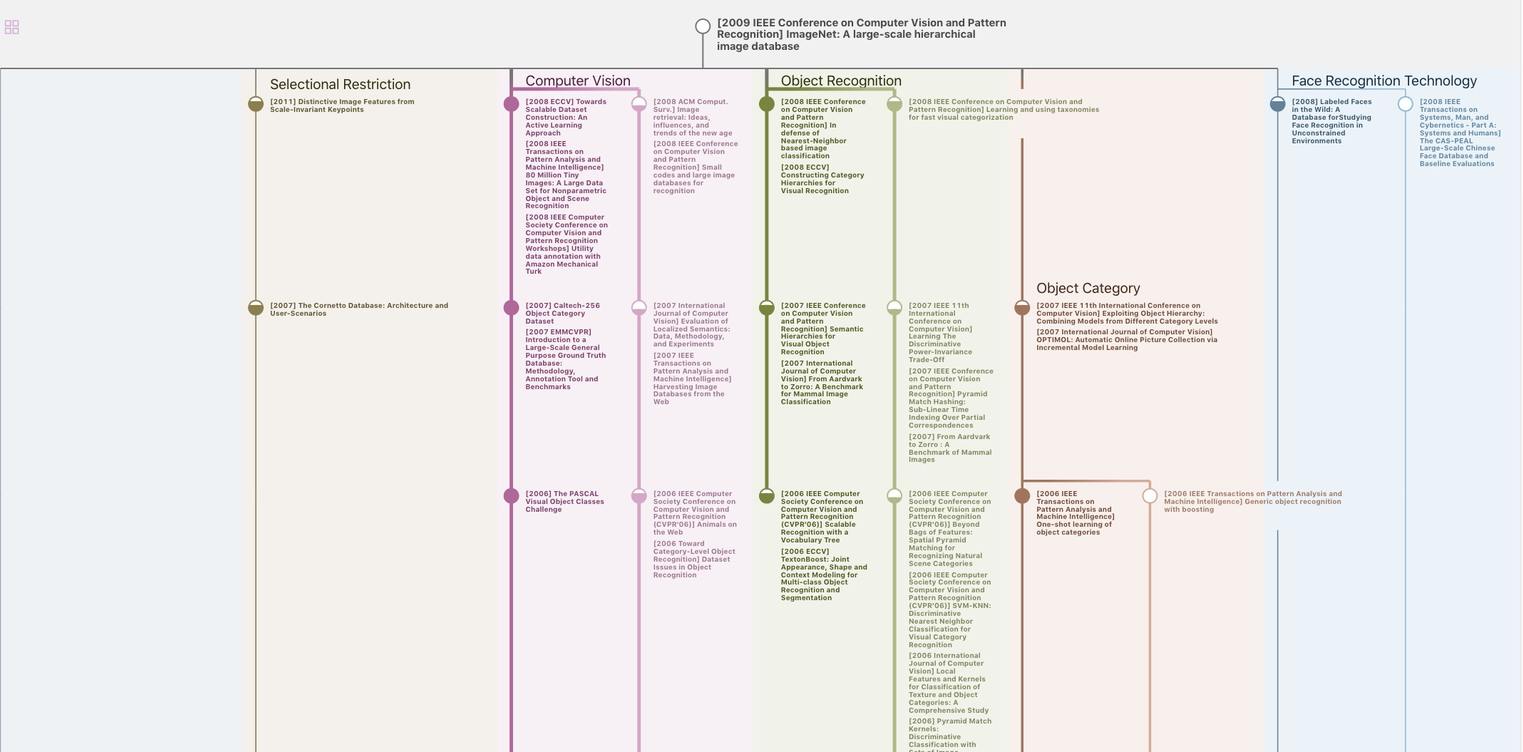
Generate MRT to find the research sequence of this paper
Chat Paper
Summary is being generated by the instructions you defined